Bayesian online regression for adaptive direct illumination sampling.
ACM Trans. Graph.(2018)
摘要
Direct illumination calculation is an important component of any physically-based Tenderer with a substantial impact on the overall performance. We present a novel adaptive solution for unbiased Monte Carlo direct illumination sampling, based on online learning of the light selection probability distributions. Our main contribution is a formulation of the learning process as Bayesian regression, based on a new, specifically designed statistical model of direct illumination. The net result is a set of regularization strategies to prevent over-fitting and ensure robustness even in early stages of calculation, when the observed information is sparse. The regression model captures spatial variation of illumination, which enables aggregating statistics over relatively large scene regions and, in turn, ensures a fast learning rate. We make the method scalable by adopting a light clustering strategy from the Lightcuts method, and further reduce variance through the use of control variates. As a main design feature, the resulting algorithm is virtually free of any preprocessing, which enables its use for interactive progressive rendering, while the online learning still enables super-linear convergence.
更多查看译文
关键词
adaptive sampling, direct illumination, learning, visibility
AI 理解论文
溯源树
样例
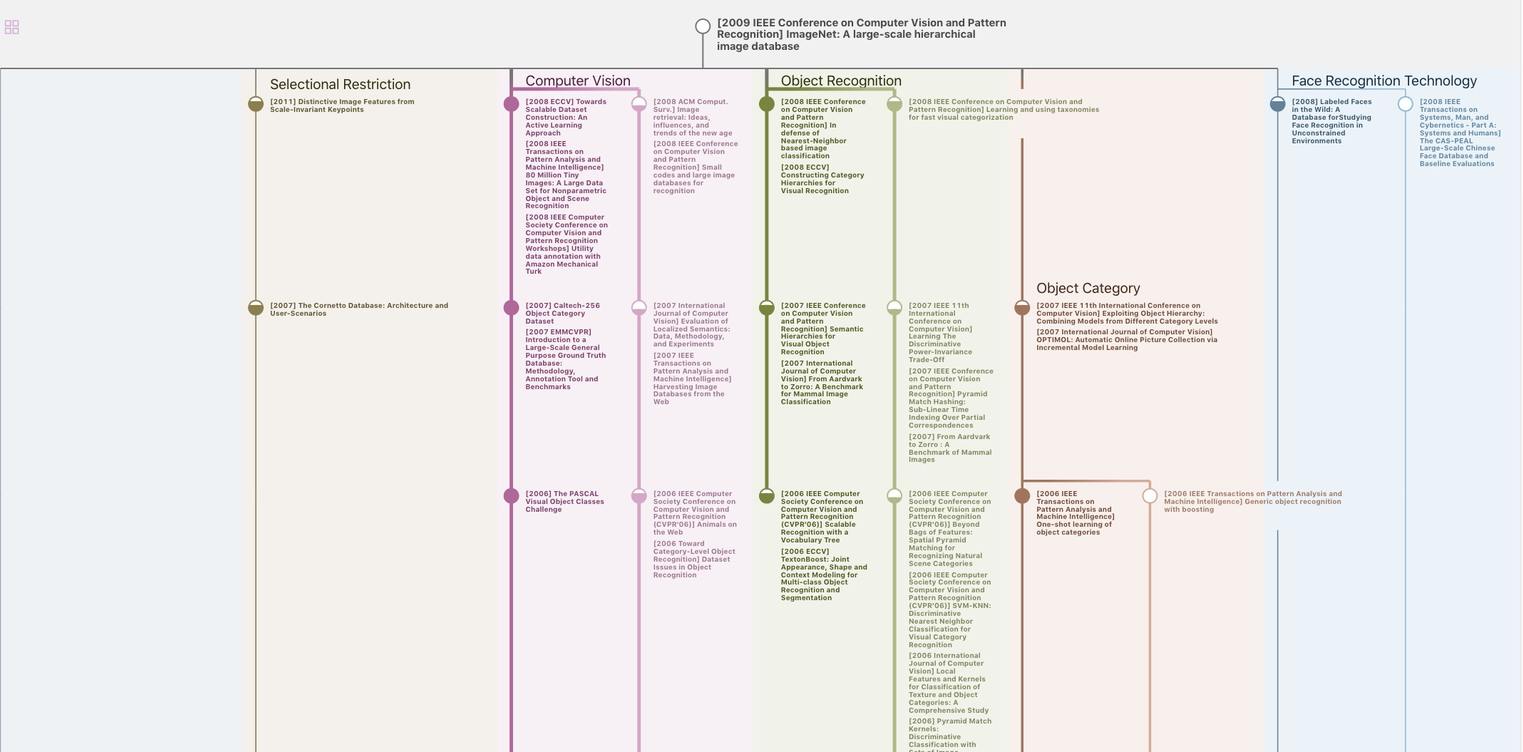
生成溯源树,研究论文发展脉络
Chat Paper
正在生成论文摘要