Local module identification in dynamic networks with correlated noise: the full input case
2019 IEEE 58th Conference on Decision and Control (CDC)(2019)
摘要
The identification of local modules in dynamic networks with known topology has recently been addressed by formulating conditions for arriving at consistent estimates of the module dynamics, typically under the assumption of having disturbances that are uncorrelated over the different nodes. The conditions typically reflect the selection of a set of node signals that are taken as predictor inputs in a MISO identification setup. In this paper an extension is made to arrive at an identification setup for the situation that process noises on the different node signals can be correlated with each other. In this situation the local module may need to be embedded in a MIMO identification setup for arriving at a consistent estimate with maximum likelihood properties. This requires the proper treatment of confounding variables. The result is an algorithm that, based on the given network topology and disturbance correlation structure, selects an appropriate set of node signals as predictor inputs and outputs in a MISO or MIMO identification setup. As a first step in the analysis, we restrict attention to the (slightly conservative) situation where the selected output node signals are predicted based on all of their in-neighbor node signals in the network.
更多查看译文
关键词
local module identification,dynamic networks,correlated noise,module dynamics,predictor inputs,MISO identification setup,process noises,MIMO identification setup,network topology,disturbance correlation structure,selected output node signals,in-neighbor node signals,maximum likelihood properties
AI 理解论文
溯源树
样例
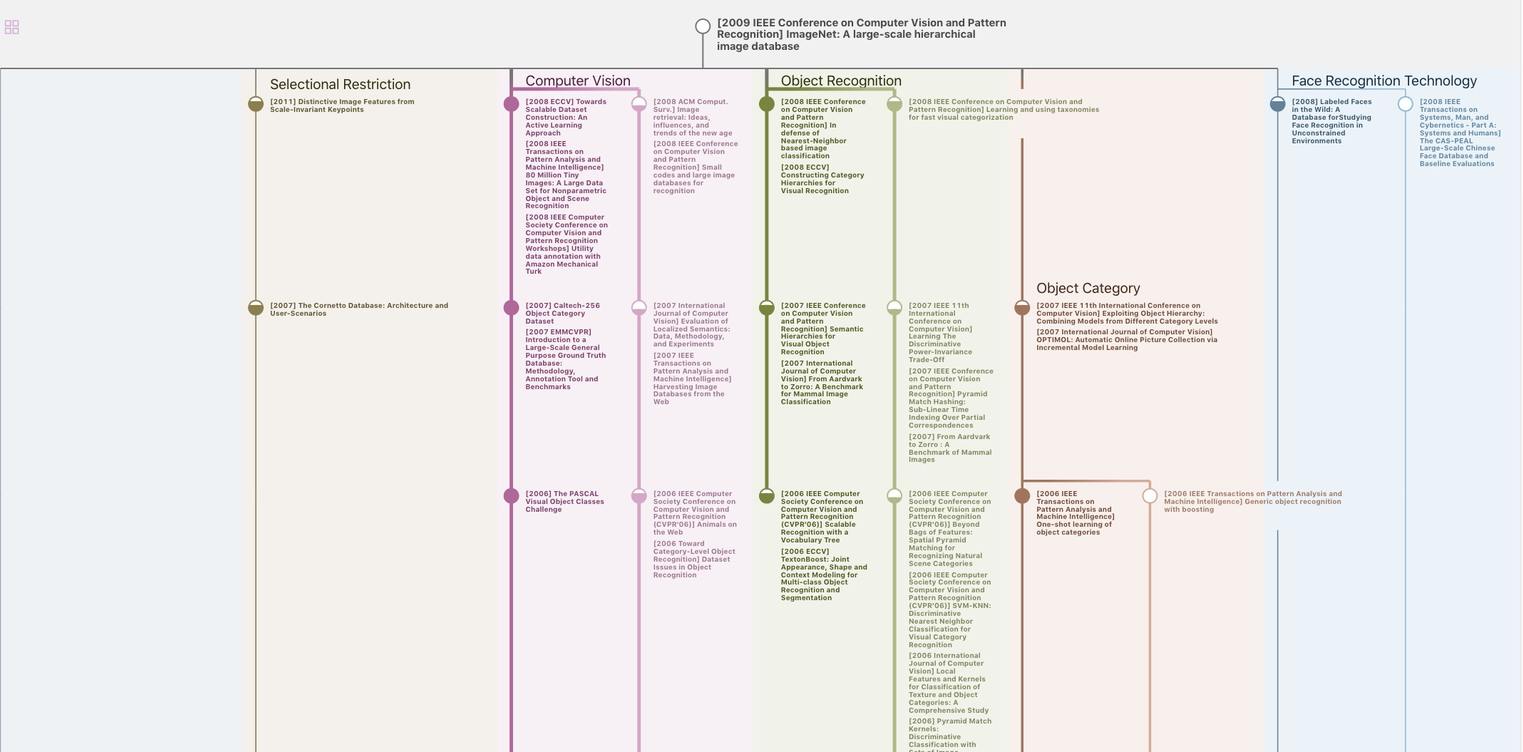
生成溯源树,研究论文发展脉络
Chat Paper
正在生成论文摘要