Sticker: A 0.41-62.1 TOPS/W 8Bit Neural Network Processor with Multi-Sparsity Compatible Convolution Arrays and Online Tuning Acceleration for Fully Connected Layers
2018 IEEE Symposium on VLSI Circuits(2018)
摘要
Neural Networks (NNs) have emerged as a fundamental technology for machine learning. The sparsity of weight and activation in NNs varies widely from 5%-90% and can potentially lower computation requirements. However, existing designs lack a universal solution to efficiently handle different sparsity in various layers and neural networks. This work, named STICKER, first systematically explores NN sparsity for inference and online tuning operations. Its major contributions are: 1) autonomous NN sparsity detector that switches the processor modes; 2) Multi-sparsity compatible Convolution (CONV) PE arrays that contain a multi-mode memory supporting different sparsity, and the set-associative PEs supporting both dense and sparse operations and reducing 92% memory area compared with previous hash memory banks; 3) Online tuning PE for sparse FCs that achieves 32.5x speedup compared with conventional CPU, using quantization center-based weight updating and Compressed Sparse Column (CSC) based back propagations. Peak energy efficiency of the 65nm STICKER chip is up to 62.1 TOPS/W at 8bit data length.
更多查看译文
关键词
quantization center-based weight updating,Online tuning acceleration,fully connected layers,machine learning,NN sparsity,processor modes,multimode memory,dense operations,sparse operations,neural network processor,computation requirements,Online tuning operations,multisparsity compatible convolution PE arrays,CONV,hash memory banks,STICKER chip,compressed sparse column,CSC,back propagations,Online tuning PE,conventional CPU,peak energy efficiency
AI 理解论文
溯源树
样例
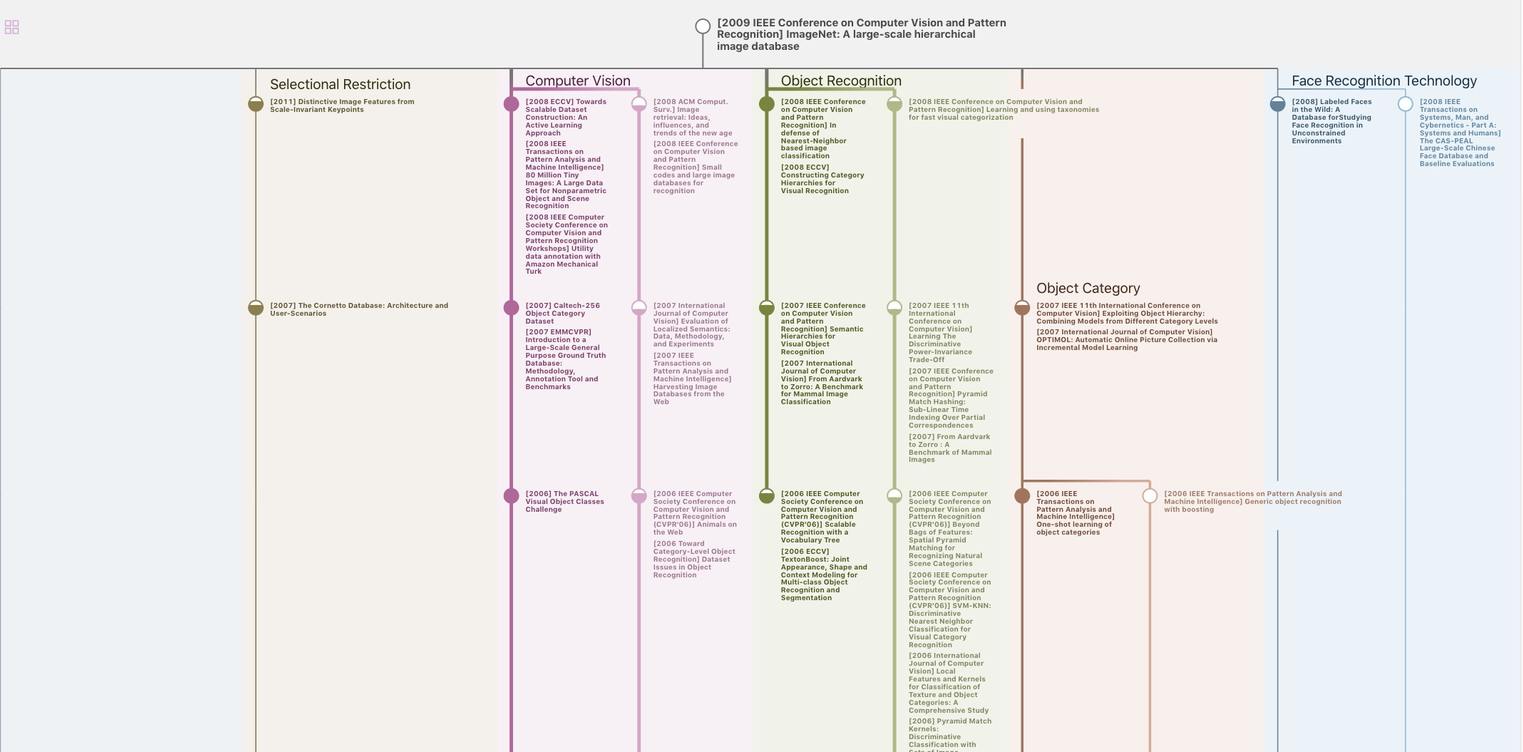
生成溯源树,研究论文发展脉络
Chat Paper
正在生成论文摘要