Unbiased Learning to Rank: Theory and Practice
CIKM'18: PROCEEDINGS OF THE 27TH ACM INTERNATIONAL CONFERENCE ON INFORMATION AND KNOWLEDGE MANAGEMENT(2018)
摘要
Implicit user feedback (such as clicks and dwell time) is an important source of data for modern search engines. While heavily biased [10, 11, 13, 27], it is cheap to collect and particularly useful for user-centric retrieval applications such as search ranking and query recommendation. Understanding the bias inherent in current systems and designing learning to rank algorithms that can effectively learn from implicit user feedback without bias is an important research direction that can significantly improve the quality of modern search engines. To develop such an unbiased learning-to-rank (ULTR) system, previous studies have focused on constructing probabilistic graphical models (e.g., click models) with user behavior hypothesis to extract and train ranking systems with unbiased relevance signals. Recently, a novel counterfactual learning framework that estimates and adopts examination propensity for unbiased learning to rank has attracted much attention, both in academia and industry. Despite its popularity, there is no systematic comparison and analysis of the unbiased learning-to-rank frameworks based on graphical models and counterfactual learning. In this tutorial, we provide an overview of the fundamental mechanism and algorithms for unbiased learning to rank. We describe and analyze the theory behind each learning framework, and give detailed instructions on how to conduct unbiased learning to rank in practice.
更多查看译文
关键词
user bias, click model, counterfactual learning, unbiased learning to rank
AI 理解论文
溯源树
样例
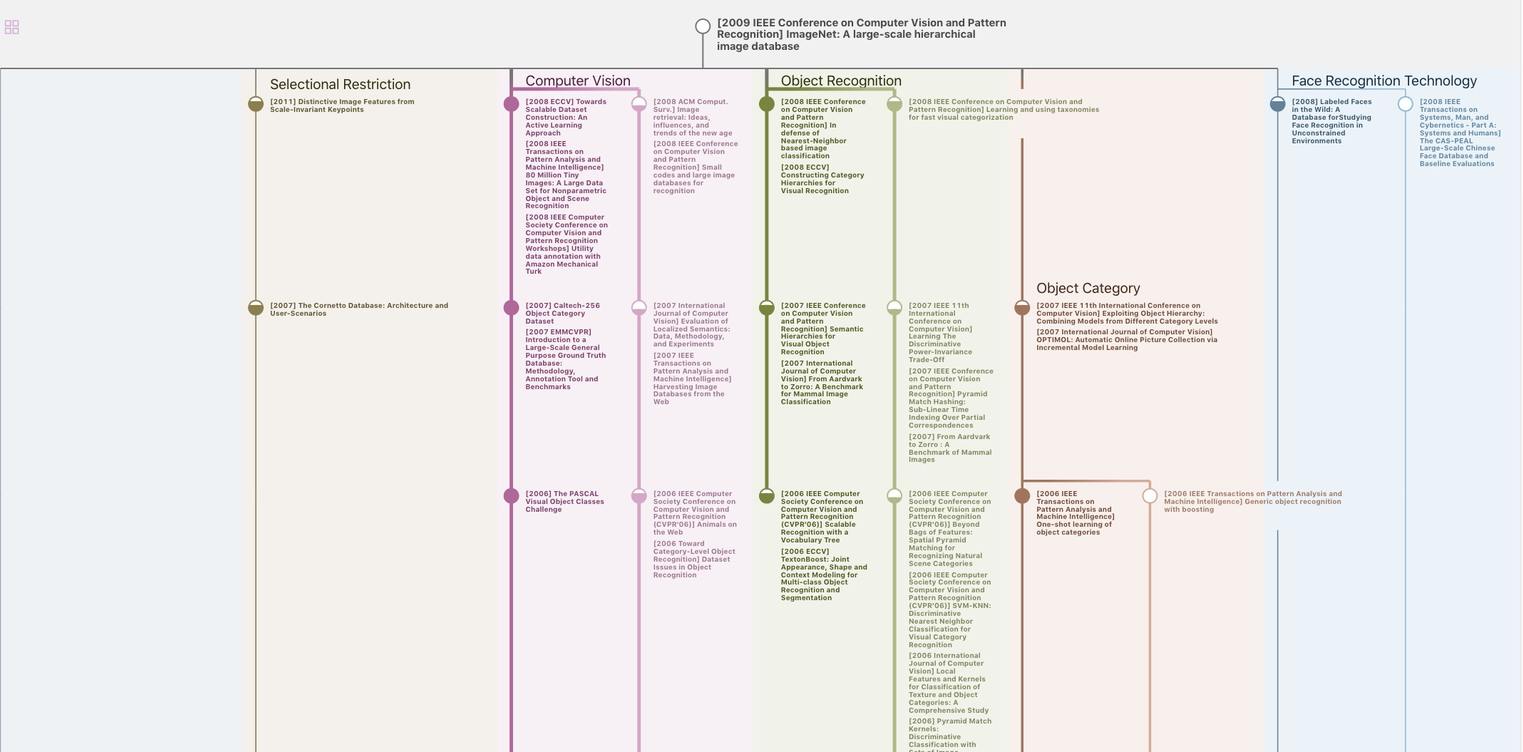
生成溯源树,研究论文发展脉络
Chat Paper
正在生成论文摘要