Towards Scalable Information-Seeking Multi-Domain Dialogue
2018 IEEE INTERNATIONAL CONFERENCE ON ACOUSTICS, SPEECH AND SIGNAL PROCESSING (ICASSP)(2018)
摘要
Multi-domain dialogue systems face challenges such as scaling algorithms to handle large ontologies, or transferring trained policy models to unseen domains. We attempt to address these challenges by proposing a dialogue management architecture that has an abstracted view of the world but yet is able to focus on relevant parts of the ontology at runtime. Specifically, we train a sub-domain identifier neural network that learns which features are relevant to the current turn and the immediate future, thus filtering out irrelevant information from the ontology and consequently the belief space at each dialogue turn. We then train a policy network that needs: a) to adapt to the sub-domain identifier's output; and b) to learn what information will carry over from previous turns and when it needs to be updated. We evaluate our method on a large information-seeking ontology that contains latent sub-domains. Our results in simulation and a small human trial show that the sub-domain identifier is able to generalise to unseen domains and achieve performance on par with a multi-domain dialogue manager where each sub-domain is carefully defined (golden standard).
更多查看译文
关键词
Spoken Dialogue Management, Multiple Domains, Dialogue Policy, Policy Network
AI 理解论文
溯源树
样例
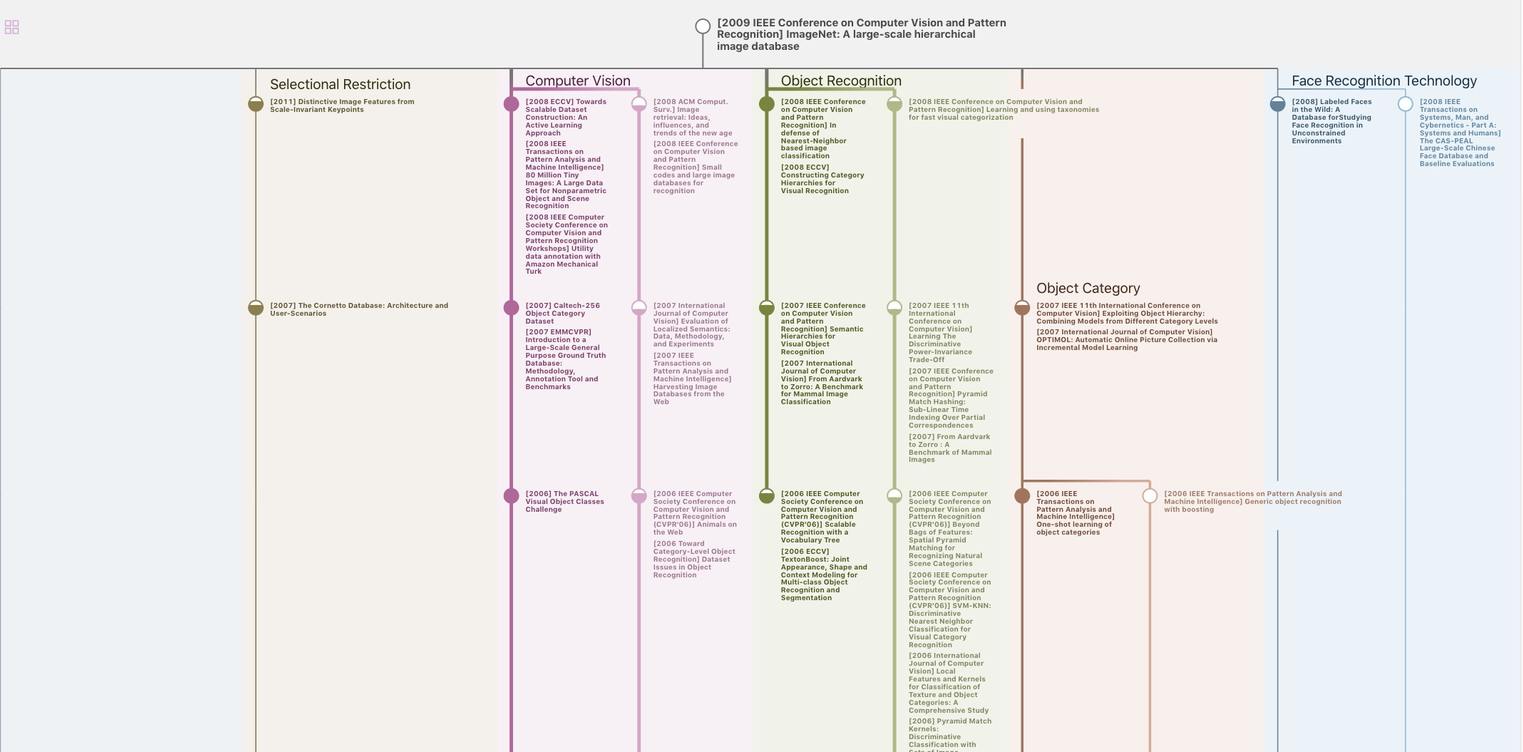
生成溯源树,研究论文发展脉络
Chat Paper
正在生成论文摘要