Deep Learning for End-to-End Atrial Fibrillation Recurrence Estimation
arXiv (Cornell University)(2018)
摘要
Left atrium shape has been shown to be an independent predictor of recurrence after atrial fibrillation (AF) ablation. Shape-based representation is imperative to such an estimation process, where correspondence-based representation offers the most flexibility and ease-of-computation for population-level shape statistics. Nonetheless, population-level shape representations in the form of image segmentation and correspondence models derived from cardiac MRI require significant human resources with sufficient anatomy-specific expertise. In this paper, we propose a machine learning approach that uses deep networks to estimate AF recurrence by predicting shape descriptors directly from MRI images, with NO image pre-processing involved. We also propose a novel data augmentation scheme to effectively train a deep network in a limited training data setting. We compare this new method of estimating shape descriptors from images with the state-of-the-art correspondence-based shape modeling that requires image segmentation and correspondence optimization. Results show that the proposed method and the current state-of-the-art produce statistically similar outcomes on AF recurrence, eliminating the need for expensive pre-processing pipelines and associated human labor.
更多查看译文
关键词
data augmentation scheme,population-level shape representations,population-level shape statistics,estimation process,shape-based representation,atrial fibrillation ablation,end-to-end atrial fibrillation recurrence estimation,correspondence optimization,image segmentation,image pre-processing,MRI images,shape descriptors,cardiac MRI
AI 理解论文
溯源树
样例
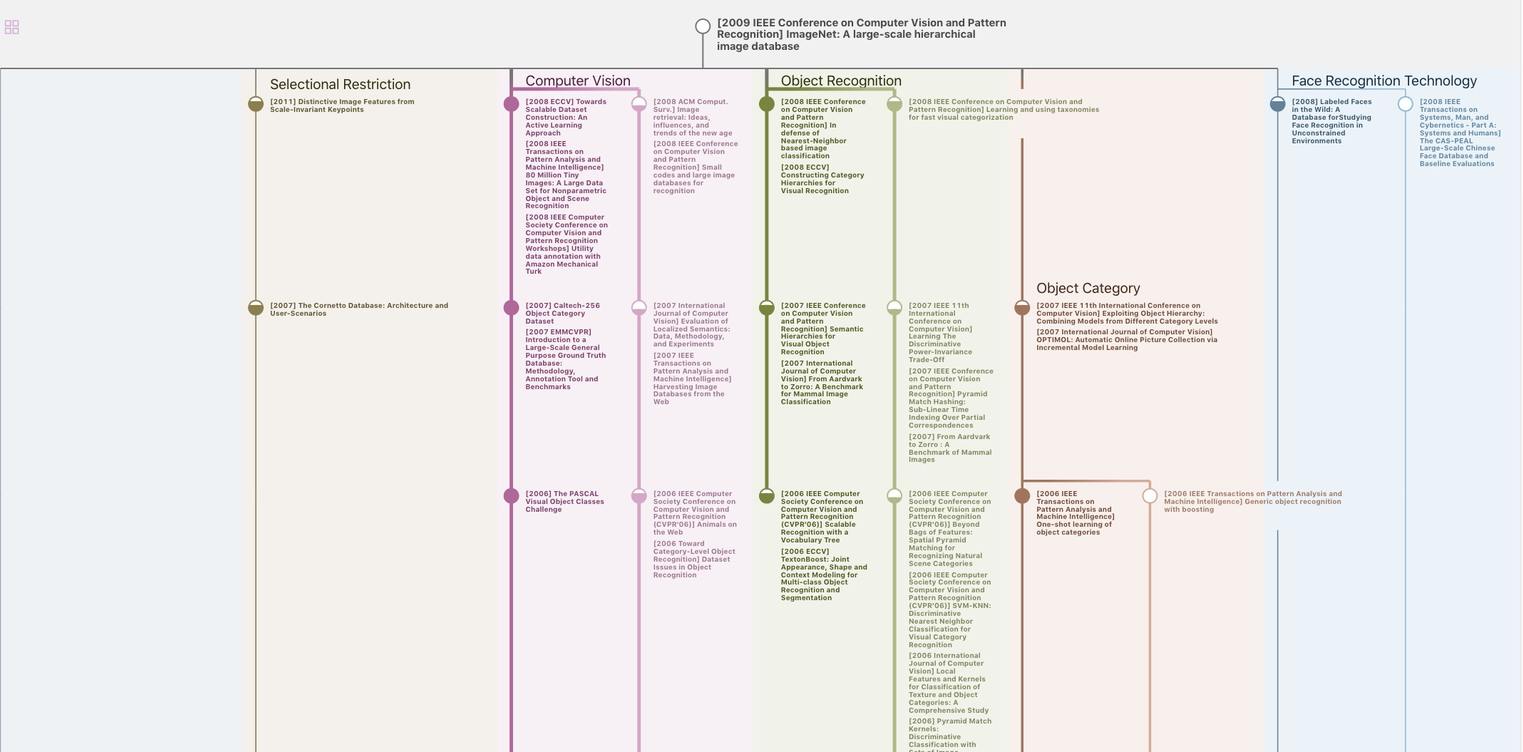
生成溯源树,研究论文发展脉络
Chat Paper
正在生成论文摘要