Type-2 Diabetes Mellitus Diagnosis From Time Series Clinical Data Using Deep Learning Models
ARTIFICIAL NEURAL NETWORKS AND MACHINE LEARNING - ICANN 2018, PT III(2018)
摘要
Clinical data is usually observed and recorded at irregular intervals and includes: evaluations, treatments, vital sign and lab test results. These provide an invaluable source of information to help diagnose and understand medical conditions. In this work, we introduce the largest patient records dataset in diabetes research: King Abdullah International Research Centre Diabetes (KAIMRCD) which includes over 14k patient data. KAIMRCD contains detailed information about the patient's visit and have been labelled against T2DM by clinicians. The data is processed as time series and then investigated using temporal predictive Deep Learning models with the goal of diagnosing Type 2 Diabetes Mellitus (T2DM). Long Short-Term Memory (LSTM) and Gated-Recurrent Unit (GRU) are trained on KAIMRCD and are demonstrated here to outperform classical machine learning approaches in the literature with over 97% accuracy.
更多查看译文
关键词
Type 2 diabetes mellitus, Deep learning, Long short-term memory, Gated-recurrent unit, King abdullah international research centre diabetes
AI 理解论文
溯源树
样例
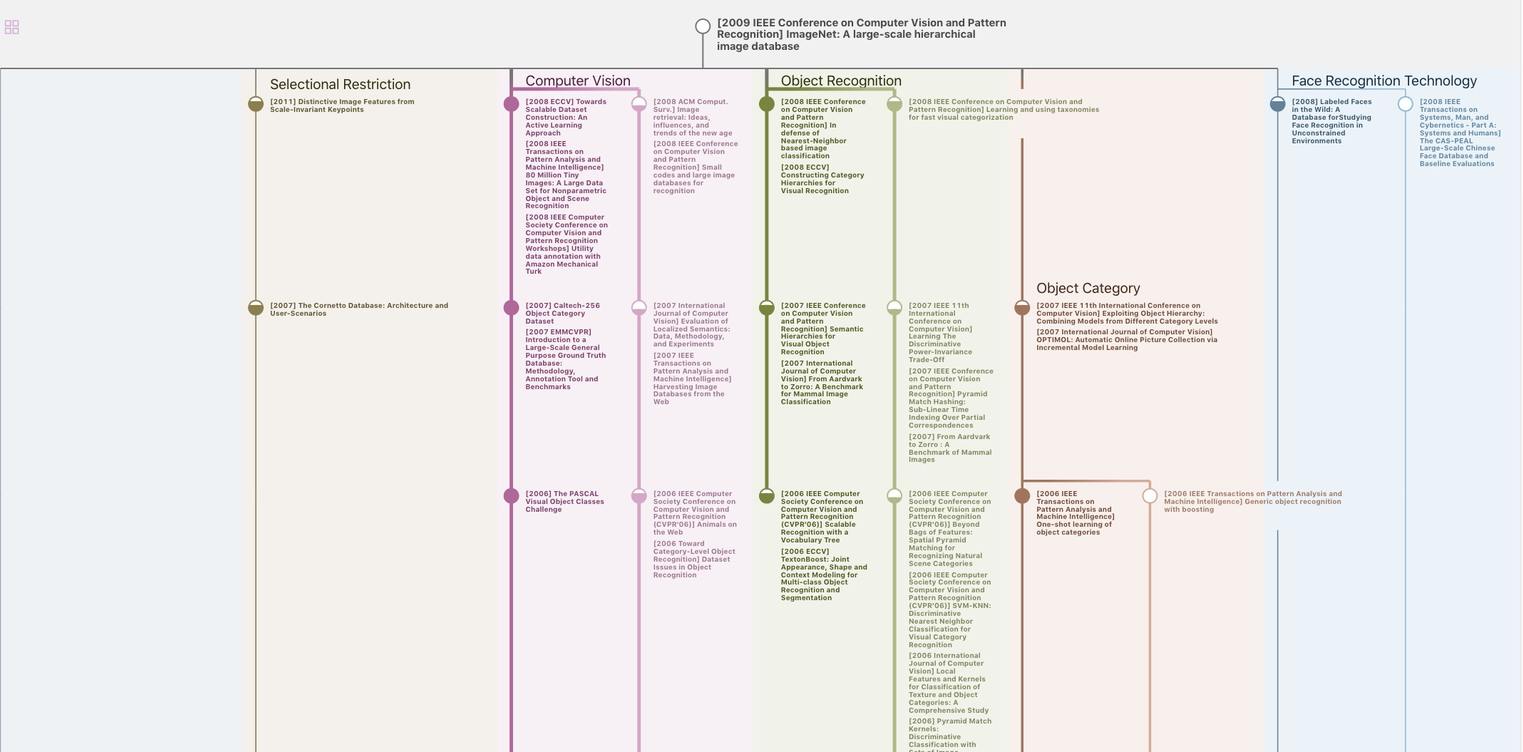
生成溯源树,研究论文发展脉络
Chat Paper
正在生成论文摘要