How machine learning won the Higgs boson challenge.
ESANN(2016)
摘要
In 2014 we ran a very successful machine learning challenge in High Ern-ergy physics attracting 1785 teams, which exposed the machine learning community for the first time to the problem of learning to discover (www.kaggle.com/c/higgs-boson). While physicists had the opportunity to improve on the state-of-the-art using feature engineering based on physics principles, this was not the determining factor in winning the challenge. Rather, the challenge revealed that the central difficulty of the problem is to develop a strategy to optimize directly the Approximate Median Significance (AMS) objective function, which is a particularly challenging and novel problem. This objective function aims at increasing the power of a statistical test. The top ranking learning machines span a variety of techniques including deep learning and gradient tree boosting. This paper presents the problem setting and analyzes the results.
更多查看译文
关键词
higgs boson,machine learning,challenge
AI 理解论文
溯源树
样例
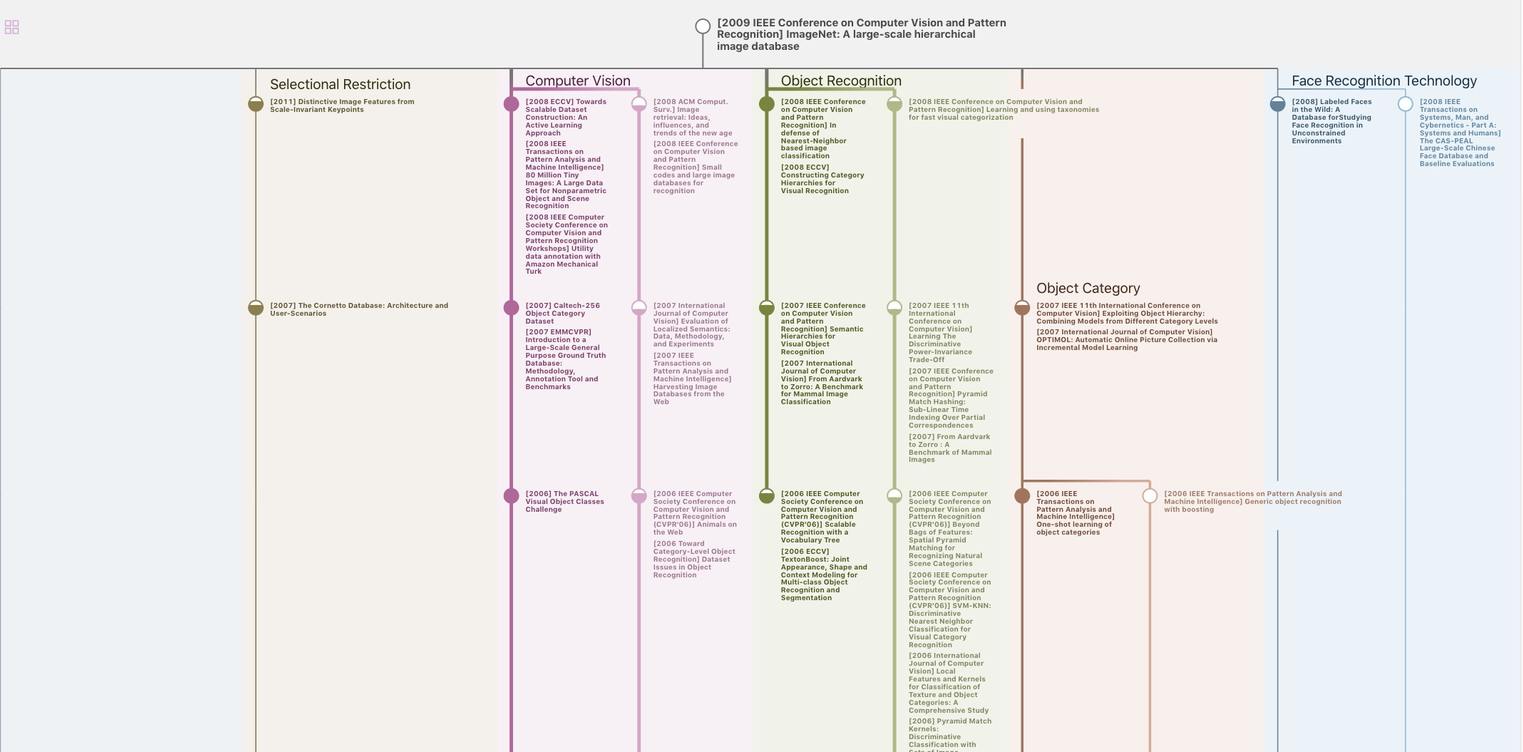
生成溯源树,研究论文发展脉络
Chat Paper
正在生成论文摘要