Modeling Consumer Buying Decision for Recommendation Based on Multi-Task Deep Learning.
CIKM(2018)
摘要
Although marketing researchers and sociologists have recognized the importance of buying decision process and its significant influence on consumer's purchasing behaviors, existing recommender systems do not explicitly model the consumer buying decision process or capture the sequential regularities of what happens before and after each purchase. In this paper, we try to bridge the gap and improve recommendation systems by explicitly modeling consumer buying decision process and corresponding stages. In particular, we propose a multi-task learning model with long short-term memory networks (LSTM) to learn consumer buying decision process. It maps items, users, product categories, and the behavior sequences into real valued vectors, with which the probability of purchasing a product can be estimated. In this way, the model can capture user intentions and preferences, predicts the conversion rate of each candidate product, and makes recommendations accordingly. Experiments on real world data demonstrate the effectiveness of the proposed approach.
更多查看译文
关键词
User Modeling, Recommendation, Multi-Task Learning
AI 理解论文
溯源树
样例
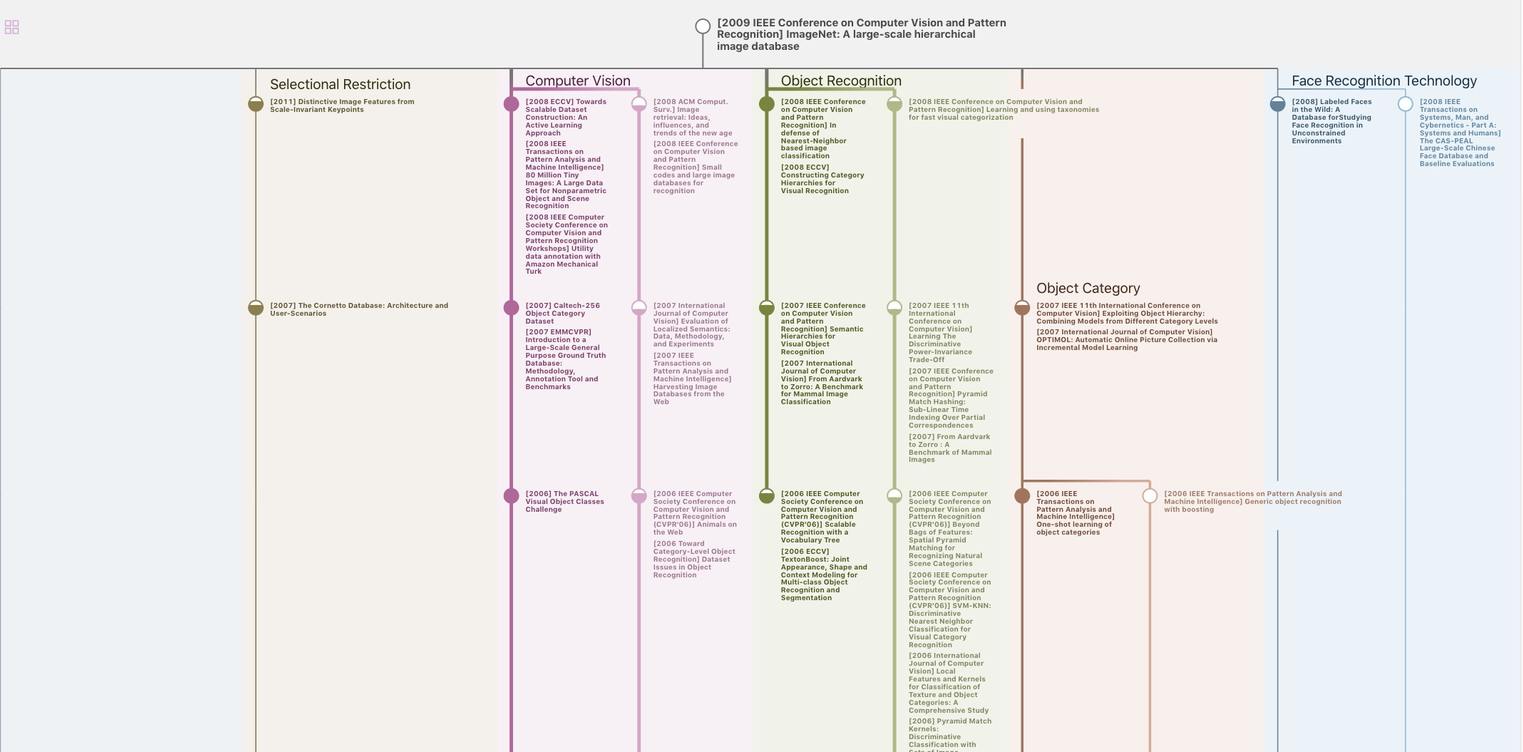
生成溯源树,研究论文发展脉络
Chat Paper
正在生成论文摘要