Using musical relationships between chord labels in automatic chord extraction tasks
ISMIR(2019)
摘要
Recent researches on Automatic Chord Extraction (ACE) have focused on the improvement of models based on machine learning. However, most models still fail to take into account the prior knowledge underlying the labeling alphabets (chord labels). Furthermore, recent works have shown that ACE performances are converging towards a glass ceiling. Therefore, this prompts the need to focus on other aspects of the task, such as the introduction of musical knowledge in the representation, the improvement of the models towards more complex chord alphabets and the development of more adapted evaluation methods. In this paper, we propose to exploit specific properties and relationships between chord labels in order to improve the learning of statistical ACE models. Hence, we analyze the interdependence of the representations of chords and their associated distances, the precision of the chord alphabets, and the impact of the reduction of the alphabet before or after training of the model. Furthermore, we propose new training losses based on musical theory. We show that these improve the results of ACE systems based on Convolutional Neural Networks. By performing an in-depth analysis of our results, we uncover a set of related insights on ACE tasks based on statistical models, and also formalize the musical meaning of some classification errors.
更多查看译文
AI 理解论文
溯源树
样例
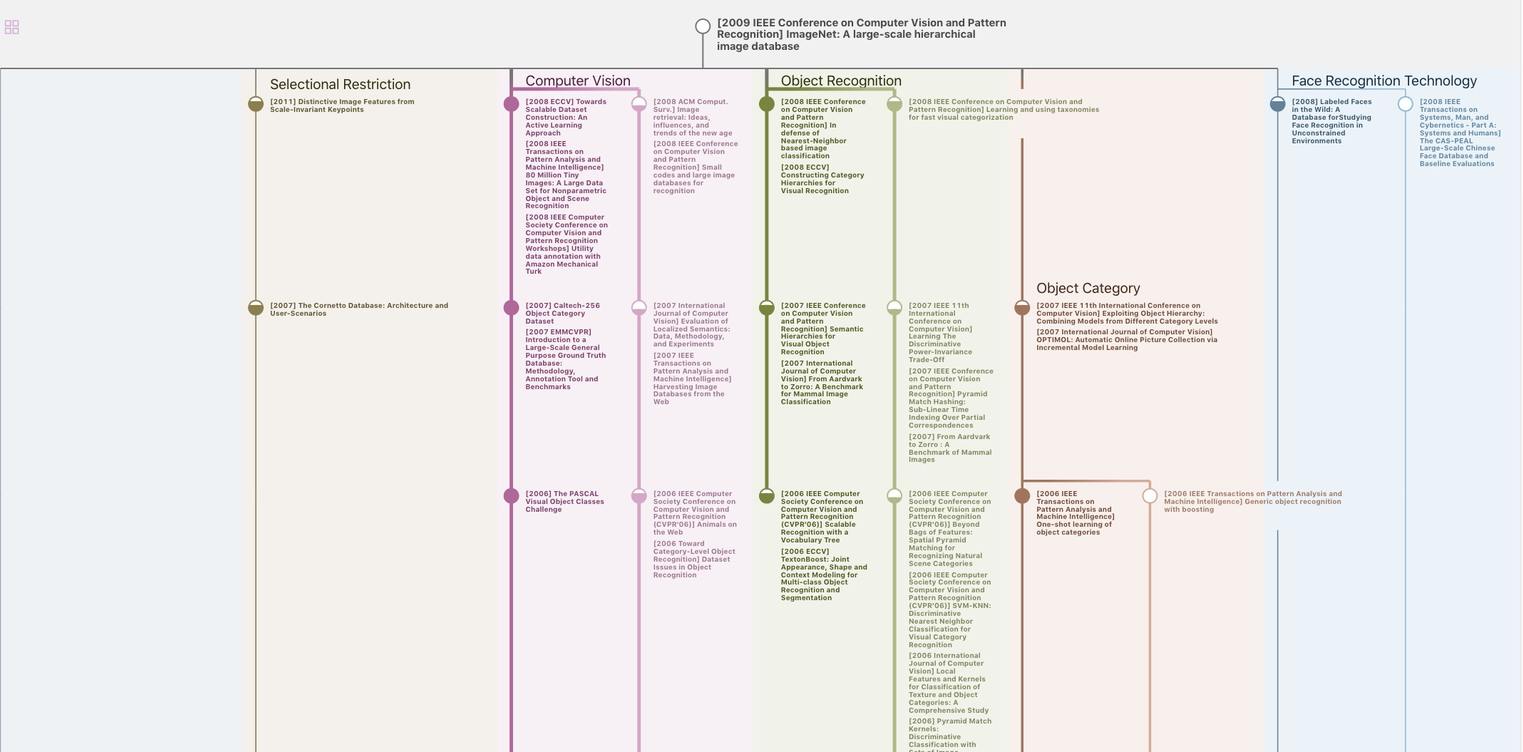
生成溯源树,研究论文发展脉络
Chat Paper
正在生成论文摘要