Remote Production and Mobile Contribution Over 5G Networks: Scenarios, Requirements and Approaches for Broadcast Quality Media Streaming
2018 IEEE International Symposium on Broadband Multimedia Systems and Broadcasting (BMSB)(2018)
摘要
Media applications are amongst the most demanding services requiring high amounts of network capacity as well as extremely low latency for synchronous audio-visual streaming in production quality. Recent technological advances in the 5G domain hold the promise to unlock the potential of the media industry by offering high quality media services through dynamic efficient resource allocation. Actual implementations are now required to validate whether advanced media applications can be realised benefiting from ultra-low latency, very-high bandwidth and flexible dynamic configuration offered by these new 5G networks. A truly integrated approach is needed that focuses on the media applications not only on the management of generic network functions and the orchestration of resources at the various radio, fronthaul/backhaul, edge and core network segments. The H2020 5G PPP Phase 2 project 5G-MEDIA [1] leverages new options for more flexible, ad-hoc and cost-effective production workflows by replacing dedicated lines and hardware equipment with software functions (VNFs) facilitating (semi-) automated smart production in remote locations. Highly scalable virtualized media services deployed on or close to the edge reduce complexity for the user, ensure operational reliability and increase the Quality of Experience (QoE). Virtual compression engines have the potential to replace dedicated encoder/decoder hardware while the network optimisation (Cognitive Network Optimizer) in combination with the Quality of Service (QoS) monitoring helps to overcome the current internet best-effort principle and ensures that the required performance needs are met at all times.
更多查看译文
关键词
Remote production,smart production,mobile contribution,broadcasting,SDN,NFV,edge computing,NFVI,VNF,FaaS
AI 理解论文
溯源树
样例
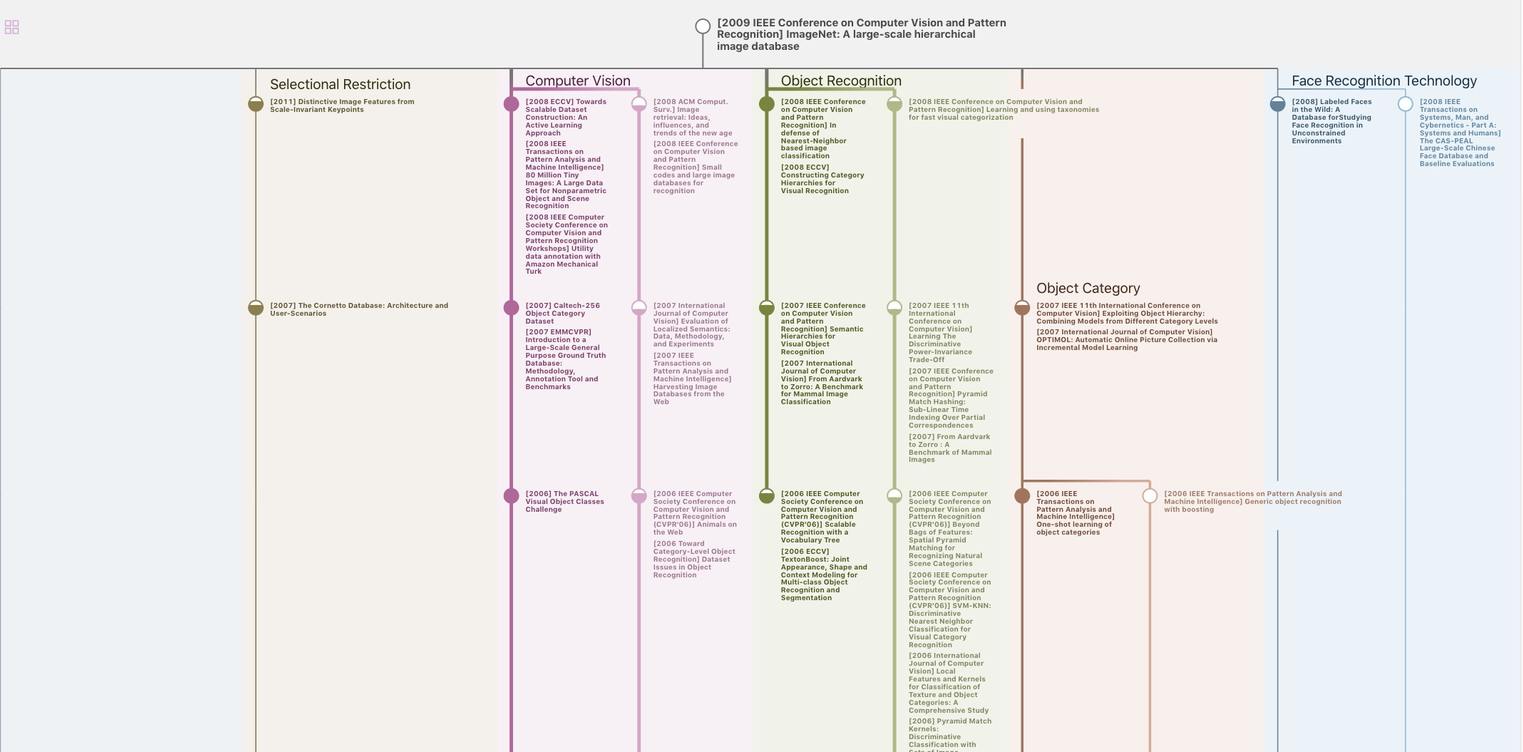
生成溯源树,研究论文发展脉络
Chat Paper
正在生成论文摘要