Multi-View Autoencoder For Image Feature Learning With Structured Nonnegative Low Rank
2018 25TH IEEE INTERNATIONAL CONFERENCE ON IMAGE PROCESSING (ICIP)(2018)
摘要
Multi-view feature learning has garnered much attention recently since many real-world data are comprised of different representations or views. As an important and challenging problem in multi-view feature learning, the key issue is how to explore complementary information and eliminate the inconsistency noise. Motivated by this observation, we propose to encode the multiple feature views to an underlying consensus representation. To enhance the robustness against the noise of disagreement among different feature views and exploit the consistency of the shared structure, we impose the nuclear norm constraint on the consensus representation and enforce the encoded representation to be nonnegative low rank structured. The superior experimental results on three image benchmarks show the effectiveness of our method.
更多查看译文
关键词
multi-view, feature learning, autoencoder, low rank, consensus representation
AI 理解论文
溯源树
样例
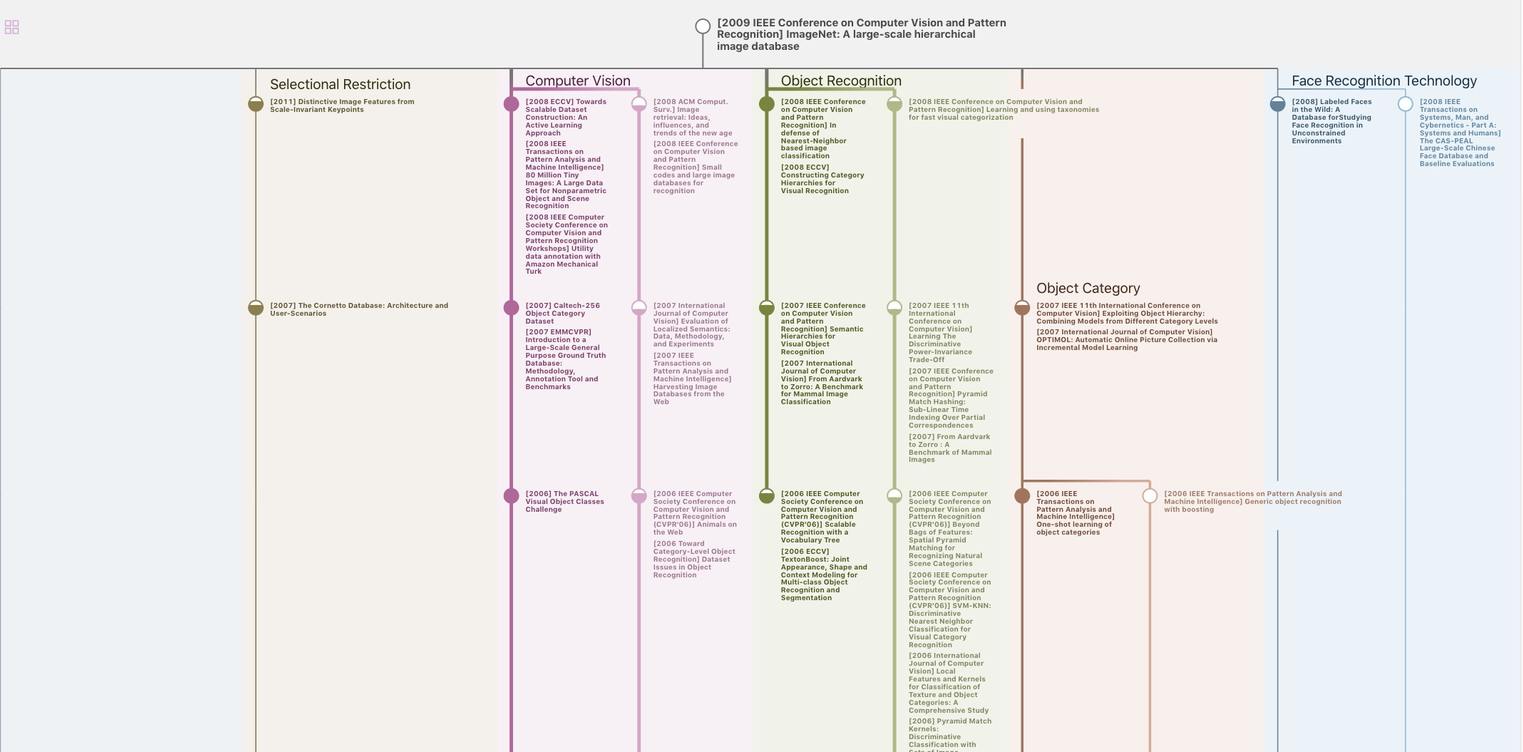
生成溯源树,研究论文发展脉络
Chat Paper
正在生成论文摘要