Malware Analysis Through High-level Behavior.
CSET @ USENIX Security Symposium(2018)
摘要
Malware is becoming more and more stealthy to evade detection and analysis. Stealth techniques often involve code transformation, ranging from equivalent code substitution and junk code injection, to continuously transforming code using a polymorphic or a metamorphic engine. Evasion techniques have a great impact on signature-based malware detection, making it very costly and often unsuccessful. We propose to study a malware's network behavior during its execution. While malware may transform its code to evade analysis, we contend that its behavior must mostly remain the same to achieve the malware's ultimate purpose, such as sending spam, scanning for vulnerable hosts, etc. While live malware analysis is hard, we leverage our Fantasm platform on the Deter-Lab testbed to perform it safely and effectively. Based on observed network traffic we propose a behavior classification approach, which can help us interpret the malware's actions and its ultimate purpose at a high level. We then apply our approach to 999 diverse samples from the Georgia Tech Apiary project to understand current trends in malware behaviors.
更多查看译文
关键词
malware analysis,behavior,high-level
AI 理解论文
溯源树
样例
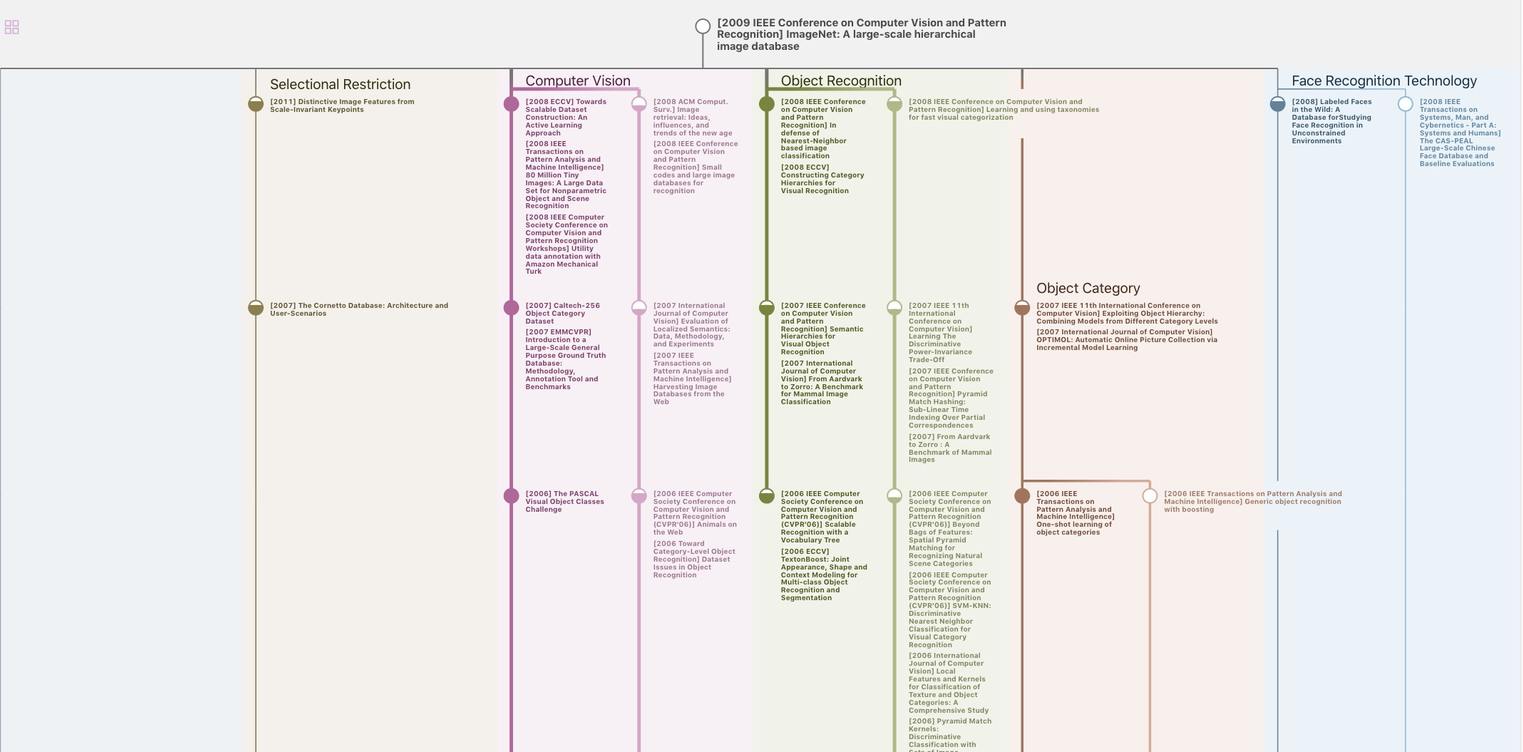
生成溯源树,研究论文发展脉络
Chat Paper
正在生成论文摘要