Fast Streaming Behavioural Pattern Mining
New Generation Computing(2018)
摘要
Identification of typical user behaviour within a web application is a crucial assumption for revealing user characteristics, preferences and habits. Typical and repeating features of user behaviour during his/her interaction with web application can be generalized through behavioural patterns. In this paper, we propose HyBPMine —a novel method, for behavioural pattern mining over a data stream. Our method combines global patterns with patterns specific to dynamically identified groups of similar users. In this way, the method finds and combines the general global patterns (typical for high number of users) with the specific patterns (typical for user groups). We represent the patterns as frequent closed itemsets of items visited by users in their sessions. The behavioural patterns are often used for personalization, prediction or recommendation. In this paper, we evaluated the performance of our method indirectly, by applying discovered patterns in personalized recommendations. In other words, we recommended next user actions within the actual user session. We performed several experiments over data from e-learning and news domains. Our results clearly show that proposed method reaches higher precision than its components used individually as well as the state-of-the-art approaches. In addition, a inclusion of group patterns brings only low and constant computational load, which does not significantly increase resource requirements.
更多查看译文
关键词
Adaptation,Behavioural patterns,Clustering,Data stream,Frequent itemsets,Personalized recommendation
AI 理解论文
溯源树
样例
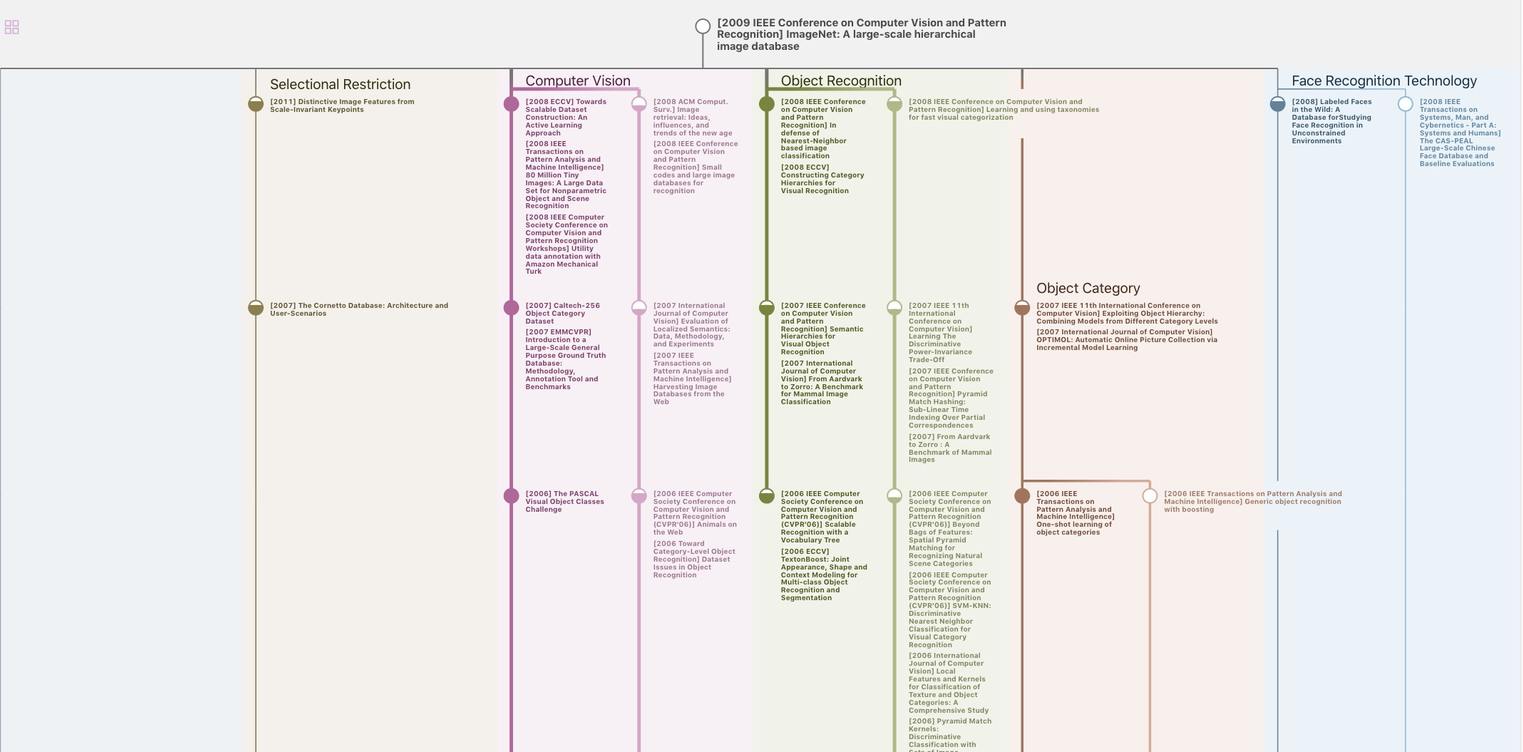
生成溯源树,研究论文发展脉络
Chat Paper
正在生成论文摘要