A Machine-Learning Item Recommendation System for Video Games
2018 IEEE Conference on Computational Intelligence and Games (CIG)(2018)
摘要
Video-game players generate huge amounts of data, as everything they do within a game is recorded. In particular, among all the stored actions and behaviors, there is information on the in-game purchases of virtual products. Such information is of critical importance in modern free-to-play titles, where gamers can select or buy a profusion of items during the game in order to progress and fully enjoy their experience. To try to maximize these kind of purchases, one can use a recommendation system so as to present players with items that might be interesting for them. Such systems can better achieve their goal by employing machine learning algorithms that are able to predict the rating of an item or product by a particular user. In this paper we evaluate and compare two of these algorithms, an ensemble-based model (extremely randomized trees) and a deep neural network, both of which are promising candidates for operational video-game recommender engines. Item recommenders can help developers improve the game. But, more importantly, it should be possible to integrate them into the game, so that users automatically get personalized recommendations while playing. The presented models are not only able to meet this challenge, providing accurate predictions of the items that a particular player will find attractive, but also sufficiently fast and robust to be used in operational settings.
更多查看译文
关键词
recommender systems,ensemble methods,deep learning,online games,user behavior
AI 理解论文
溯源树
样例
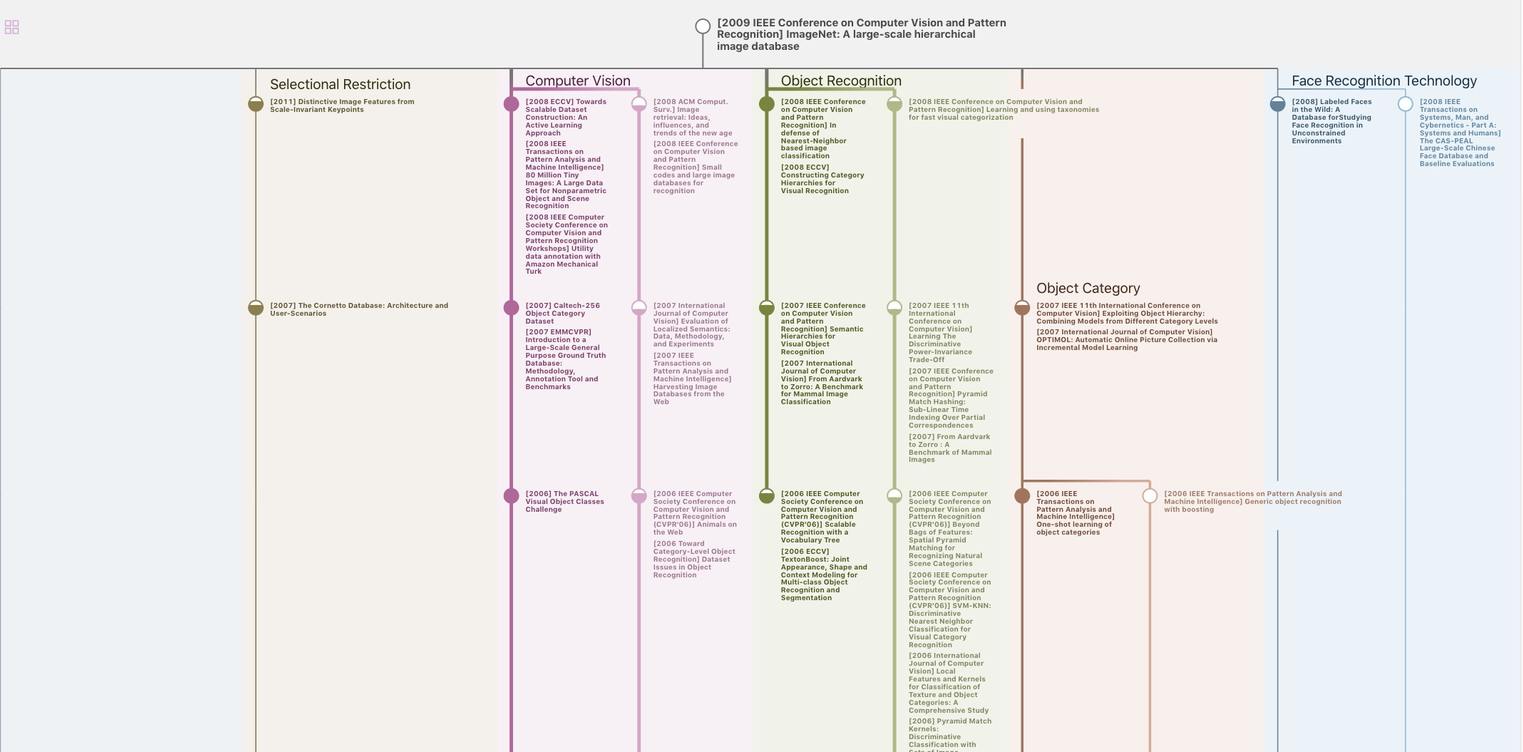
生成溯源树,研究论文发展脉络
Chat Paper
正在生成论文摘要