Actor-Expert: A Framework for using Action-Value Methods in Continuous Action Spaces.
arXiv: Learning(2018)
摘要
Value-based approaches can be difficult to use in continuous action spaces, because an optimization has to be solved to find the greedy action for the action-values. A common strategy has been to restrict the functional form of the action-values to be convex or quadratic in the actions, to simplify this optimization. Such restrictions, however, can prevent learning accurate action-values. In this work, we propose the Actor-Expert framework for value-based methods, that decouples action-selection (Actor) from the action-value representation (Expert). The Expert uses Q-learning to update the action-values towards the optimal action-values, whereas the Actor (learns to) output the greedy action for the current action-values. We develop a Conditional Cross Entropy Method for the Actor, to learn the greedy action for a generically parameterized Expert, and provide a two-timescale analysis to validate asymptotic behavior. We demonstrate in a toy domain with bimodal action-values that previous restrictive action-value methods fail whereas the decoupled Actor-Expert with a more general action-value parameterization succeeds. Finally, we demonstrate that Actor-Expert performs as well as or better than these other methods on several benchmark continuous-action domains.
更多查看译文
AI 理解论文
溯源树
样例
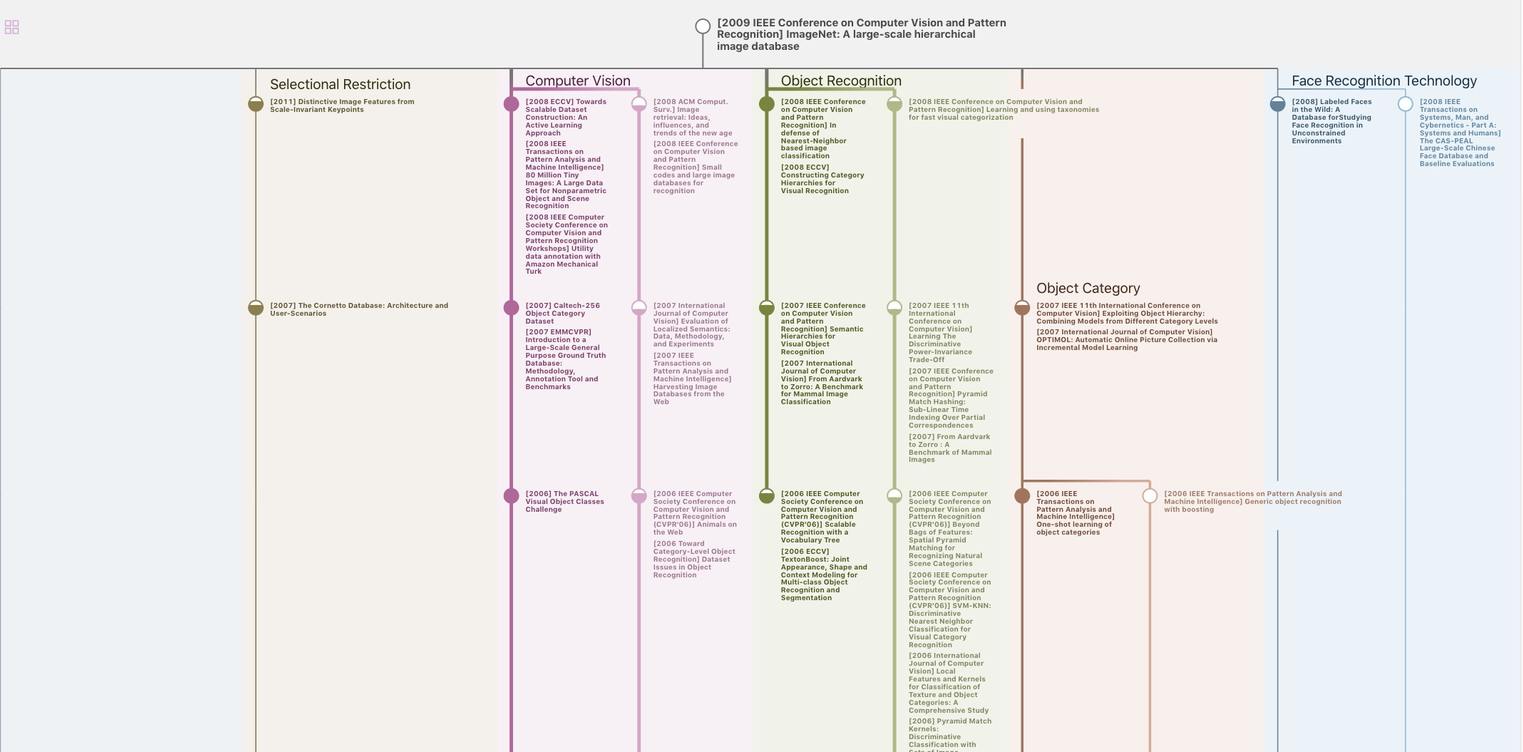
生成溯源树,研究论文发展脉络
Chat Paper
正在生成论文摘要