IL-Net: Using Expert Knowledge to Guide the Design of Furcated Neural Networks
2018 IEEE INTERNATIONAL CONFERENCE ON BIG DATA (BIG DATA)(2018)
摘要
Deep neural networks (DNN) excel at extracting patterns. Through representation learning and automated feature engineering on large datasets, such models have been highly successful in computer vision and natural language applications. Designing optimal network architectures from a principled or rational approach however has been less than successful, with the best successful approaches utilizing an additional machine learning algorithm to tune the network hyperparameters. However, in many technical fields, there exist established domain knowledge and understanding about the subject matter. In this work, we develop a novel furcated neural network architecture that utilizes domain knowledge as high-level design principles of the network. We demonstrate proof-of-concept by developing IL-Net, a furcated network for predicting the properties of ionic liquids, which is a class of complex multi-chemicals entities. Compared to existing state-of-the-art approaches, we show that furcated networks can improve model accuracy by approximately 20-35%, without using additional labeled data. Lastly, we distill two key design principles for furcated networks that can be adapted to other domains.
更多查看译文
关键词
neural networks,expert knowledge,il-net
AI 理解论文
溯源树
样例
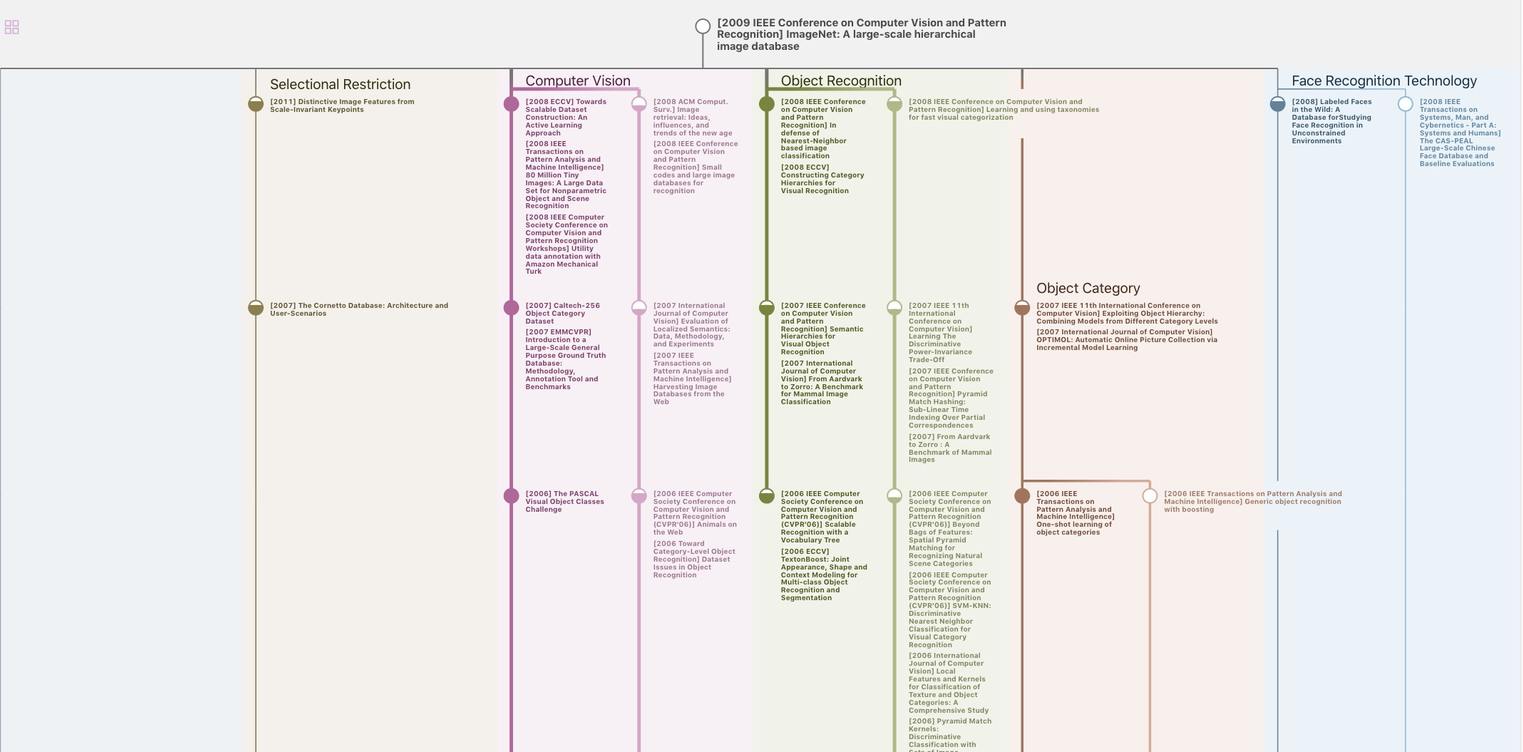
生成溯源树,研究论文发展脉络
Chat Paper
正在生成论文摘要