Identification of Genetic Causality Statements in Medline Abstracts Leveraging Distant Supervision
2018 IEEE International Conference on Healthcare Informatics Workshop (ICHI-W)(2018)
摘要
In the era of precision medicine, the clinical utility of next generation sequencing technology highly depends on the ability of interpreting the causality association of genetic variants and phenotyping which can be a labor intensive process. There are various resources available for cataloging such associations such as HGMD or ClinVar. Given the exponential growth in literature in the field, it is desired to accelerate the process by automatically identifying genetic causality statements from literature. Here, we define the task of identifying the statements as a classification task for sentences containing gene and disease entities. We used the cancer gene census available at the Catalogue of Somatic Mutations in Cancer (COSMIC) and to generate a weakly labeled data set for our classification task. We evaluated multiple feature sets such as: words, bi-grams, word embedding, and several machine-learning methods and showed the weighted F-measure around 95%. Evaluation using the top 50 genetic variant disease sentences demonstrated that the proposed method can identify genetic causality statements.
更多查看译文
关键词
cancer,disease,causality,genetic variant,distance supervision,classification,Semantic Medline,MutD,ClinVar
AI 理解论文
溯源树
样例
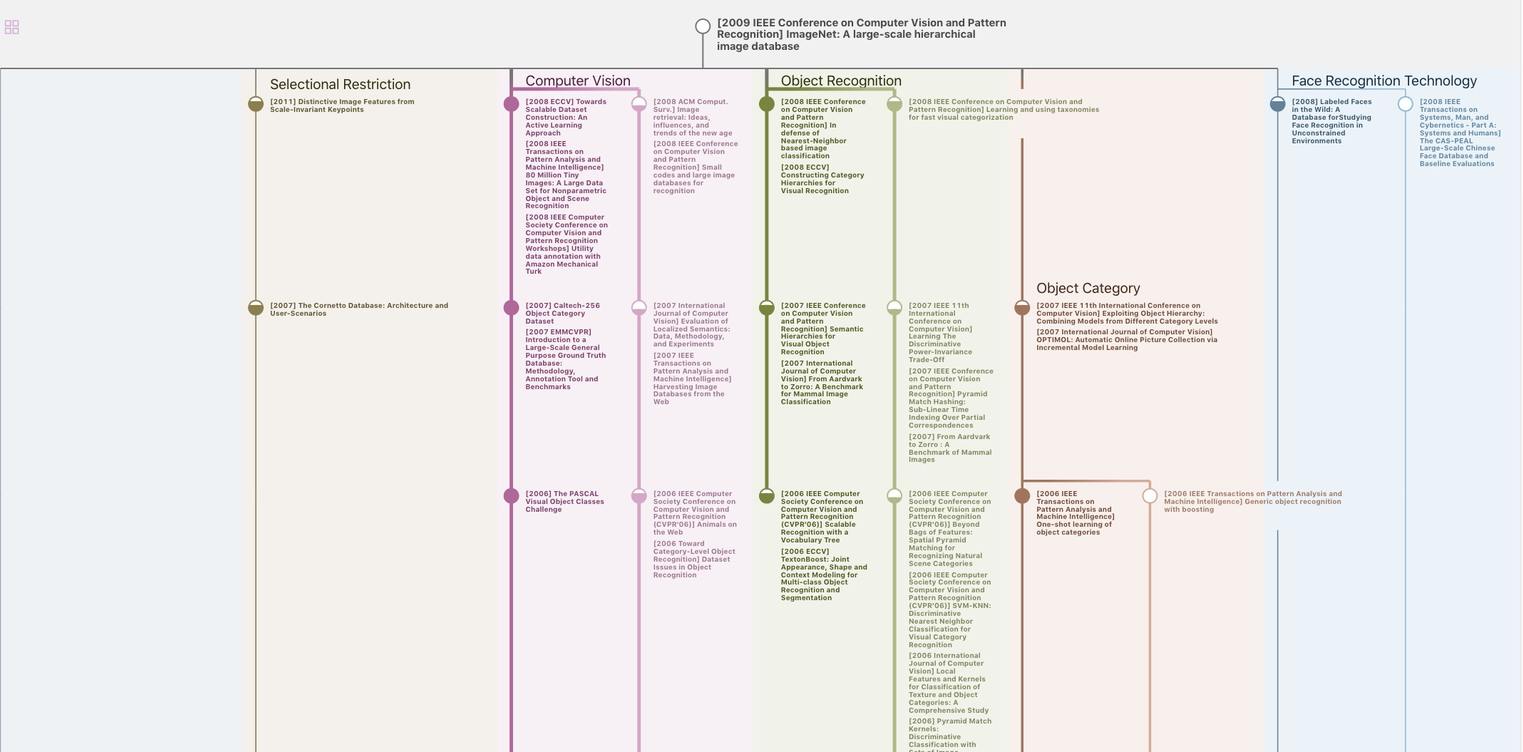
生成溯源树,研究论文发展脉络
Chat Paper
正在生成论文摘要