Segmental Encoder-Decoder Models For Large Vocabulary Automatic Speech Recognition
19TH ANNUAL CONFERENCE OF THE INTERNATIONAL SPEECH COMMUNICATION ASSOCIATION (INTERSPEECH 2018), VOLS 1-6: SPEECH RESEARCH FOR EMERGING MARKETS IN MULTILINGUAL SOCIETIES(2018)
摘要
It has been known for a long time that the classic Hidden-Markov-Model (HMM) derivation for speech recognition contains assumptions such as independence of observation vectors and weak duration modeling that are practical but unrealistic. When using the hybrid approach this is amplified by trying to fit a discriminative model into a generative one. Hidden Conditional Random Fields (CRFs) and segmental models (e.g. Semi-Markov CRFs / Segmental CRFs) have been proposed as an alternative, but for a long time have failed to get traction until recently. In this paper we explore different length modeling approaches for segmental models, their relation to attention-based systems. Furthermore we show experimental results on a handwriting recognition task and to the best of our knowledge the first reported results on the Switchboard 300h speech recognition corpus using this approach.
更多查看译文
关键词
automatic speech recognition (ASR), (hidden) conditional random fields, segmental models, encoder-decoder, attention mechanism
AI 理解论文
溯源树
样例
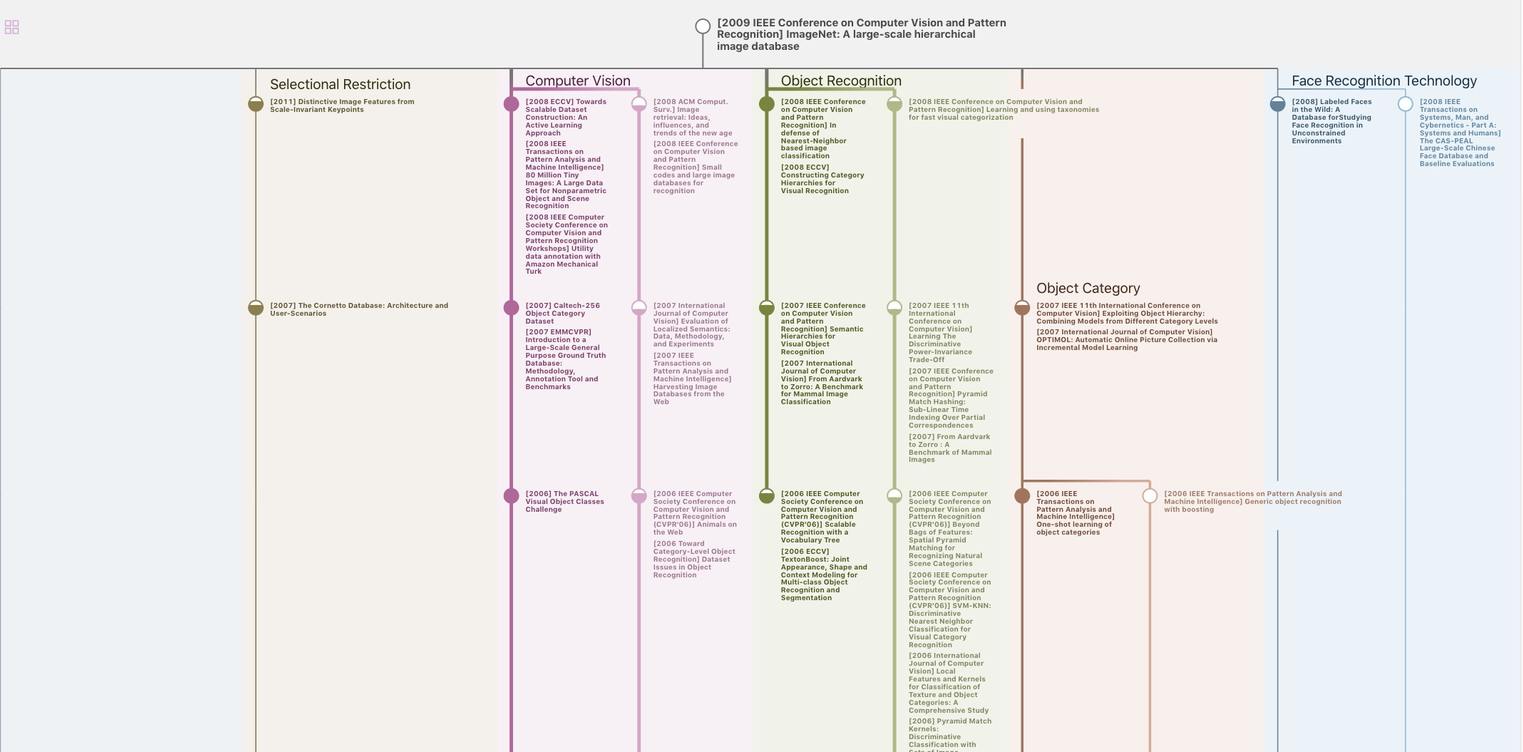
生成溯源树,研究论文发展脉络
Chat Paper
正在生成论文摘要