Extending Detection with Privileged Information via Generalized Distillation
2018 IEEE Security and Privacy Workshops (SPW)(2018)
摘要
Detection systems based on machine learning models are essential tools for system and enterprise defense. These systems construct models of attacks (or non-attacks) from past observations (i.e., features) using a training algorithm. After that, the detection systems use that model for detection at run-time. In this way, the detection system recognizes when the environmental state becomes-at least probabilistically-dangerous. A limitation of this traditional model of detection is that model training is limited to features available at run-time. However, many features are either too expensive to collect in real-time or only available after the fact. In traditional detection, such features are ignored for the purpose of detection. In this paper, we consider an alternative detection model learning approach, generalized distillation, that trains models using privileged information-features available at training time but not at run-time-to improve the accuracy of detection systems. We use a deep neural network to implement generalized distillation for the training of detection models and making predictions. Our empirical study shows that detection with privileged information via generalized distillation increases precision and recall in systems of user face authentication, fast-flux bot detection, and malware classification over systems with no privileged information.
更多查看译文
关键词
privileged information,detection systems,generalized distillation,deep learning
AI 理解论文
溯源树
样例
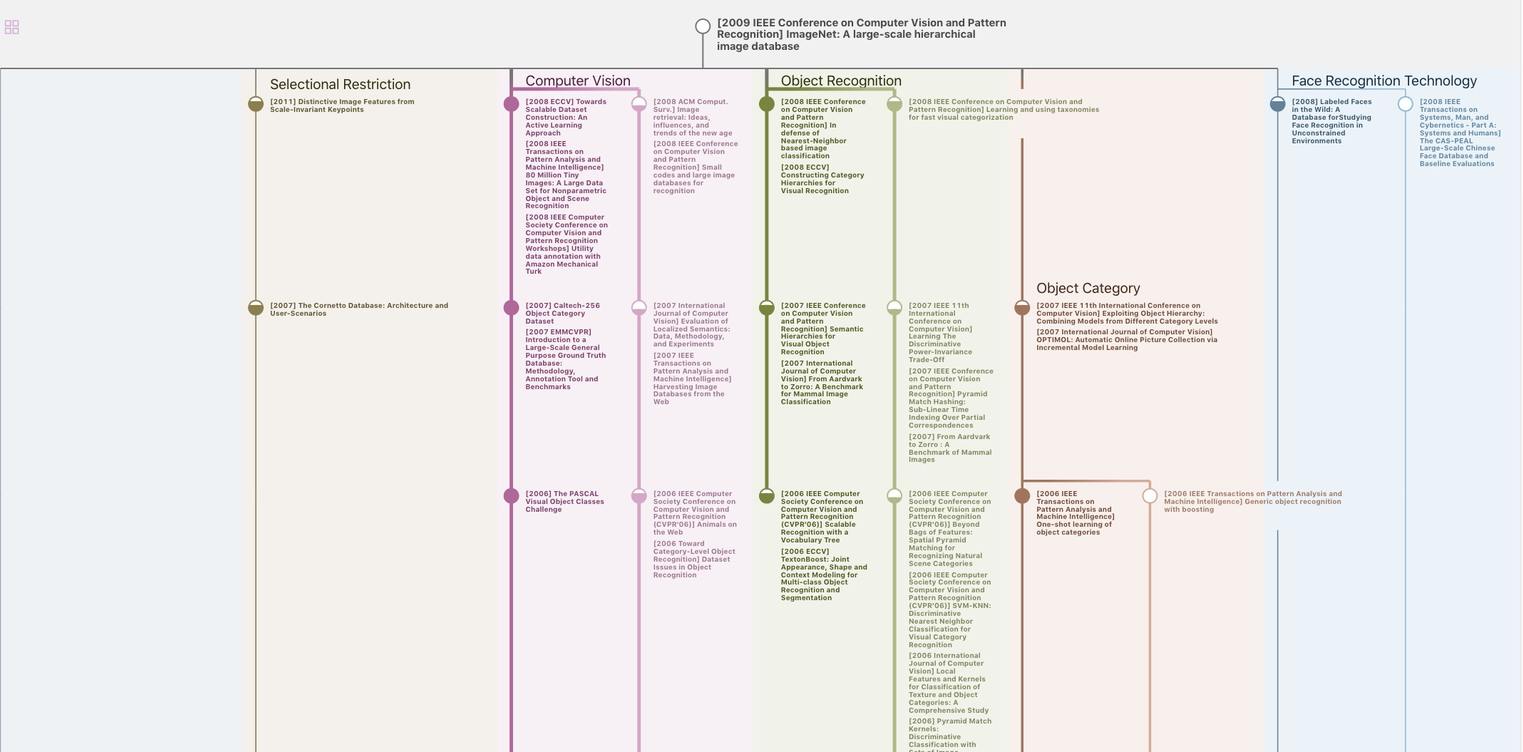
生成溯源树,研究论文发展脉络
Chat Paper
正在生成论文摘要