SDCNet: Video Prediction Using Spatially-Displaced Convolution
COMPUTER VISION - ECCV 2018, PT VII(2018)
摘要
We present an approach for high-resolution video frame prediction by conditioning on both past frames and past optical flows. Previous approaches rely on resampling past frames, guided by a learned future optical flow, or on direct generation of pixels. Resampling based on flow is insufficient because it cannot deal with disocclusions. Generative models currently lead to blurry results. Recent approaches synthesis a pixel by convolving input patches with a predicted kernel. However, their memory requirement increases with kernel size. Here, we spatially-displaced convolution (SDC) module for video frame prediction. We learn a motion vector and a kernel for each pixel and synthesize a pixel by applying the kernel at a displaced location in the source image, defined by the predicted motion vector. Our approach inherits the merits of both vector-based and kernel-based approaches, while ameliorating their respective disadvantages. We train our model on 428K unlabelled 1080p video game frames. Our approach produces state-of-the-art results, achieving an SSIM score of 0.904 on high-definition YouTube-8M videos, 0.918 on Caltech Pedestrian videos. Our model handles large motion effectively and synthesizes crisp frames with consistent motion.
更多查看译文
关键词
3D CNN, Sampling kernel, Optical flow, Frame prediction
AI 理解论文
溯源树
样例
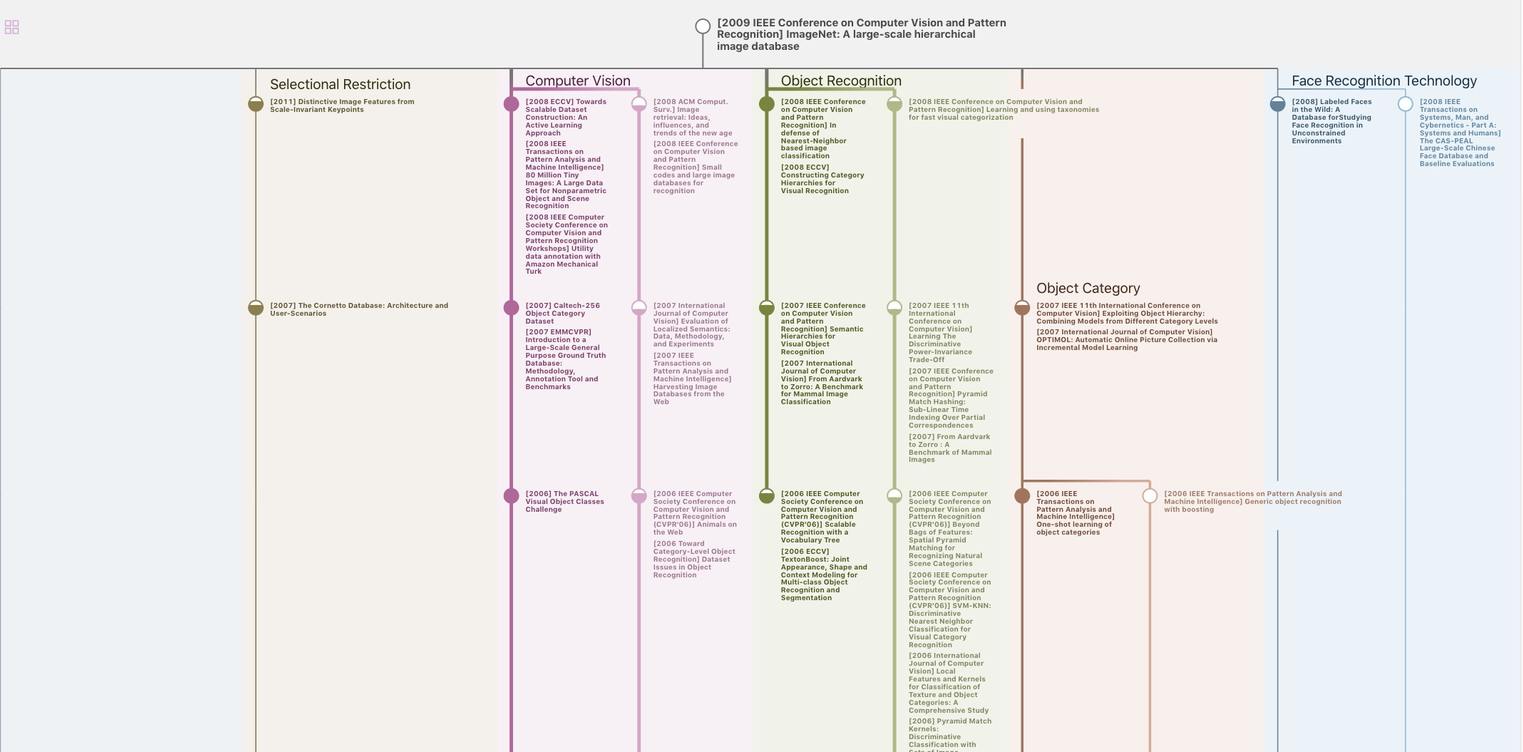
生成溯源树,研究论文发展脉络
Chat Paper
正在生成论文摘要