CA-LSTM: Search Task Identification with Context Attention based LSTM.
SIGIR(2018)
摘要
Search task identification aims to understand a user's information needs to improve search quality for applications such as query suggestion, personalized search, and advertisement retrieval. To properly identify the search task within long query sessions, it is important to partition these sessions into segments before further processing. In this paper, we present the first search session segmentation model that uses a long short-term memory (LSTM) network with an attention mechanism. This model considers sequential context knowledge, including temporal information, word and character, and essentially learns which parts of the input query sequence are relevant to the current segmentation task and determines the influence of these parts. This segmentation technique is also combined with an efficient clustering method using a novel query relevance metric for end-to-end search task identification. Using real-world datasets, we demonstrate that our segmentation technique improves task identification accuracy of existing clustering-based algorithms.
更多查看译文
关键词
Search Session Identification,Long Short-Term Memory Recurrent Neural Network,Attention Mechanism
AI 理解论文
溯源树
样例
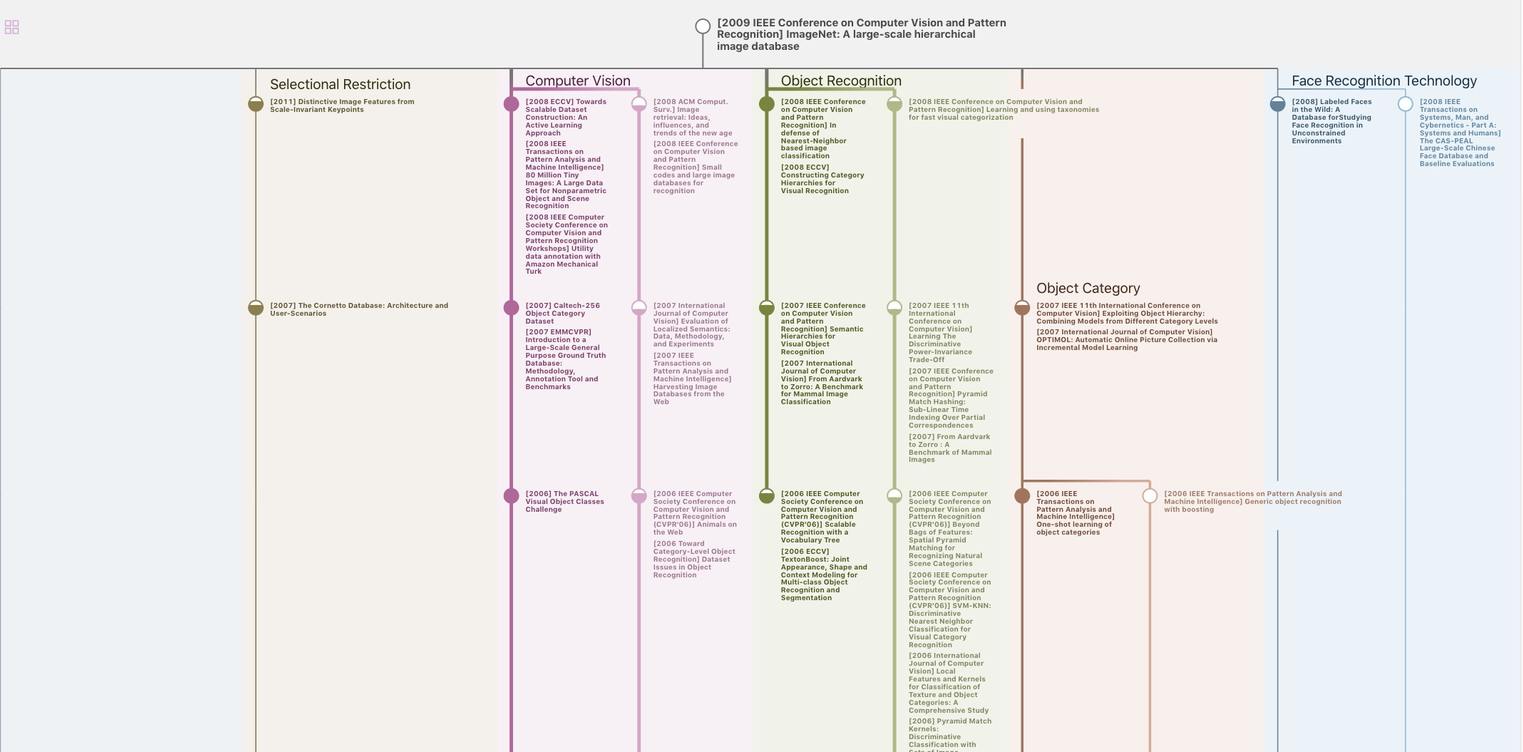
生成溯源树,研究论文发展脉络
Chat Paper
正在生成论文摘要