Open Set Recognition By Regularising Classifier With Fake Data Generated By Generative Adversarial Networks
2018 IEEE INTERNATIONAL CONFERENCE ON ACOUSTICS, SPEECH AND SIGNAL PROCESSING (ICASSP)(2018)
摘要
We present a new method to generate fake data in unknown classes in generative adversarial networks (GANs) framework. The generator in GANs is trained to generate somewhat similar to data in known classes but the different one by modelling noisy distribution on feature space of a classifier using proposed marginal denoising autoencoder. The generated data are treated as fake instances in unknown classes and given to the classifier to make it be robust to the real unknown classes. Our results show that synthetic data can act as fake unknown classes and keep down the certainty of the classifier on real unknown classes meanwhile the classification capability of known classes is not degenerated, even improved.
更多查看译文
关键词
Generative adversarial networks, Denoising autoencoder, Open set recognition, Feature matching
AI 理解论文
溯源树
样例
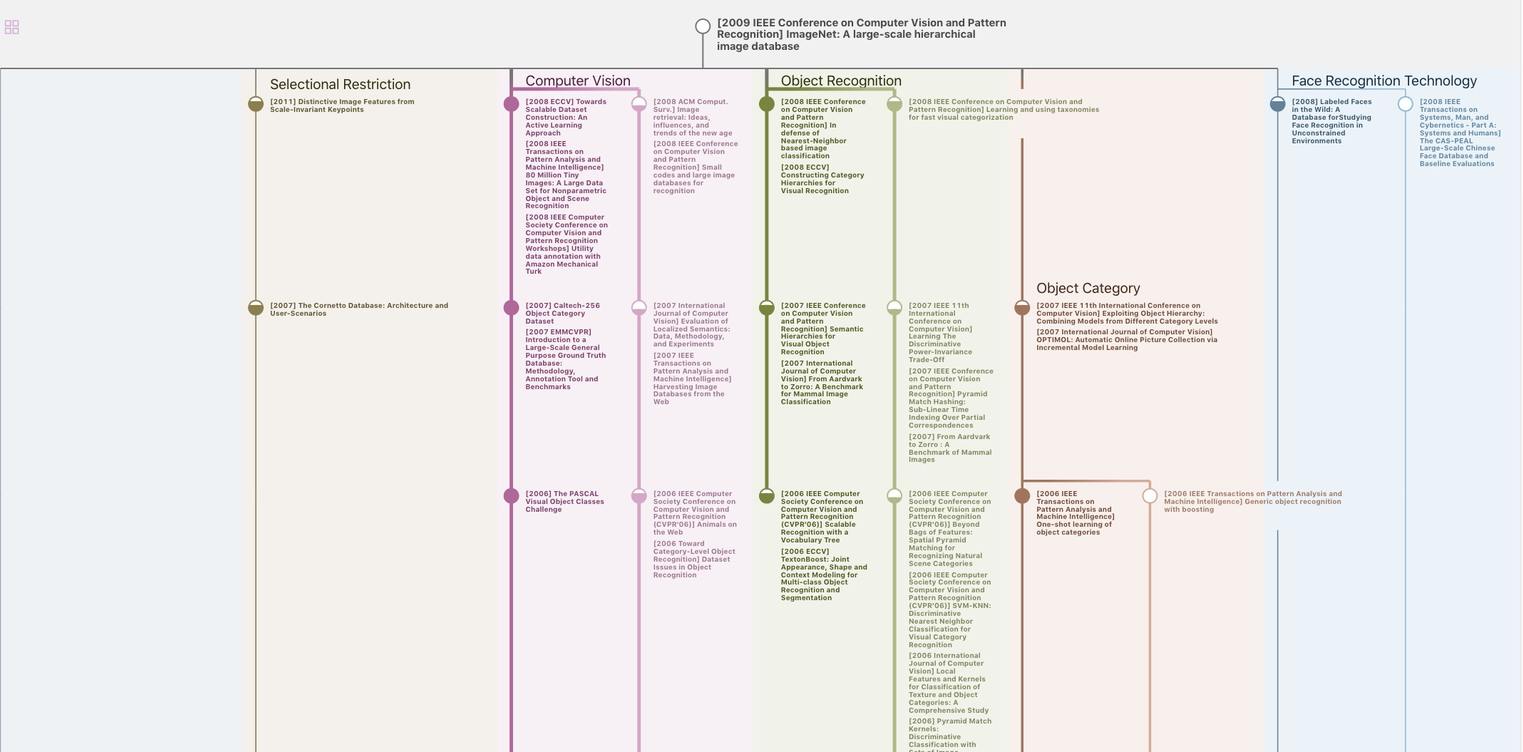
生成溯源树,研究论文发展脉络
Chat Paper
正在生成论文摘要