Active Learning For Semiautomatic Sleep Staging And Transitional Eeg Segments
PROCEEDINGS 2018 IEEE INTERNATIONAL CONFERENCE ON BIOINFORMATICS AND BIOMEDICINE (BIBM)(2018)
摘要
A semiautomatic sleep staging system based on EEG classification is focused in the paper. Such an expert-in-the-loop system interacts with the annotating expert by suggesting him EEG segments that should be annotated. After a sufficient number of labeled segments is reached, a pattern classification model is trained and used for automatic annotation of the rest of the signal. It is shown that this can save 85% of the labeling effort and consequently improve the annotation quality due to the prevention of errors caused by expert's fatigue. The selection of the data for labeling is based on confidence based active learning approach. It is shown that such a strategy is statistically significantly better in terms of mean class error than baseline random sampling strategy. Moreover, it is argued that transitional instances that correspond to transitions between sleep stages are often erroneously labeled and their elimination can improve especially the active learning process. This hypothesis is examined and surprisingly such elimination significantly improved the random sampling strategy which became comparable to the active learning without removal of transitions. Although the active learning strategy with transitions removal performed better in terms of mean, there were not sufficient data that would prove this statistically.
更多查看译文
关键词
EEG, sleep staging, active learning, machine learning, doctor-in-the-loop
AI 理解论文
溯源树
样例
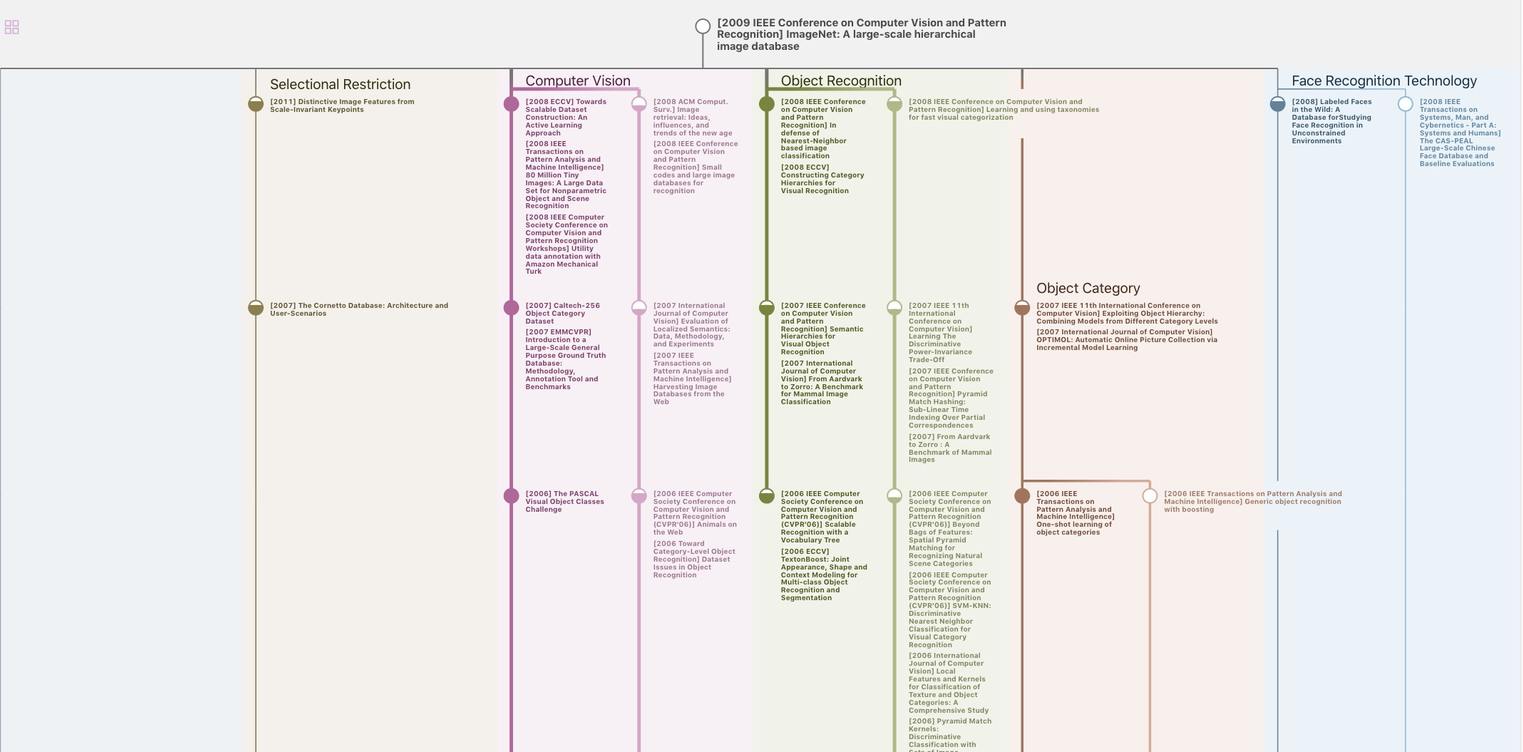
生成溯源树,研究论文发展脉络
Chat Paper
正在生成论文摘要