Inferring Temporal Structure From Predictability In Bumblebee Learning Flight
INTELLIGENT DATA ENGINEERING AND AUTOMATED LEARNING - IDEAL 2018, PT I(2018)
摘要
Insects are succeeding in remarkable navigational tasks. Bumblebees, for example, are capable of learning their nest location with sophisticated flight manoeuvres, forming a so-called learning flight. The learning flights - thought to be partially pre-programmed - enable the bumblebee to memorise spatial relations between its inconspicuous nest entrance and environmental cues. To date, environmental features (e.g. object positions on the eyes) and learning experience of the insect were used to describe the flights, but its structure, thought to facilitated learning, has not been investigated systematically. In this work, we present a novel approach, to examine whether and in which time span flight behaviour is predictable based on intrinsic properties only rather than external sensory information. We study the temporal composition of learning flights by estimating the smoothness of the underlying process. We then use echo state networks (ESN) and linear models (ARIMA) to predict the bumblebee trajectory from its past motion and identify different time-scales in learning flights using their prediction-power. We found that direct visual information is not necessary within a 200ms time-window to explain the bumblebee behaviour during its learning flight.
更多查看译文
关键词
Time series prediction, Modelling, Echo state network, Learning flight, Bumblebee
AI 理解论文
溯源树
样例
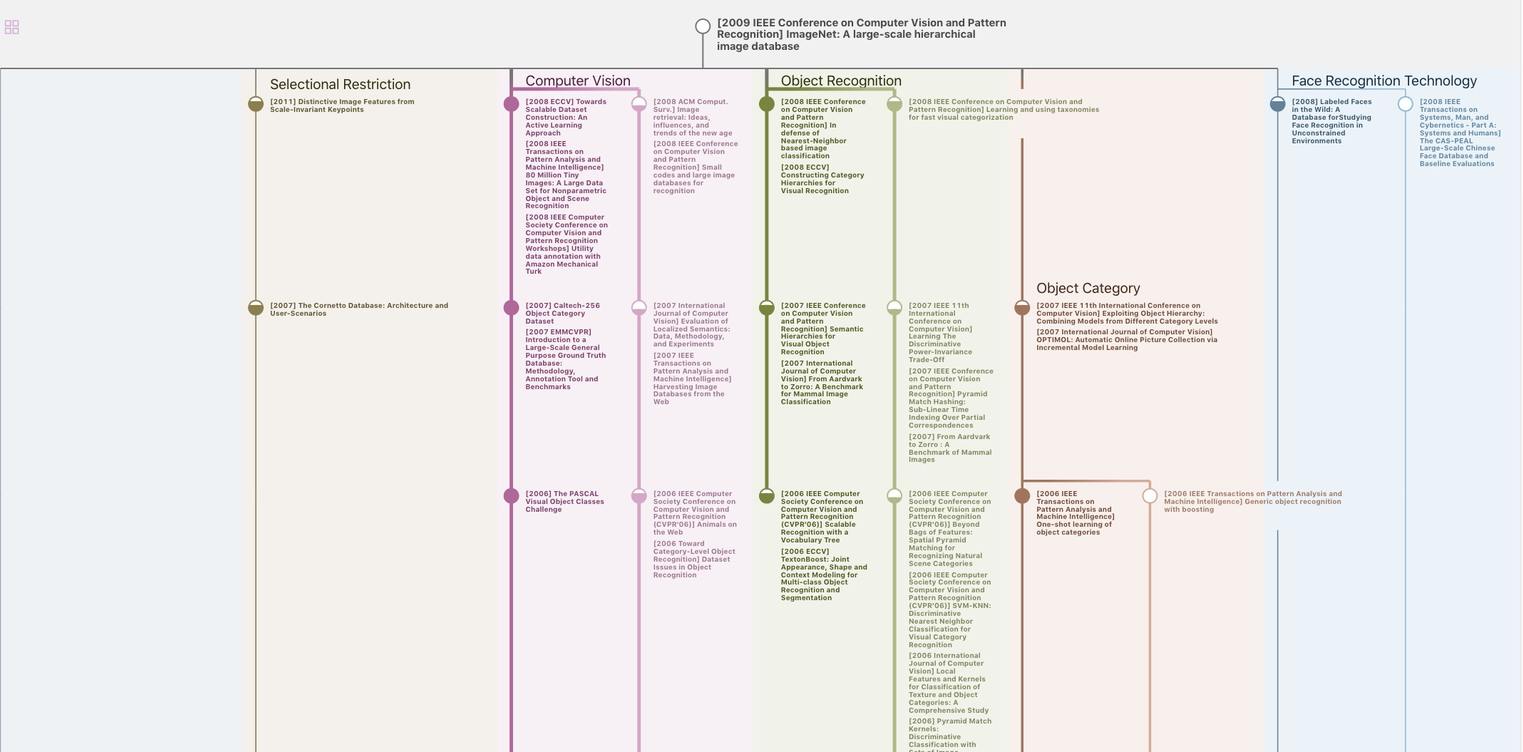
生成溯源树,研究论文发展脉络
Chat Paper
正在生成论文摘要