Multichannel Sleep Stage Classification and Transfer Learning using Convolutional Neural Networks
2018 40th Annual International Conference of the IEEE Engineering in Medicine and Biology Society (EMBC)(2018)
摘要
Current sleep medicine relies on the supervised analysis of polysomnographic measurements, comprising amongst others electroencephalogram (EEG), electromyogram (EMG), and electrooculogram (EOG) signals. Convolutional neural networks (CNN) provide an interesting framework to automated classification of sleep based on these raw waveforms. In this study, we compare existing CNN approaches to four databases of pathological and physiological subjects. The best performing model resulted in Cohen's Kappa of κ = 0.75 on healthy subjects and κ = 0.64 on patients suffering from a variety of sleep disorders. Further, we show the advantages of additional sensor data (i.e. EOG and EMG). Deep learning approaches require a lot of data which is scarce for less prevalent diseases. For this, we propose a transfer learning procedure by pretraining a model on large public data and fine-tune this on each subject from a smaller dataset. This procedure is demonstrated using a private REM Behaviour Disorder database, improving sleep classification by 24.4%.
更多查看译文
关键词
Databases, Factual,Deep Learning,Electroencephalography,Electromyography,Electrooculography,Humans,Neural Networks, Computer,Sleep,Sleep Stages,Sleep Wake Disorders,Transfer, Psychology
AI 理解论文
溯源树
样例
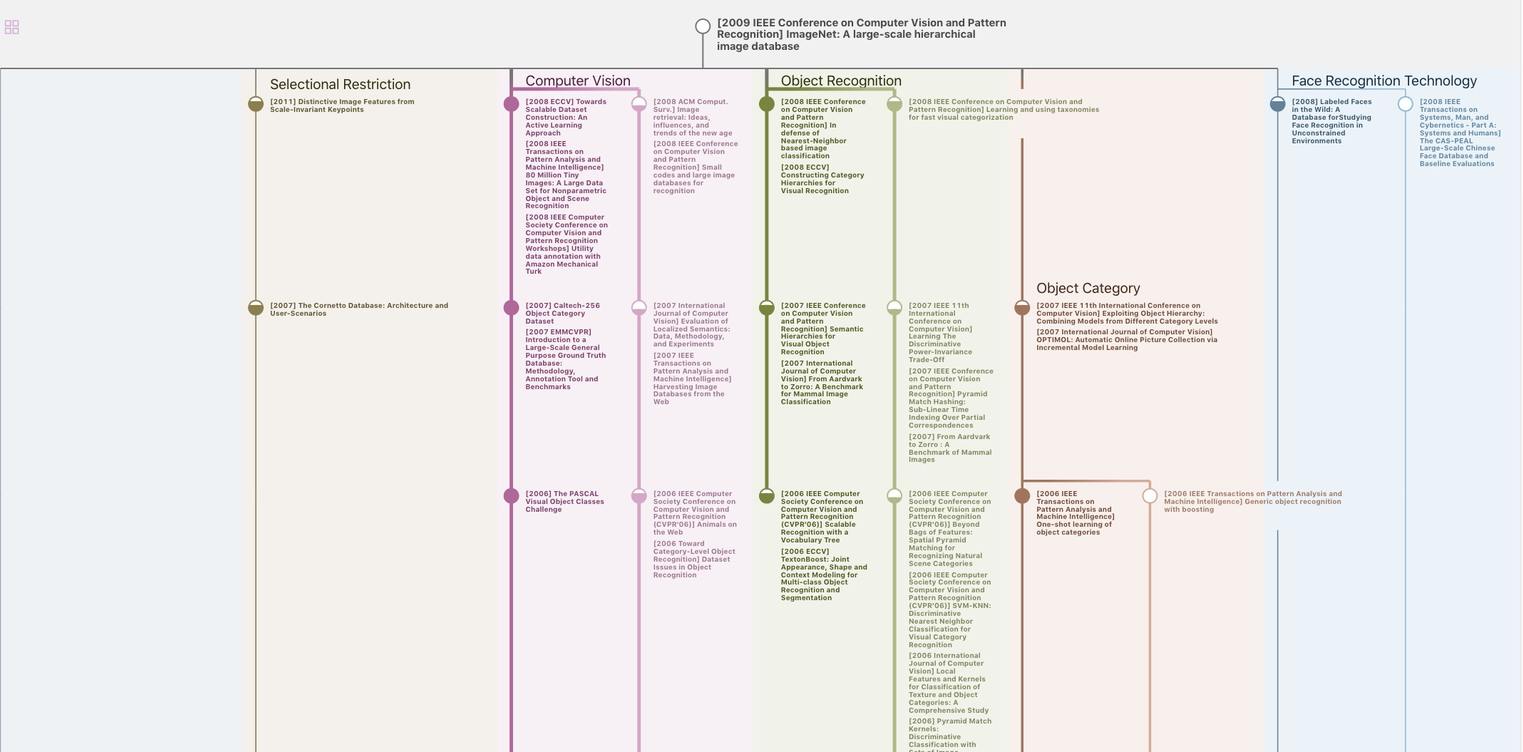
生成溯源树,研究论文发展脉络
Chat Paper
正在生成论文摘要