Detector Monitoring with Artificial Neural Networks at the CMS Experiment at the CERN Large Hadron Collider
Comput. Softw. Big Sci.(2019)
摘要
Reliable data quality monitoring is a key asset in delivering collision data suitable for physics analysis in any modern large-scale high energy physics experiment. This paper focuses on the use of artificial neural networks for supervised and semi-supervised problems related to the identification of anomalies in the data collected by the CMS muon detectors. We use deep neural networks to analyze LHC collision data, represented as images organized geographically. We train a classifier capable of detecting the known anomalous behaviors with unprecedented efficiency and explore the usage of convolutional autoencoders to extend anomaly detection capabilities to unforeseen failure modes. A generalization of this strategy could pave the way to the automation of the data quality assessment process for present and future high energy physics experiments.
更多查看译文
关键词
High energy physics,Large Hadron Collider,Compact Muon Solenoid,Machine learning,Data quality monitoring,Artificial neural networks
AI 理解论文
溯源树
样例
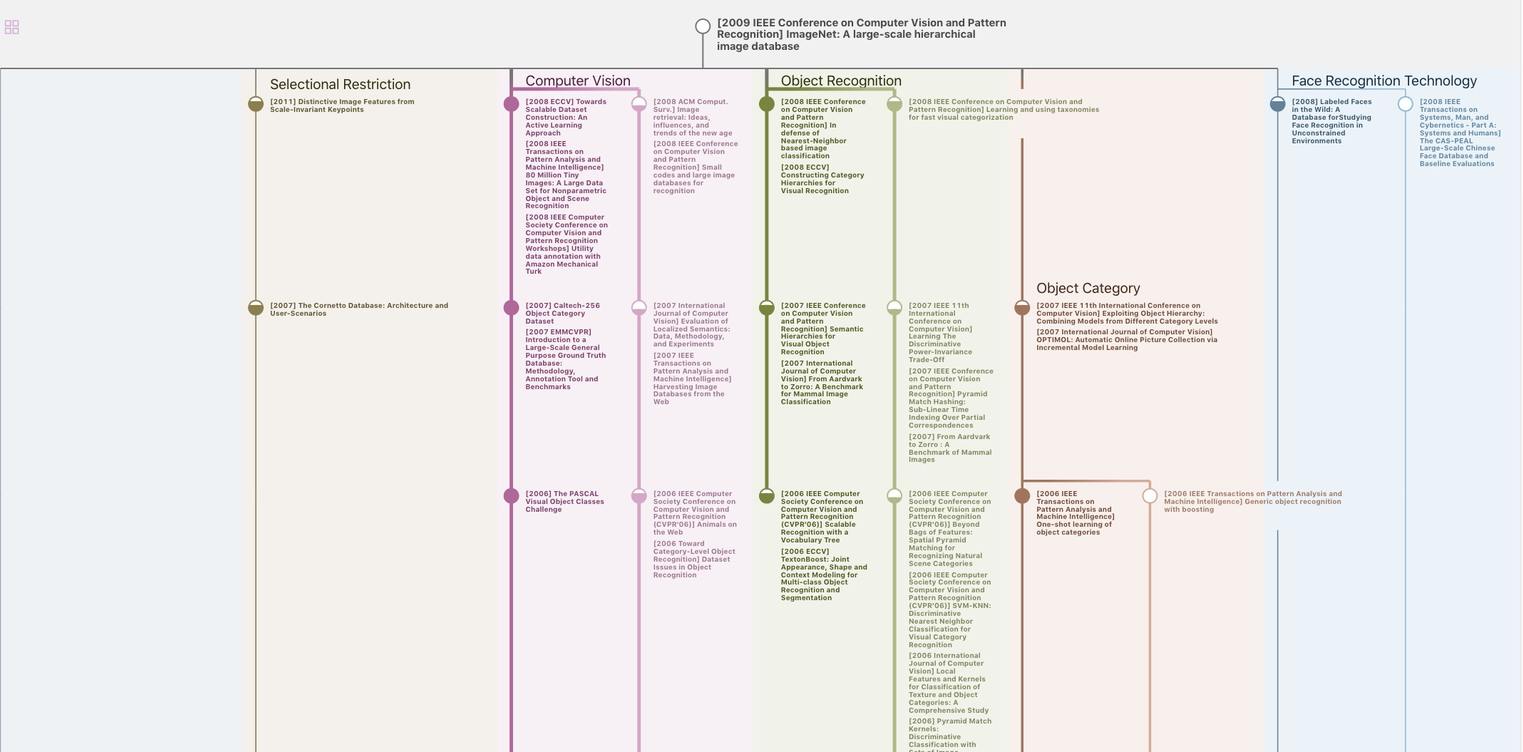
生成溯源树,研究论文发展脉络
Chat Paper
正在生成论文摘要