Design-Space Exploration Of Pareto-Optimal Architectures For Deep Learning With Dvfs
2018 IEEE INTERNATIONAL SYMPOSIUM ON CIRCUITS AND SYSTEMS (ISCAS)(2018)
摘要
Specialized computing engines are required to accelerate the execution of Deep Learning (DL) algorithms in an energy-efficient way. To adapt the processing throughput of these accelerators to the workload requirements while saving power, Dynamic Voltage and Frequency Scaling (DVFS) seems the natural solution. However, DL workloads need to frequently access the off-chip memory, which tends to make the performance of these accelerators memory-bound rather than computation-bound, hence reducing the effectiveness of DVFS. In this work we use a performance-power analytical model fitted on a parametrized implementation of a DL accelerator in a 28-nm FDSOI technology to explore a large design space and to obtain the Pareto points that maximize the effectiveness of DVFS in the sub-space of throughput and energy efficiency. In our model we consider the impact on performance and power of the off-chip memory using real data of a commercial low-power DRAM.
更多查看译文
关键词
deep learning algorithms,dynamic voltage,frequency scaling,FDSOI technology,low-power DRAM,energy efficiency,Pareto points,DL accelerator,performance-power analytical model,accelerators memory-bound,off-chip memory,DL workloads,specialized computing engines,DVFS,Pareto-optimal architectures,design-space exploration,size 28 nm,Si
AI 理解论文
溯源树
样例
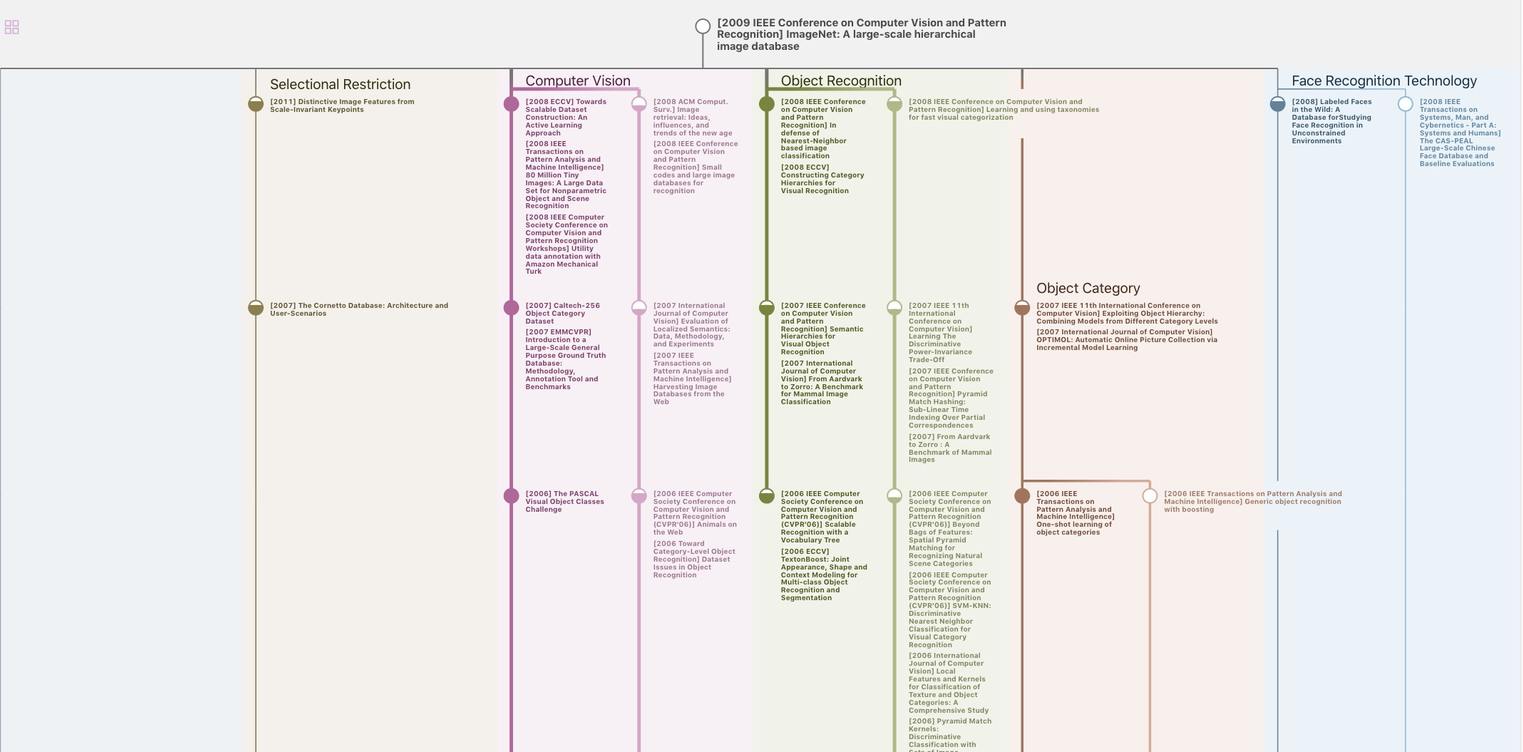
生成溯源树,研究论文发展脉络
Chat Paper
正在生成论文摘要