Vehicle Safety Improvement Through Deep Learning And Mobile Sensing
IEEE NETWORK(2018)
摘要
Information about vehicle safety, such as the driving safety status and the road safety index, is of great importance to protect humans and support safe driving route planning. Despite some research on driving safety analysis, the accuracy and granularity of driving safety assessment are both very limited. Also, the problem of precisely and dynamically predicting road safety throughout a city has not been sufficiently studied and remains open. With the proliferation of sensor-equipped vehicles and smart devices, a huge amount of mobile sensing data provides an opportunity to conduct vehicle safety analysis. In this article, we first discuss mobile sensing data collection in VANETs and then identify two main challengs in vehicle safety analysis in VANETs, i.e., driving safety analysis and road safety analysis. In each issue, we review and classify the state-of-the-art vehicle safety analysis techniques into different categories. For each category, a short description is given followed by a discussion of limitations. In order to improve vehicle safety, we propose a new deep learning framework (DeepRSI) to conduct real-time road safety prediction from the data mining perspective. Specifically, the proposed framework considers the spatio-temporal relationship of vehicle GPS trajectories and external environment factors. The evaluation results demonstrate the advantages of our proposed scheme over other methods by utilizing mobile sensing data collected in VANETs.
更多查看译文
关键词
Sensors,Road safety,Vehicle safety,Accidents,Machine learning
AI 理解论文
溯源树
样例
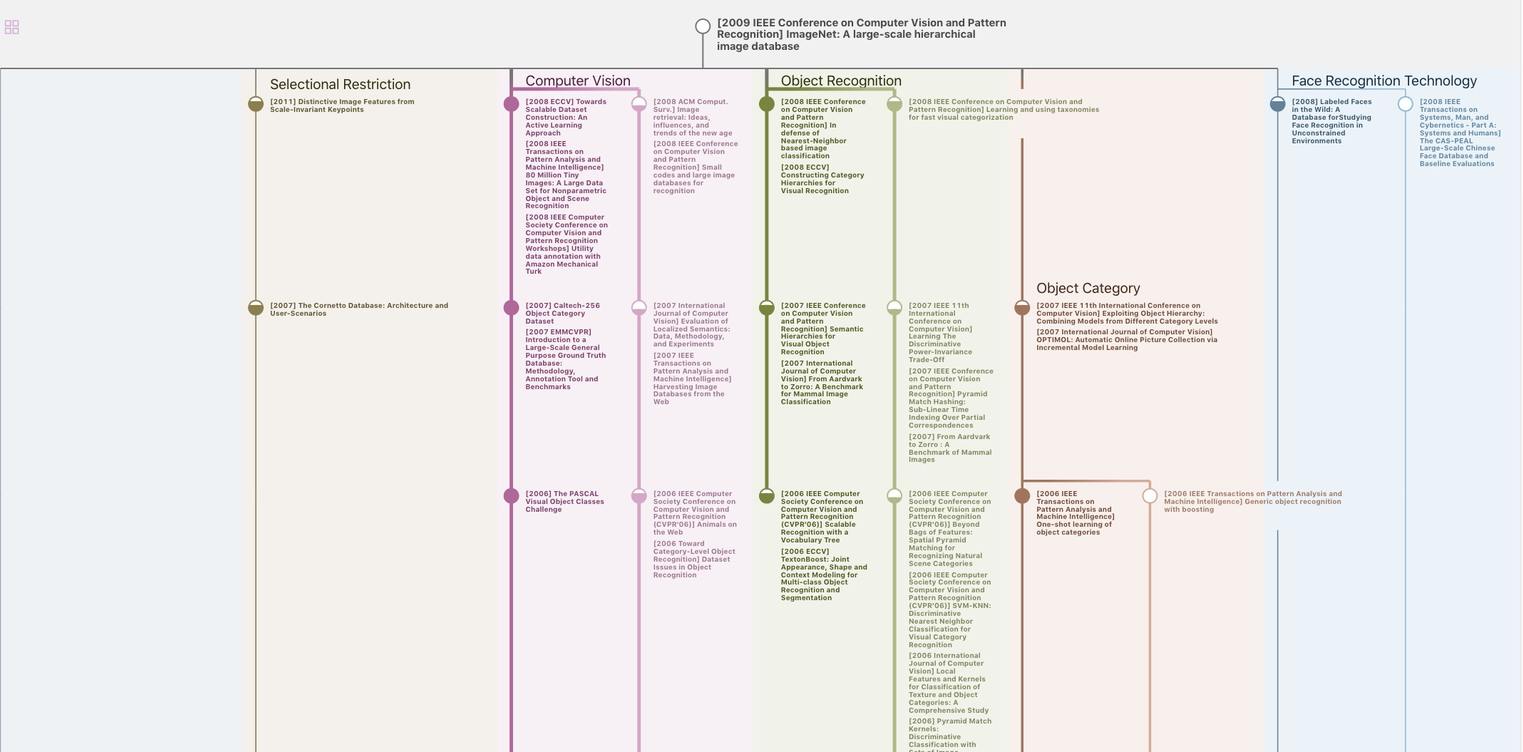
生成溯源树,研究论文发展脉络
Chat Paper
正在生成论文摘要