On the relationship between Dropout and Equiangular Tight Frames.
arXiv: Learning(2018)
摘要
Dropout is a popular regularization technique in neural networks. Yet, the reason for its success is still not fully understood. This paper provides a new interpretation of Dropout from a frame theory perspective. This leads to a novel regularization technique for neural networks that minimizes the cross-correlation between filters in the network. We demonstrate its applicability in convolutional and fully connected layers in both feed-forward and recurrent networks.
更多查看译文
AI 理解论文
溯源树
样例
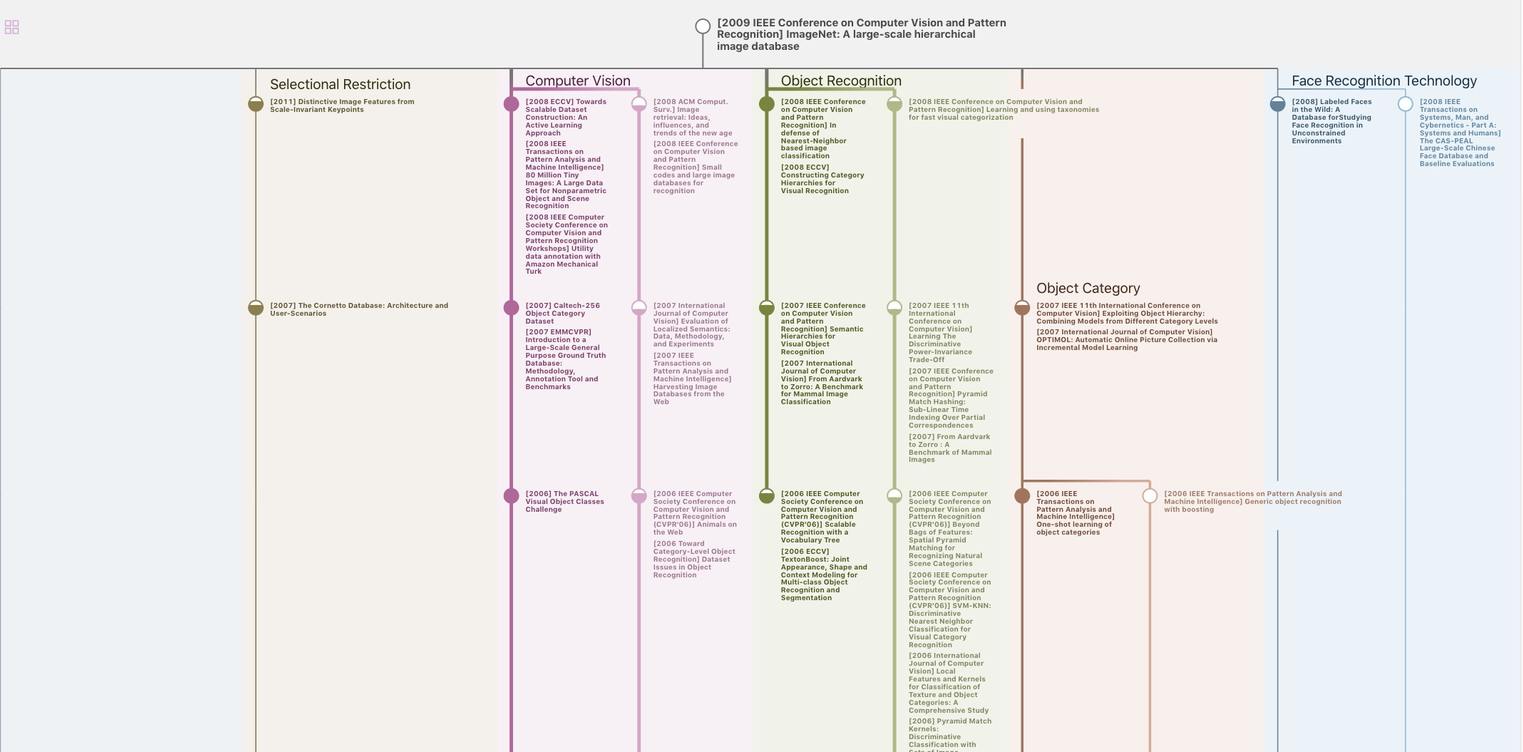
生成溯源树,研究论文发展脉络
Chat Paper
正在生成论文摘要