Multimodal Deep Neural Networks using Both Engineered and Learned Representations for Biodegradability Prediction
arXiv: Learning(2018)
摘要
Deep learning algorithms excel at extracting patterns from raw data, and with large datasets, they have been very successful in computer vision and natural language applications. However, in other domains, large datasets on which to learn representations from may not exist. In this work, we develop a novel multimodal CNN-MLP neural network architecture that utilizes both domain-specific feature engineering as well as learned representations from raw data. We illustrate the effectiveness of such network designs in the chemical sciences, for predicting biodegradability. DeepBioD, a multimodal CNN-MLP network is more accurate than either standalone network designs, and achieves an error classification rate of 0.125 that is 27 state-of-the-art. Thus, our work indicates that combining traditional feature engineering with representation learning can be effective, particularly in situations where labeled data is limited.
更多查看译文
AI 理解论文
溯源树
样例
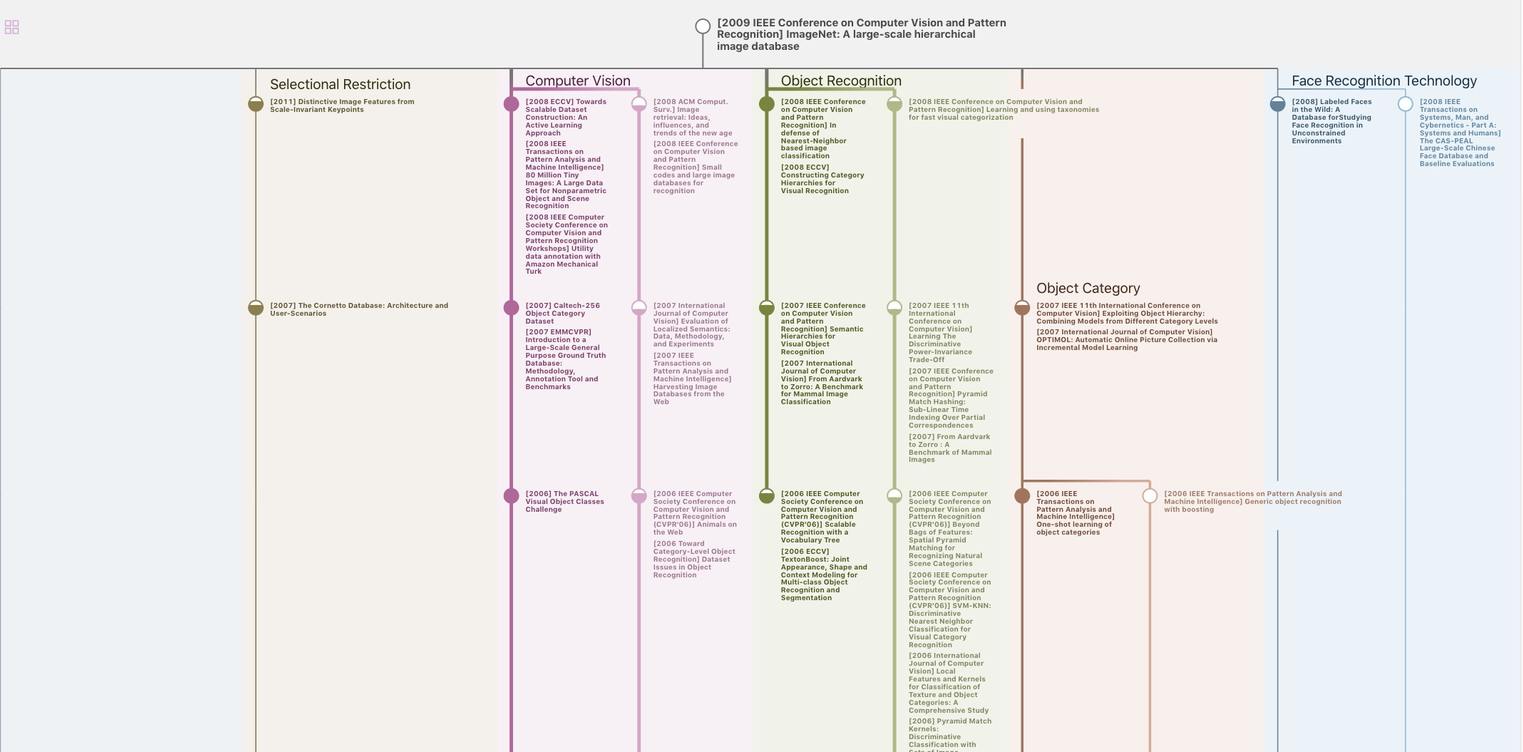
生成溯源树,研究论文发展脉络
Chat Paper
正在生成论文摘要