Quantifying Learning Guarantees For Convex But Inconsistent Surrogates
ADVANCES IN NEURAL INFORMATION PROCESSING SYSTEMS 31 (NIPS 2018)(2018)
摘要
We study consistency properties of machine learning methods based on minimizing convex surrogates. We extend the recent framework of Osokin et al. [14] for the quantitative analysis of consistency properties to the case of inconsistent surrogates. Our key technical contribution consists in a new lower bound on the calibration function for the quadratic surrogate, which is non-trivial (not always zero) for inconsistent cases. The new bound allows to quantify the level of inconsistency of the setting and shows how learning with inconsistent surrogates can have guarantees on sample complexity and optimization difficulty. We apply our theory to two concrete cases: multi-class classification with the tree-structured loss and ranking with the mean average precision loss. The results show the approximation-computation trade-offs caused by inconsistent surrogates and their potential benefits.
更多查看译文
关键词
sample complexity,quantitative analysis,mean average precision,parallel optimization,hierarchical classification
AI 理解论文
溯源树
样例
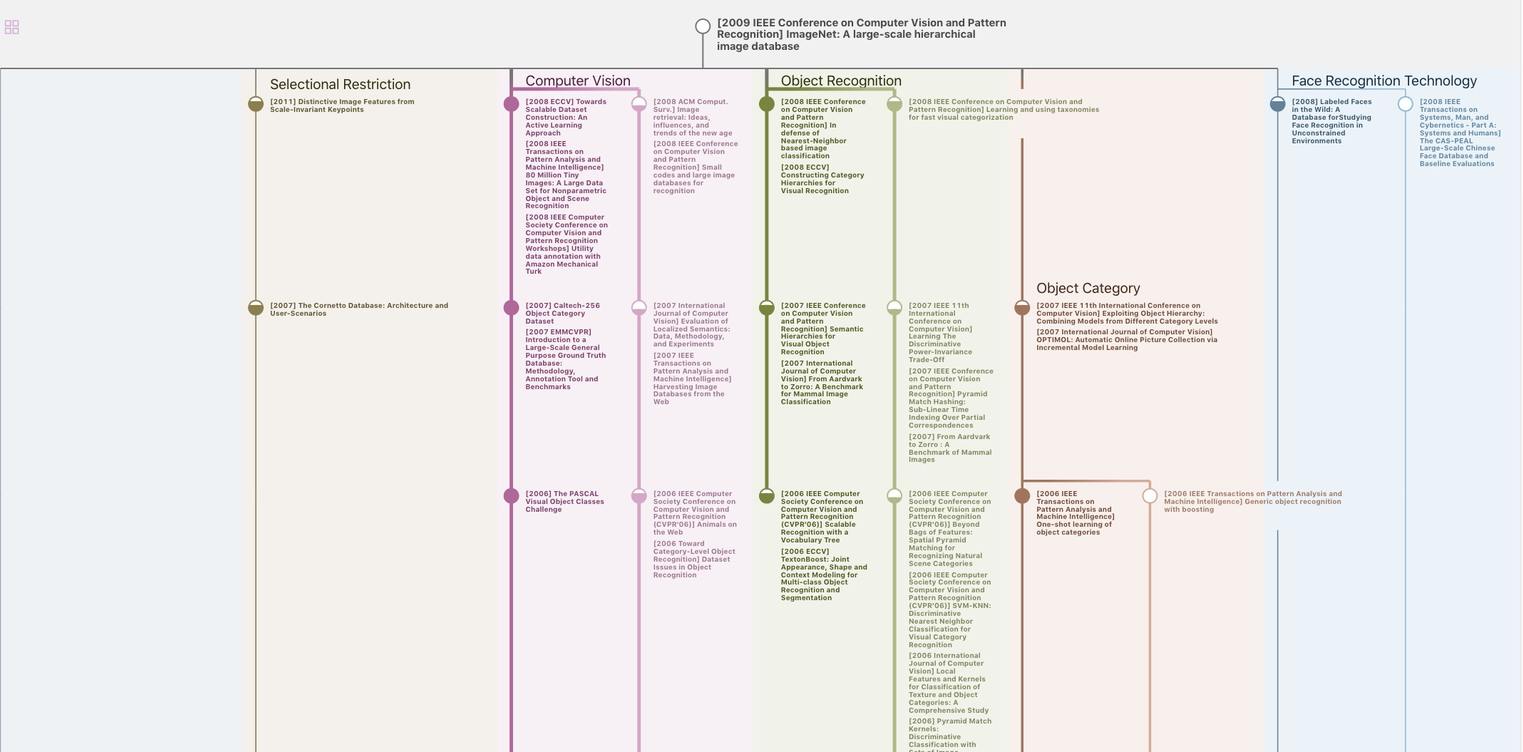
生成溯源树,研究论文发展脉络
Chat Paper
正在生成论文摘要