Accel: A Corrective Fusion Network for Efficient Semantic Segmentation on Video
2019 IEEE/CVF CONFERENCE ON COMPUTER VISION AND PATTERN RECOGNITION (CVPR 2019)(2019)
摘要
We present Accel, a novel semantic video segmentation system that achieves high accuracy at low inference cost by combining the predictions of two network branches: (1) a reference branch that extracts high-detail features on a reference keyframe, and warps these features forward using frame-to-frame optical flow estimates, and (2) an update branch that computes features of adjustable quality on the current frame, performing a temporal update at each video frame. The modularity of the update branch, where feature subnetworks of varying layer depth can be inserted (e.g. ResNet-18 to ResNet-101), enables operation over a new, state-of-the-art accuracy-throughput trade-off spectrum. Over this curve, Accel models achieve both higher accuracy and faster inference times than the closest comparable single-frame segmentation networks. In general, Accel significantly outperforms previous work on efficient semantic video segmentation, correcting warping-related error that compounds on datasets with complex dynamics. Accel is end-to-end trainable and highly modular: the reference network, the optical flow network, and the update network can each be selected independently, depending on application requirements, and then jointly fine-tuned. The result is a robust, general system for fast, high-accuracy semantic segmentation on video.
更多查看译文
关键词
Segmentation,Grouping and Shape,Scene Analysis and Understanding
AI 理解论文
溯源树
样例
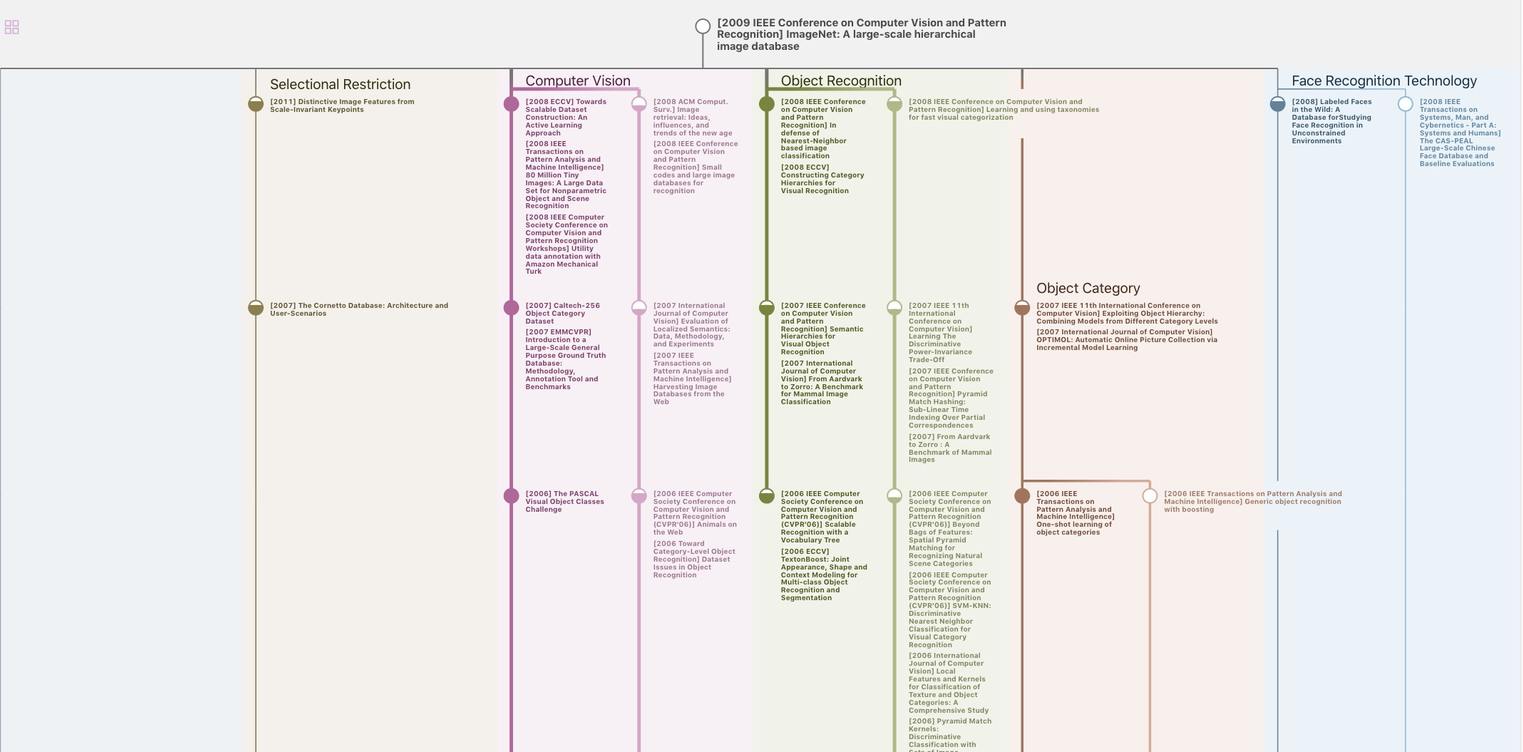
生成溯源树,研究论文发展脉络
Chat Paper
正在生成论文摘要