Identifying Interactions for Information Fusion System Design Using Machine Learning Techniques.
FUSION(2018)
摘要
An Information Fusion System (IFS) is a complex system consisting of various interdependent elements such as sensors, information processors, fusers, sense-makers, and resource managers, etc. These elements are typically designed and evaluated independently, but isolated performance evaluation does not scale to a system-level performance in complex systems. Since the IFS capability results from the collective behavior of these elements, identifying interactions becomes critical for engineering an IFS. In this paper, we investigate machine learning techniques (deep neural networks and general linear models) to provide holistic performance evaluation of the IFS, where the objective is to understand IFS design implications based on variations and interactions of its constituents elements. The challenge for employing machine learning techniques is the availability of a data set to build a predictive performance model of the IFS. We utilize Optimal Design of Experiments to provide the data collection strategy for building the machine learning models, and our results demonstrate that it is imperative to include interactions in the data collection strategy. This attests to the significance of interactions and advises against the independent design and evaluation of IFS constituent elements. Furthermore, we demonstrate how the IFS designers can leverage insights from statistical analysis to exploit interactions between elements to improve IFS design and its performance.
更多查看译文
关键词
Information Fusion System, Performance Evaluation, Machine Learning, Optimal Design of Experiments, Systems Engineering, Complex Systems
AI 理解论文
溯源树
样例
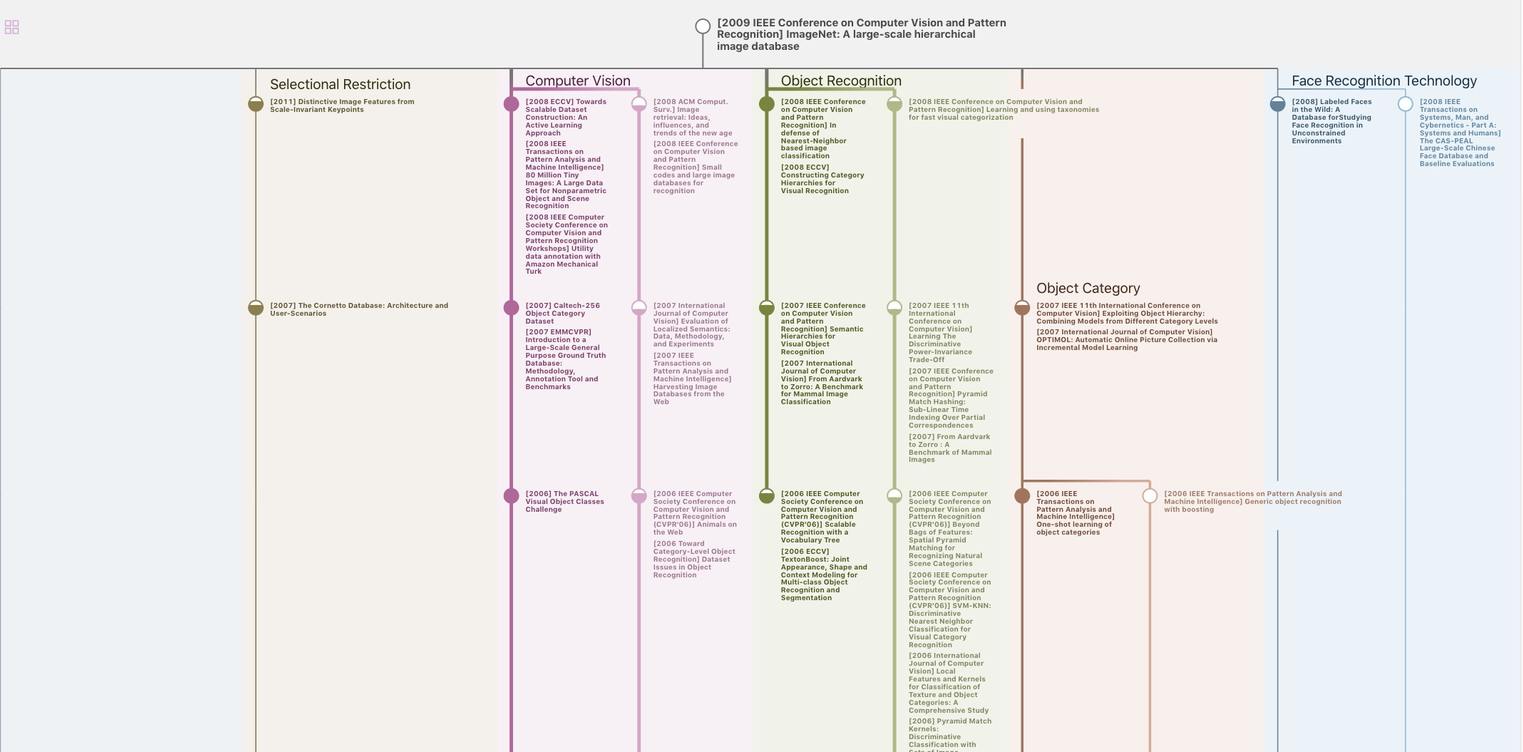
生成溯源树,研究论文发展脉络
Chat Paper
正在生成论文摘要