Subset Replay Based Continual Learning for Scalable Improvement of Autonomous Systems
2018 IEEE/CVF Conference on Computer Vision and Pattern Recognition Workshops (CVPRW)(2018)
摘要
While machine learning techniques have come a long way in showing astounding performance on various vision problems, the conventional way of training is not applicable for learning from a sequence of new data or tasks. For most real life applications like perception for autonomous vehicles, multiple stages of data collection are necessary to improve the performance of machine learning models over time. The newer observations may have a different distribution than the older ones and thus a simply fine-tuned model often overfits while forgetting the knowledge from past experiences. Recently, few lifelong or continual learning approaches have shown promising results towards overcoming this problem of catastrophic forgetting. In this work, we show that carefully choosing a small subset of the older data with the objective of promoting representativeness and diversity can also help in learning continuously. For large scale cloud based training, this can help in significantly reducing the amount of storage required along with lessening the computation and time for each retraining session.
更多查看译文
关键词
subset replay,continual learning,autonomous systems,vision problems,autonomous vehicles,data collection,machine learning models,large scale cloud based training,lifelong learning
AI 理解论文
溯源树
样例
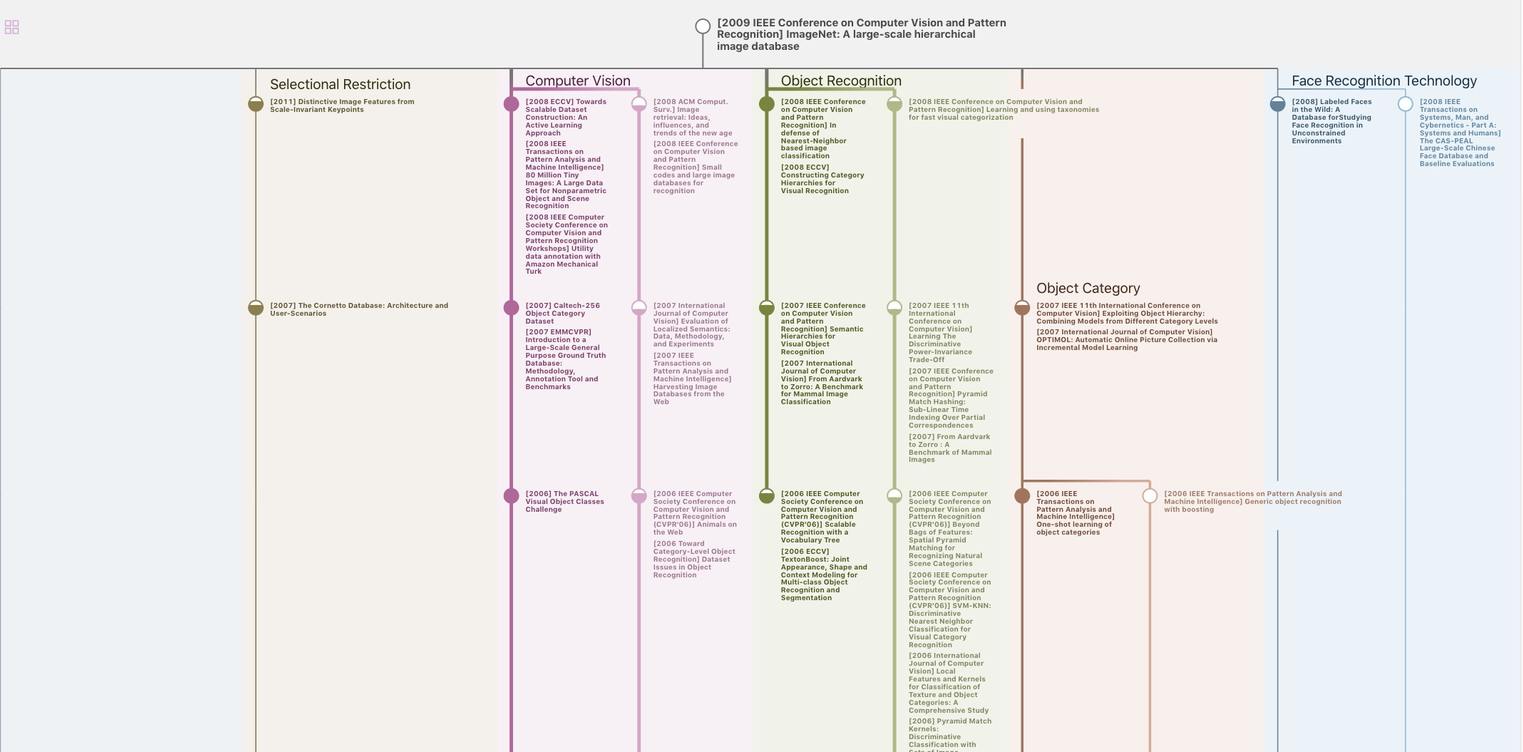
生成溯源树,研究论文发展脉络
Chat Paper
正在生成论文摘要