Design Space Exploration For Orlando Ultra Low-Power Convolutional Neural Network Soc
2018 IEEE 29TH INTERNATIONAL CONFERENCE ON APPLICATION-SPECIFIC SYSTEMS, ARCHITECTURES AND PROCESSORS (ASAP)(2018)
摘要
With the recent advances in machine learning, Deep Convolutional Neural Networks (DCNNs) represent state-of-the-art solutions especially in image and speech recognition and classification. The most important enabler factor of deep learning consists of the massive computing power offered by programmable GPUs for training DCNNs on large amounts of data. Even the complexity of DCNN deployment scenarios, where trained models are used for inference, have started to require powerful computing systems. Especially in the embedded systems domain, the computational requirements along with ultra low-power and memory constraints exacerbate the situation even further. The STM Orlando ultra low-power processor architecture with convolutional neural network acceleration targets exactly this class of problems. Orlando SoC integrates HW-accelerated blocks together with DSPs and on-chip memory resources to enable energy-efficient convolutions for future generations of DCNNs. Although Orlando platform provides flexibility with programmable DSPs, the large variety of DCNN applications make it challenging the design space exploration of the next generations of Orlando architecture. Many hardware design parameters affect the performance and energy-efficiency of the Orlando SoC. Given the huge size of the design space, design space exploration (DSE) and cost-performance tradeoff analysis is needed to select the best set of parameters for the target DCNN application. In this work, we present the exploration results and the tradeoff analysis carried out for the Orlando architecture on the VGG - 16 case study.
更多查看译文
关键词
Convolutional Neural Networks, Ultra Low-Power Embedded Systems, Design Space Exploration
AI 理解论文
溯源树
样例
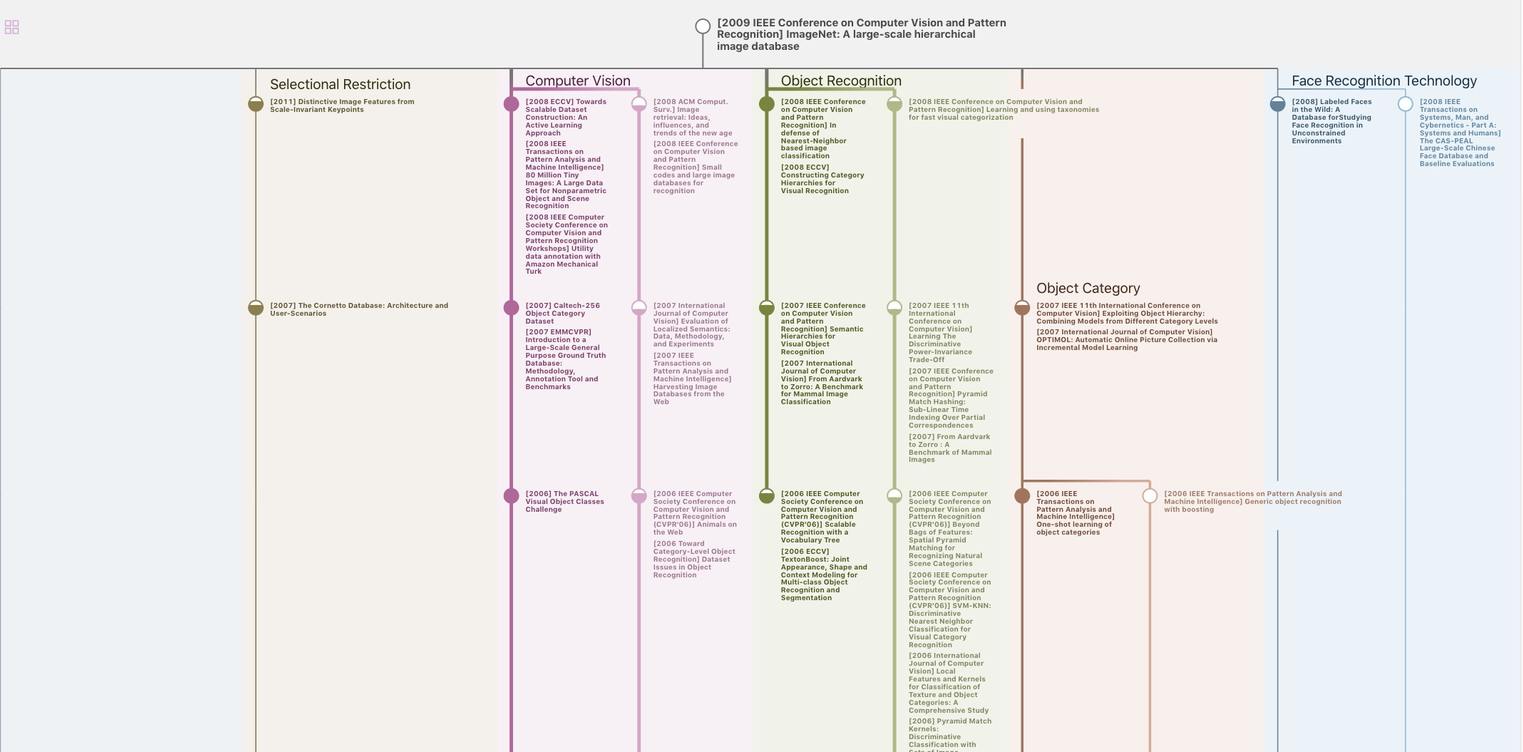
生成溯源树,研究论文发展脉络
Chat Paper
正在生成论文摘要