Interpretability is Harder in the Multiclass Setting: Axiomatic Interpretability for Multiclass Additive Models.
arXiv: Learning(2018)
摘要
Generalized additive models (GAMs) are favored in many regression and binary classification problems because they are able to fit complex, nonlinear functions while still remaining interpretable. In the first part of this paper, we generalize a state-of-the-art GAM learning algorithm based on boosted trees to the multiclass setting, and show that this multiclass algorithm outperforms existing GAM fitting algorithms and sometimes matches the performance of full complex models. In the second part, we turn our attention to the interpretability of GAMs in the multiclass setting. Surprisingly, the natural interpretability of GAMs breaks down when there are more than two classes. Drawing inspiration from binary GAMs, we identify two axioms that any additive model must satisfy to not be visually misleading. We then develop a post-processing technique (API) that provably transforms pretrained additive models to satisfy the interpretability axioms without sacrificing accuracy. The technique works not just on models trained with our algorithm, but on any multiclass additive model. We demonstrate API on a 12-class infant-mortality dataset.
更多查看译文
关键词
additive models, interpretable model, machine learning
AI 理解论文
溯源树
样例
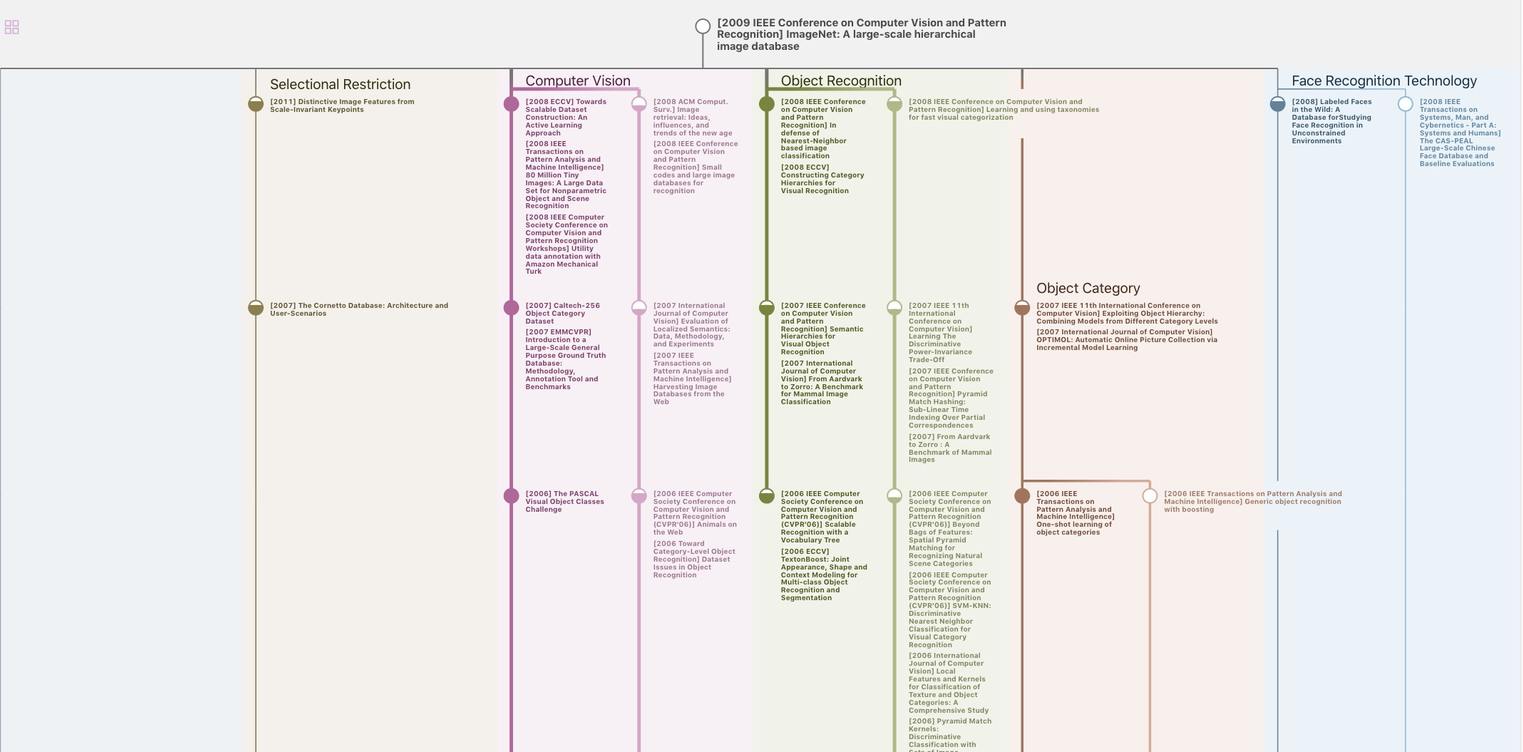
生成溯源树,研究论文发展脉络
Chat Paper
正在生成论文摘要