Non-euclidean vector product for neural networks
2018 IEEE INTERNATIONAL CONFERENCE ON ACOUSTICS, SPEECH AND SIGNAL PROCESSING (ICASSP)(2018)
摘要
We present a non-Euclidean vector product for artificial neural networks. The vector product operator does not require any multiplications while providing correlation information between two vectors. Ordinary neurons require inner product of two vectors. We propose a class of neural networks with the universal approximation property over the space of Lebesgue integrable functions based on the proposed non-Euclidean vector product. In this new network, the "product" of two real numbers is defined as the sum of their absolute values, with the sign determined by the sign of the product of the numbers. This "product' is used to construct a vector product in R-N. The vector product induces the l(1) norm. The additive neural network successfully solves the XOR problem. Experiments on MNIST and CIFAR datasets show that the classification performance of the proposed additive neural network is comparable to the corresponding multi-layer perceptron and convolutional neural networks.
更多查看译文
关键词
non-Euclidean operator,additive neural networks,multiplication-free operator
AI 理解论文
溯源树
样例
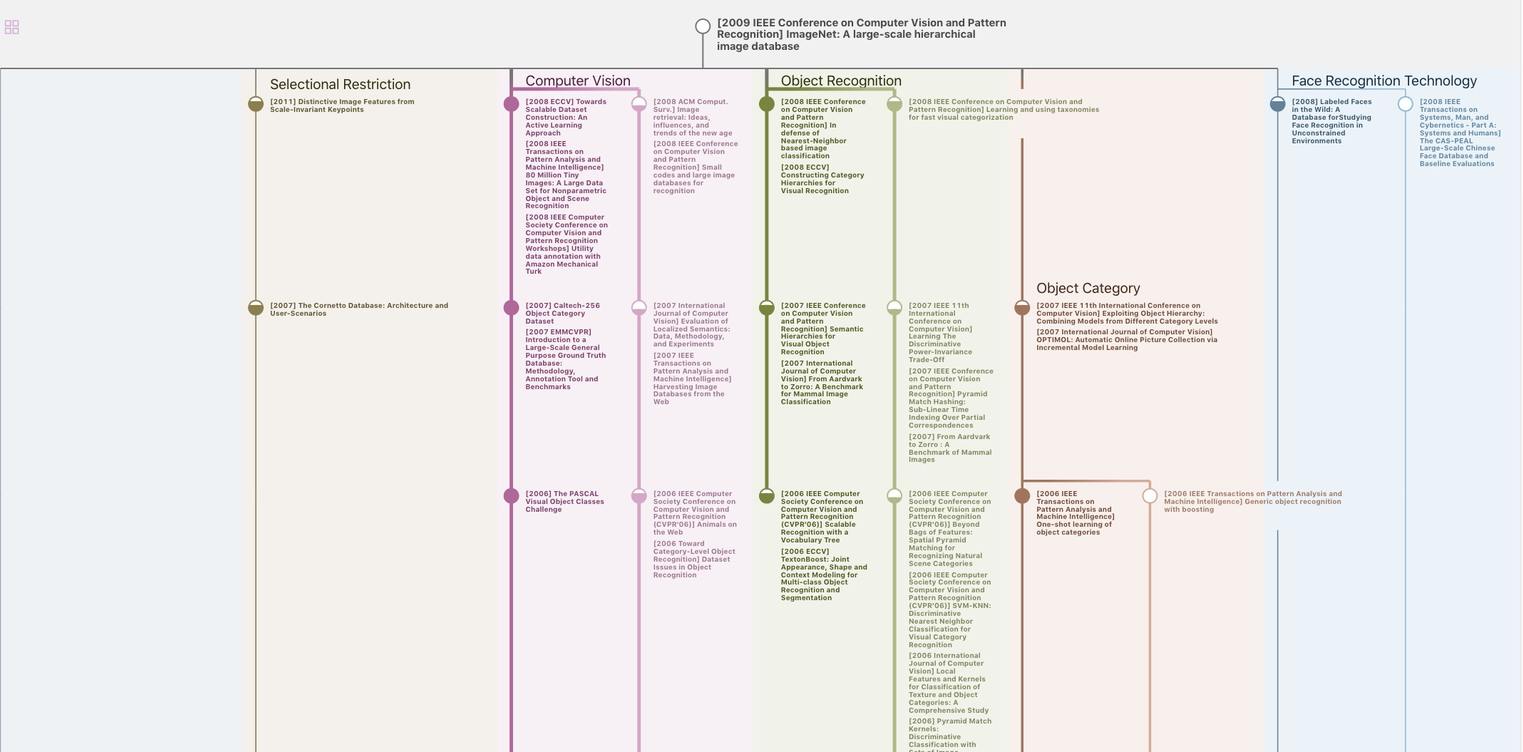
生成溯源树,研究论文发展脉络
Chat Paper
正在生成论文摘要