DNN Filter Bank Improves 1-Max Pooling CNN for Single-Channel EEG Automatic Sleep Stage Classification.
2018 40TH ANNUAL INTERNATIONAL CONFERENCE OF THE IEEE ENGINEERING IN MEDICINE AND BIOLOGY SOCIETY (EMBC)(2018)
摘要
We present in this paper an efficient convolutional neural network (CNN) running on time-frequency image features for automatic sleep stage classification. Opposing to deep architectures which have been used for the task, the proposed CNN is much simpler However, the CNN's convolutional layer is able to support convolutional kernels with different sizes, and therefore, capable of learning features at multiple temporal resolutions. In addition, the 1-max pooling strategy is employed at the pooling layer to better capture the shift-invariance property of EEG signals. We further propose a method to discriminatively learn a frequency-domain filter bank with a deep neural network (DNN) to preprocess the time-frequency image features. Our experiments show that the proposed 1-max pooling CNN performs comparably with the very deep CNNs in the literature on the Sleep- EDF dataset. Preprocessing the time-frequency image features with the learned filter bank before presenting them to the CNN leads to significant improvements on the classification accuracy, setting the state- of-the-art performance on the dataset.
更多查看译文
关键词
Algorithms,Electroencephalography,Humans,Neural Networks, Computer,Sleep Stages
AI 理解论文
溯源树
样例
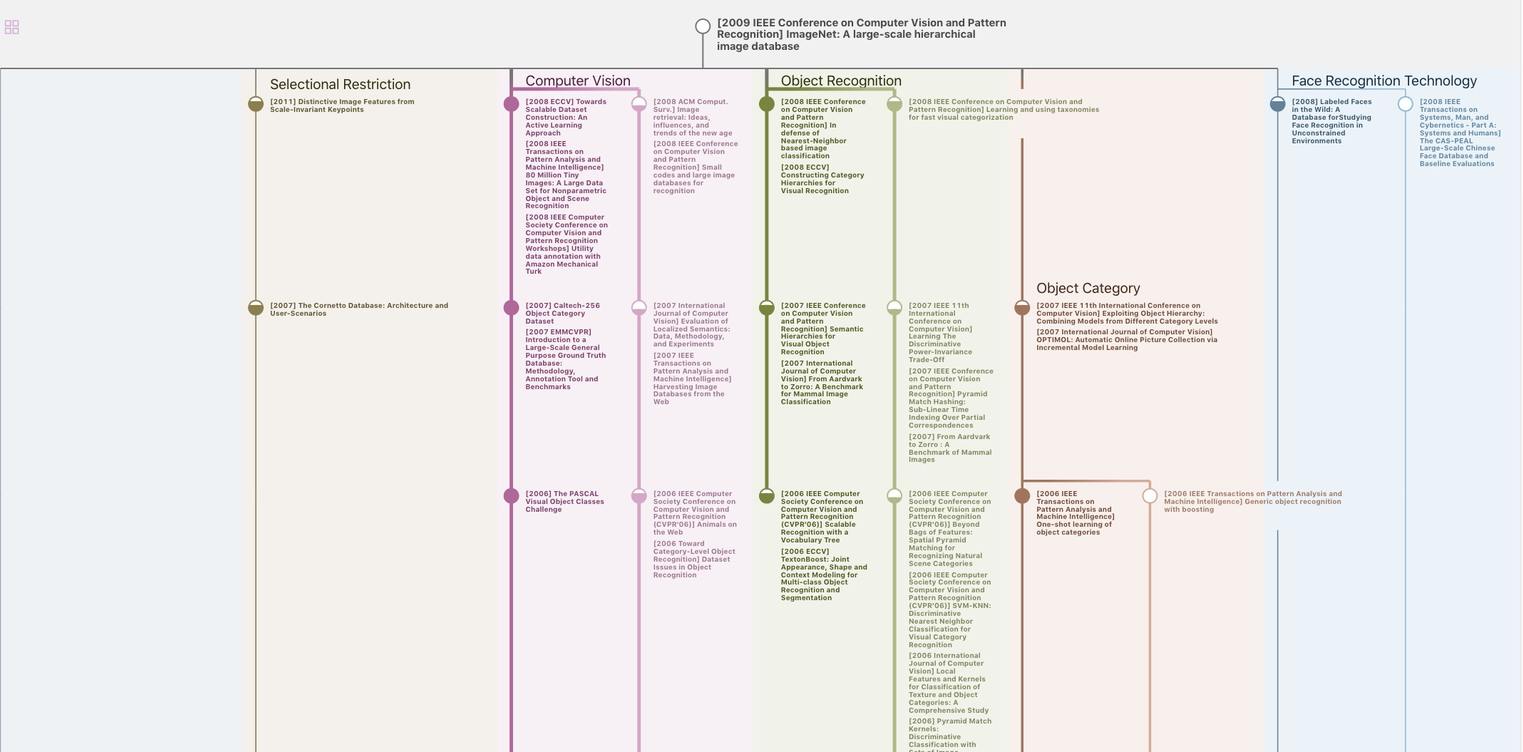
生成溯源树,研究论文发展脉络
Chat Paper
正在生成论文摘要