Improving Energy Efficiency in NFV Clouds with Machine Learning
2018 IEEE 11th International Conference on Cloud Computing (CLOUD)(2018)
摘要
Widespread deployments of Network Function Virtualization (NFV) technology will replace many physical appliances in telecommunication networks with software executed on cloud platforms. Setting compute servers continuously to high-performance operating modes is a common NFV approach for achieving predictable operations. However, this has the effect that large amounts of energy are consumed even when little traffic needs to be forwarded. The Dynamic Voltage-Frequency Scaling (DVFS) technology available in Intel processors is a known option for adapting the power consumption to the workload, but it is not optimized for network traffic processing workloads. We developed a novel control method for DVFS, based observing the ongoing traffic and online predictions using machine learning. Our results show that we can save up to 27% compared to commodity DVFS, even when including the computational overhead of machine learning.
更多查看译文
关键词
energy efficiency,NFV,machine learning,Dynamic Voltage and Frequency Scaling
AI 理解论文
溯源树
样例
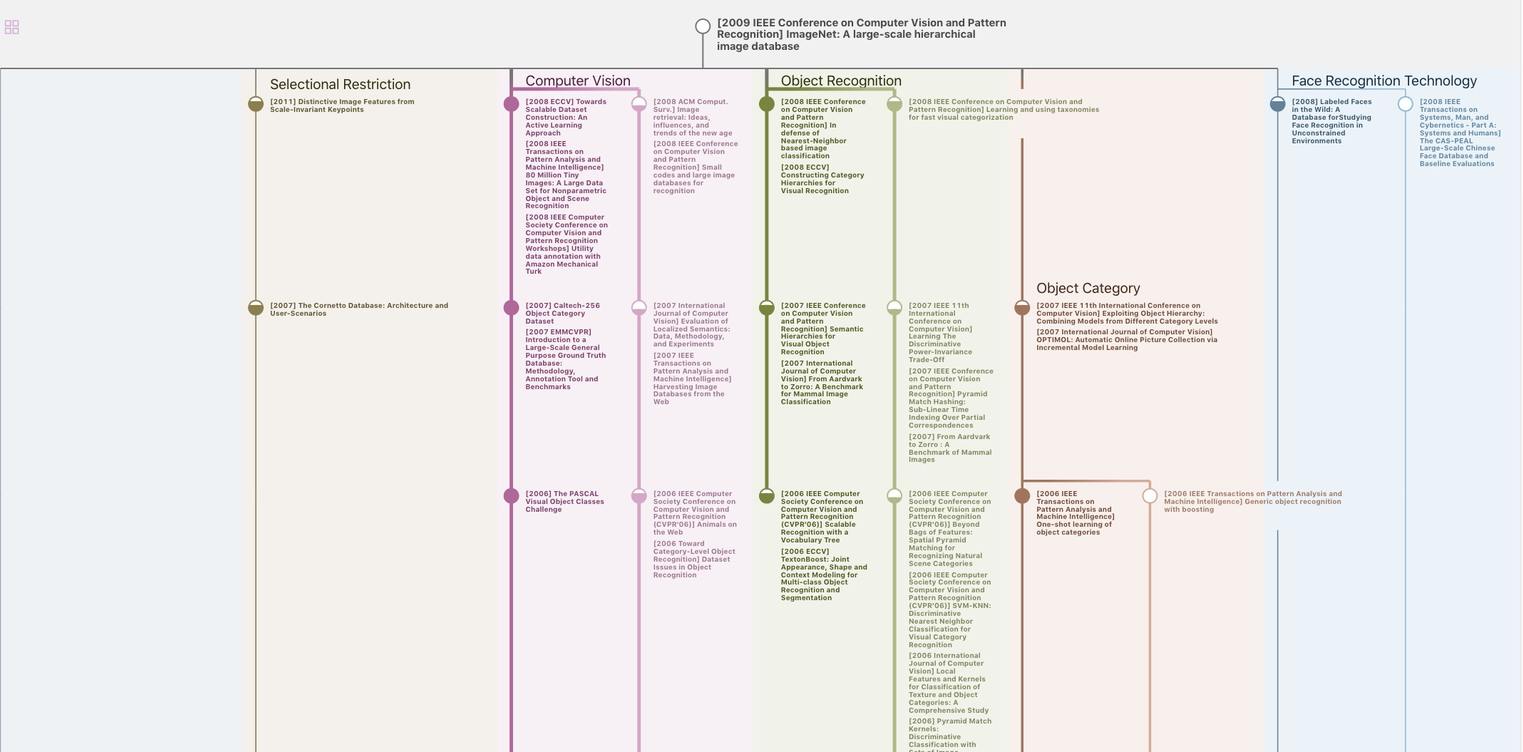
生成溯源树,研究论文发展脉络
Chat Paper
正在生成论文摘要