The complementary roles of auditory and motor information evaluated in a Bayesian perceptuo-motor model of speech perception.
PSYCHOLOGICAL REVIEW(2017)
摘要
There is a consensus concerning the view that both auditory and motor representations intervene in the perceptual processing of speech units. However, the question of the functional role of each of these systems remains seldom addressed and poorly understood. We capitalized on the formal framework of Bayesian Programming to develop COSMO (Communicating Objects using SensoryMotor Operations), an integrative model that allows principled comparisons of purely motor or purely auditory implementations of a speech perception task and tests the gain of efficiency provided by their Bayesian fusion. Here, we show 3 main results: (a) In a set of precisely defined "perfect conditions,"auditory and motor theories of speech perception are indistinguishable; (b) When a learning process that mimics speech development is introduced into COSMO, it departs from these perfect conditions. Then auditory recognition becomes more efficient than motor recognition in dealing with learned stimuli, while motor recognition is more efficient in adverse conditions. We interpret this result as a general "auditory-narrowband versus motor-wideband" property; and (c) Simulations of plosive-vowel syllable recognition reveal possible cues from motor recognition for the invariant specification of the place of plosive articulation in context that are lacking in the auditory pathway. This provides COSMO with a second property, where auditory cues would be more efficient for vowel decoding and motor cues for plosive articulation decoding. These simulations provide several predictions, which are in good agreement with experimental data and suggest that there is natural complementarity between auditory and motor processing within a perceptuomotor theory of speech perception.
更多查看译文
关键词
speech perception,computational modeling,sensory-motor interactions,adverse conditions,plosive invariance
AI 理解论文
溯源树
样例
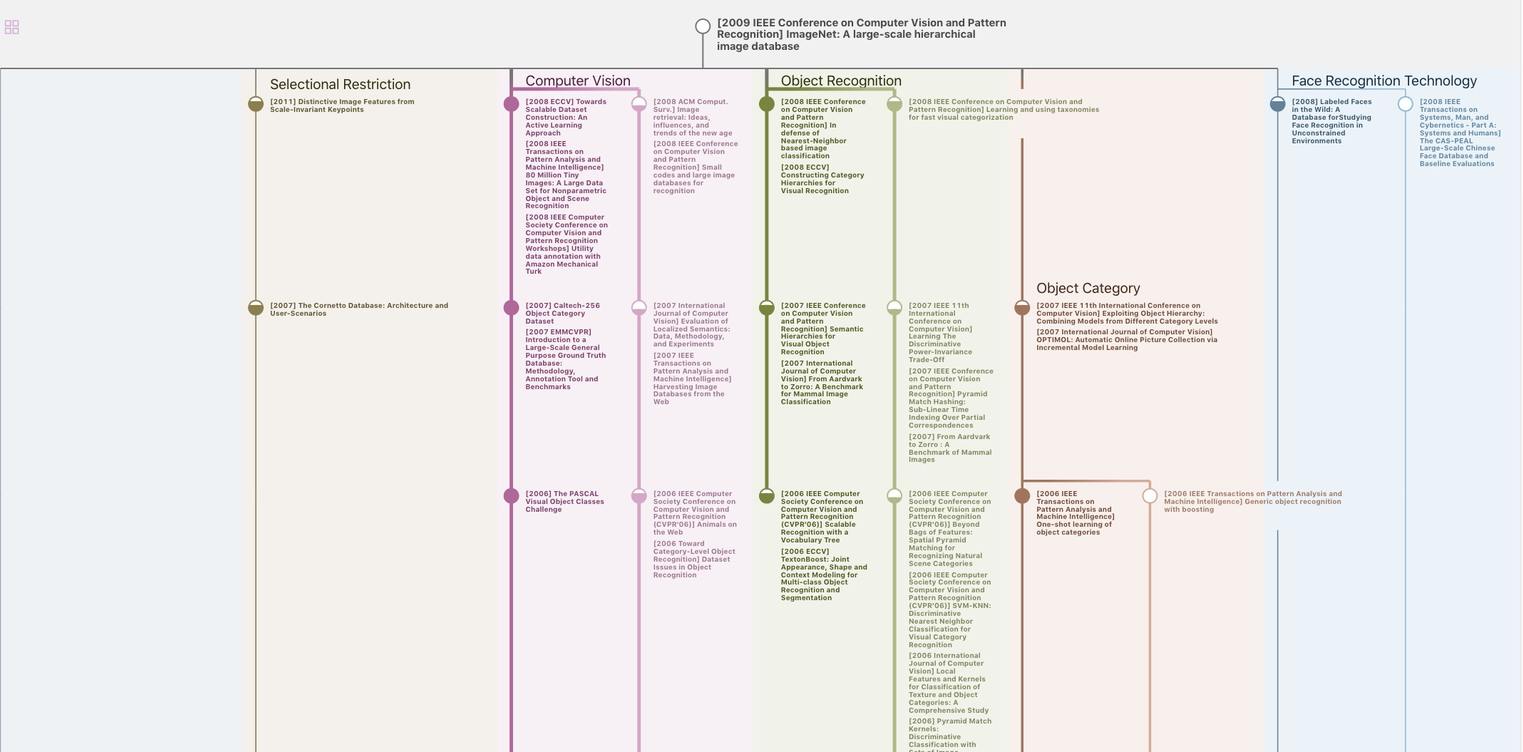
生成溯源树,研究论文发展脉络
Chat Paper
正在生成论文摘要