Automatic Sleep Stage Classification Using Single-Channel EEG: Learning Sequential Features with Attention-Based Recurrent Neural Networks.
EMBC(2018)
摘要
We propose in this work a feature learning approach using deep bidirectional recurrent neural networks (RNNs) with attention mechanism for single-channel automatic sleep stage classification. We firstly decompose an EEG epoch into multiple small frames and subsequently transform them into a sequence of frame-wise feature vectors. Given the training sequences, the attention-based RNN is trained in a sequence-to-label fashion for sleep stage classification. Due to discriminative training, the network is expected to encode information of an input sequence into a high-level feature vector after the attention layer. We, therefore, treat the trained network as a feature extractor and extract these feature vectors for classification which is accomplished by a linear SVM classifier. We also propose a discriminative method to learn a filter bank with a DNN for preprocessing purpose. Filtering the frame-wise feature vectors with the learned filter bank beforehand leads to further improvement on the classification performance. The proposed approach demonstrates good performance on the Sleep-EDF dataset.
更多查看译文
关键词
Electroencephalography,Humans,Neural Networks, Computer,Sleep Stages
AI 理解论文
溯源树
样例
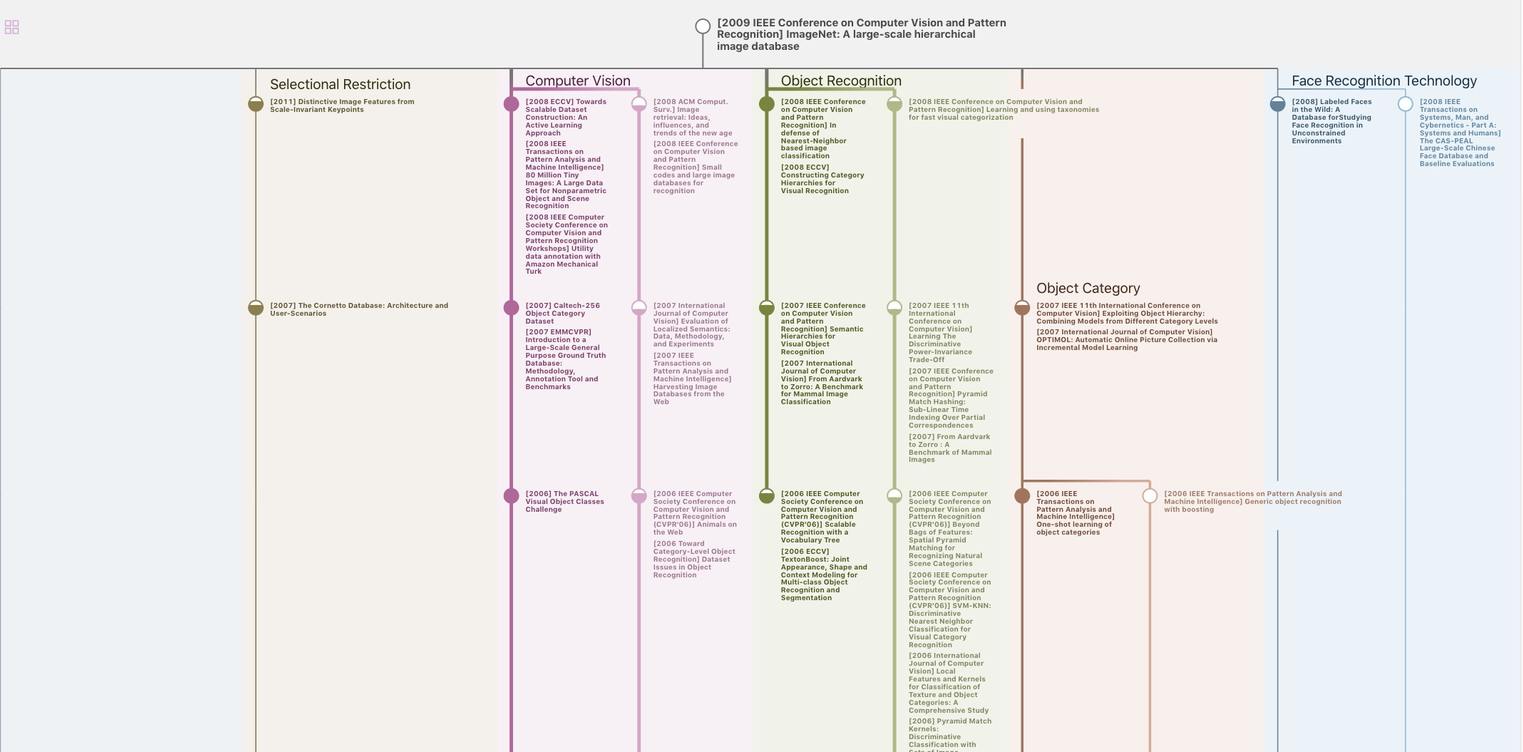
生成溯源树,研究论文发展脉络
Chat Paper
正在生成论文摘要