Transferable Adversarial Perturbations
COMPUTER VISION - ECCV 2018, PT XIV(2018)
摘要
State-of-the-art deep neural network classifiers are highly vulnerable to adversarial examples which are designed to mislead classifiers with a very small perturbation. However, the performance of black-box attacks (without knowledge of the model parameters) against deployed models always degrades significantly. In this paper, We propose a novel way of perturbations for adversarial examples to enable black-box transfer. We first show that maximizing distance between natural images and their adversarial examples in the intermediate feature maps can improve both white-box attacks (with knowledge of the model parameters) and black-box attacks. We also show that smooth regularization on adversarial perturbations enables transferring across models. Extensive experimental results show that our approach outperforms state-of-the-art methods both in white-box and black-box attacks.
更多查看译文
关键词
Adversarial perturbations, Transferability, Black-box attacks
AI 理解论文
溯源树
样例
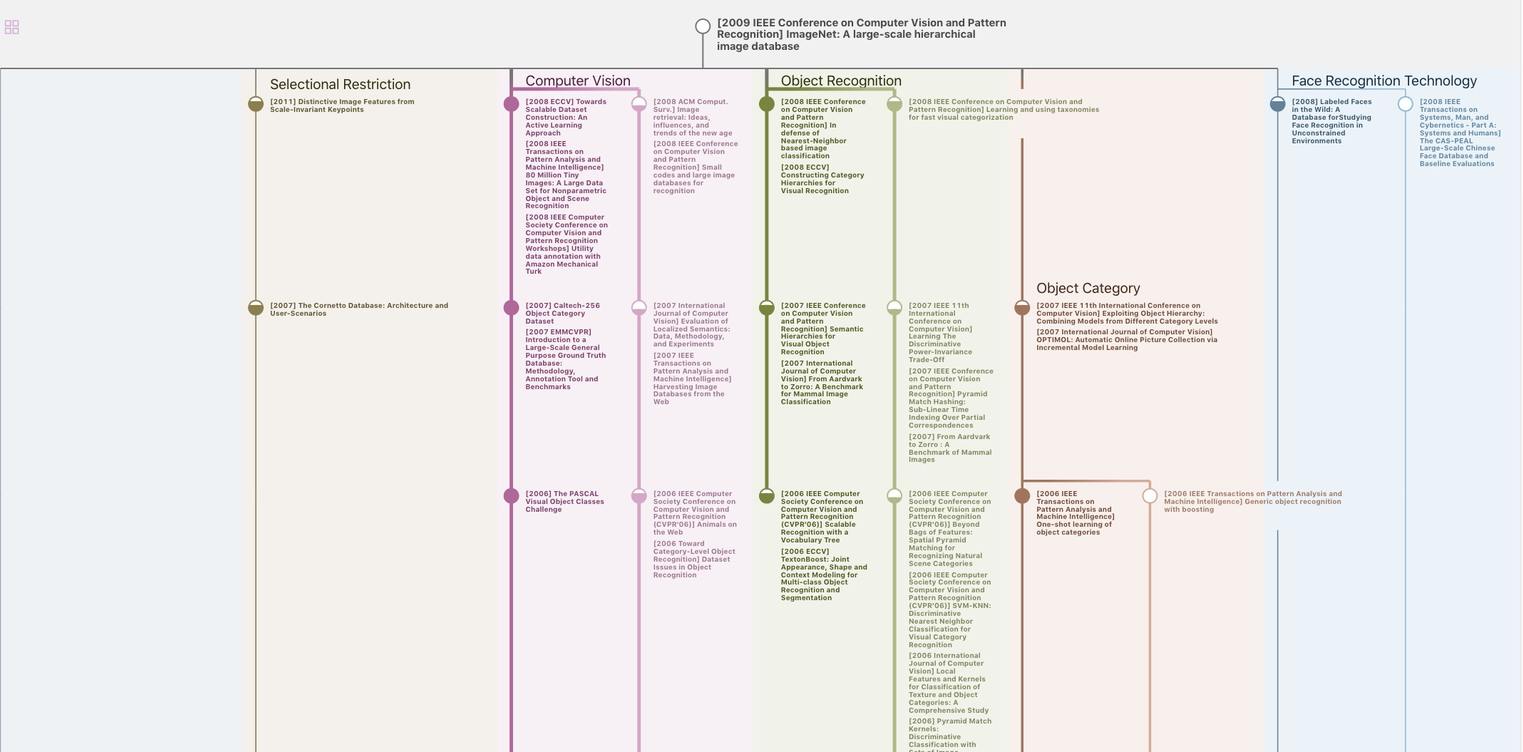
生成溯源树,研究论文发展脉络
Chat Paper
正在生成论文摘要