Explainable Time Series Tweaking via Irreversible and Reversible Temporal Transformations
2018 IEEE International Conference on Data Mining (ICDM)(2018)
摘要
Time series classification has received great attention over the past decade with a wide range of methods focusing on predictive performance by exploiting various types of temporal features. Nonetheless, little emphasis has been placed on interpretability and explainability. In this paper, we formulate the novel problem of explainable time series tweaking, where, given a time series and an opaque classifier that provides a particular classification decision for the time series, we want to find the minimum number of changes to be performed to the given time series so that the classifier changes its decision to another class. We show that the problem is NP-hard, and focus on two instantiations of the problem, which we refer to as reversible and irreversible time series tweaking. The classifier under investigation is the random shapelet forest classifier. Moreover, we propose two algorithmic solutions for the two problems along with simple optimizations, as well as a baseline solution using the nearest neighbor classifier. An extensive experimental evaluation on a variety of real datasets demonstrates the usefulness and effectiveness of our problem formulation and solutions.
更多查看译文
关键词
time series classification,interpretability,explainability,time series tweaking
AI 理解论文
溯源树
样例
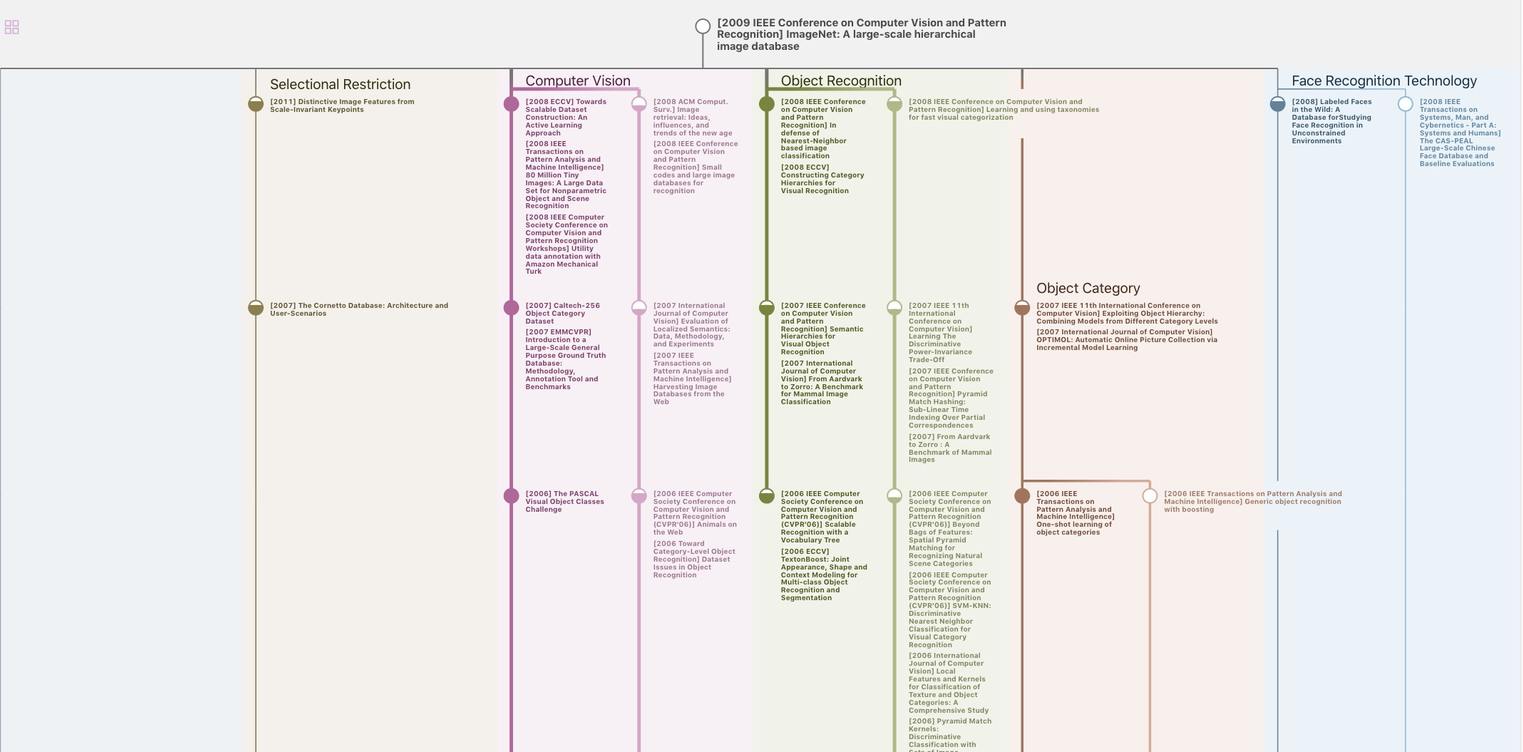
生成溯源树,研究论文发展脉络
Chat Paper
正在生成论文摘要