LSTM-based traffic flow prediction with missing data.
Neurocomputing(2018)
摘要
Traffic flow prediction plays a key role in intelligent transportation systems. However, since traffic sensors are typically manually controlled, traffic flow data with varying length, irregular sampling and missing data are difficult to exploit effectively. To overcome this problem, we propose a novel approach that is based on Long Short-Term Memory (LSTM) in this paper. In addition, the multiscale temporal smoothing is employed to infer lost data and the prediction residual is learned by our approach. We demonstrate the performance of our approach on both the Caltrans Performance Measurement System (PeMS) data set and our own traffic flow data set. According to the experimental results, our approach obtains higher accuracy in traffic flow prediction compared with other approaches.
更多查看译文
关键词
Traffic flow prediction,Intelligent transportation systems,Deep learning,LSTM
AI 理解论文
溯源树
样例
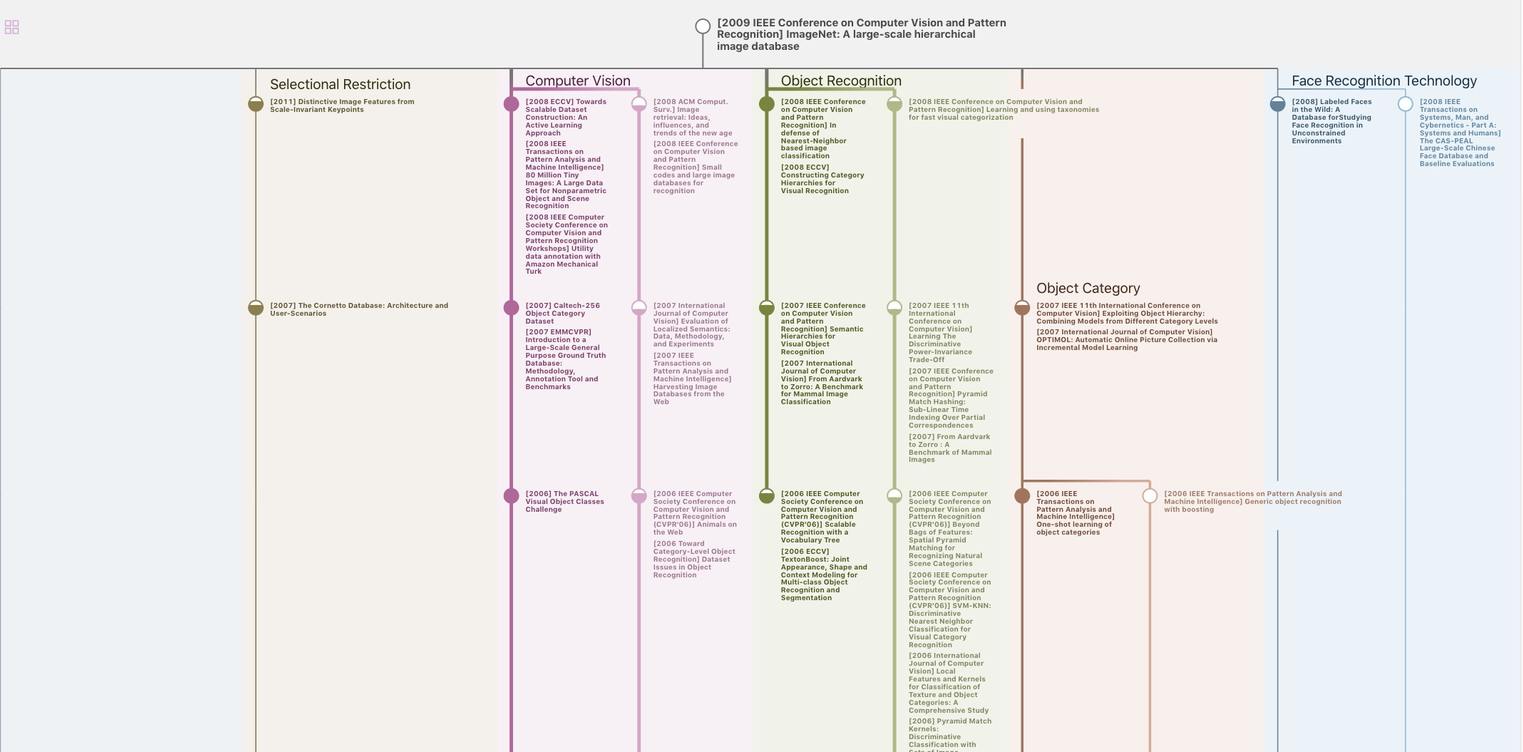
生成溯源树,研究论文发展脉络
Chat Paper
正在生成论文摘要