A Cognitive Stochastic Machine Based on Bayesian Inference: A Behavioral Analysis
2018 IEEE 17th International Conference on Cognitive Informatics & Cognitive Computing (ICCI*CC)(2018)
摘要
Bayesian models and stochastic computing form a promising paradigm for non-conventional, bio-inspired computation architectures. In particular, they are able to handle uncertainty and promise low power consumption. In this paper we study the application of such an architecture, the Sliced Bayesian Machine (SlicedBM) to a real-world problem, Sound Source Localization (SSL) for robots. We present an analysis of the quality of results and of computing time according to several parameters: sensor precision, result threshold, internal word length. Furthermore, we show that sensor data precision does not heavily influence the computation. On the opposite, the precision of the probability values plays an important role on result quality. This parameter also determines the circuit size. We also show that the higher the re-sampling threshold (RT), the better the distribution computed by the machine. Our results make it possible to choose optimal design parameters for a circuit along several trade-offs, and according to a given sensor fusion application.
更多查看译文
关键词
Bayesian models,stochastic computing form,bio-inspired computation architectures,low power consumption,Sliced Bayesian Machine,SlicedBM,real-world problem,Sound Source Localization,SSL,sensor precision,internal word length,sensor data precision,probability values,result quality,optimal design parameters,cognitive stochastic Machine,Bayesian inference,behavioral analysis,re-sampling threshold,sensor fusion application
AI 理解论文
溯源树
样例
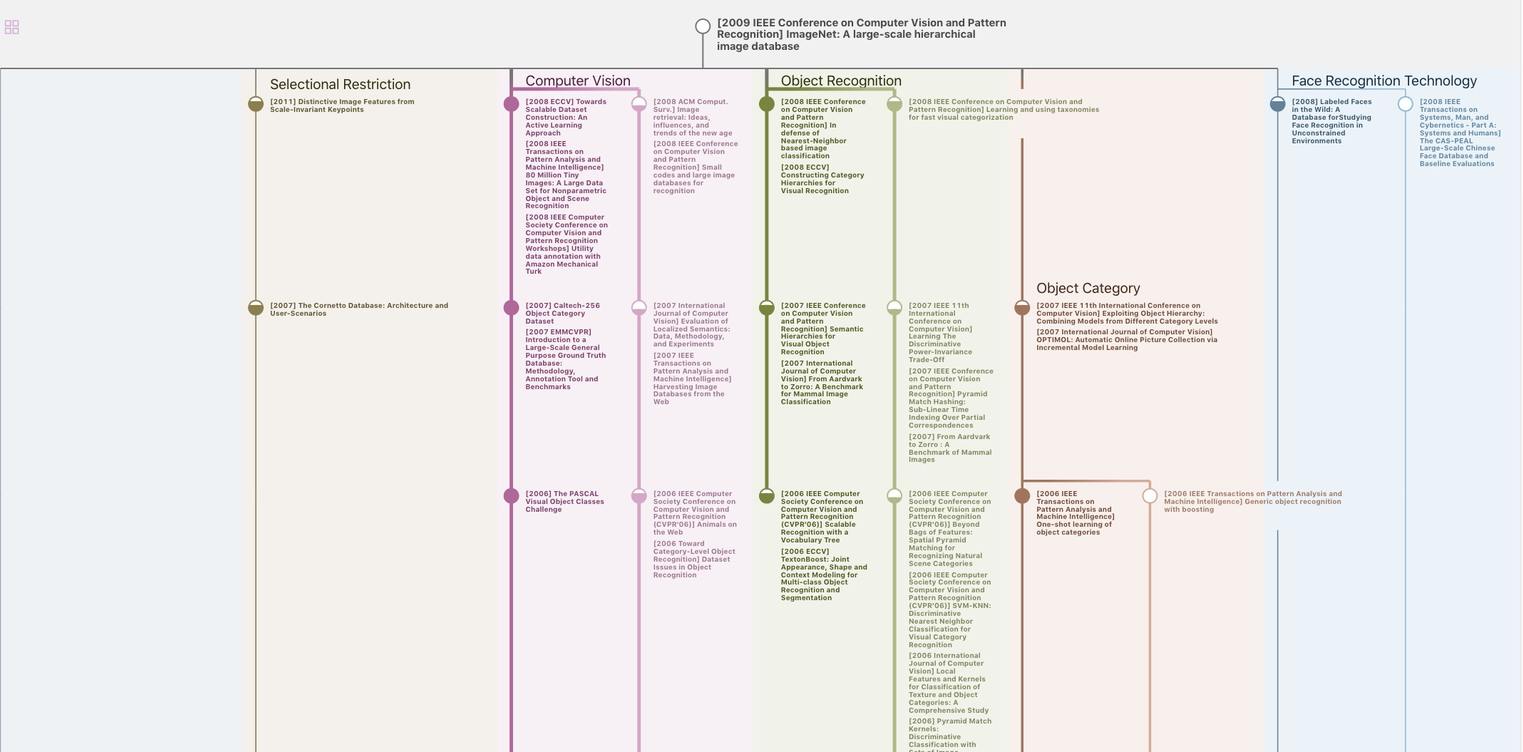
生成溯源树,研究论文发展脉络
Chat Paper
正在生成论文摘要