Deep Learning for Epidemiological Predictions.
SIGIR(2018)
摘要
Predicting new and urgent trends in epidemiological data is an important problem for public health, and has attracted increasing attention in the data mining and machine learning communities. The temporal nature of epidemiology data and the need for real-time prediction by the system makes the problem residing in the category of time-series forecasting or prediction. While traditional autoregressive (AR) methods and Gaussian Process Regression (GPR) have been actively studied for solving this problem, deep learning techniques have not been explored in this domain. In this paper, we develop a deep learning framework, for the first time, to predict epidemiology profiles in the time-series perspective. We adopt Recurrent Neural Networks (RNNs) to capture the long-term correlation in the data and Convolutional Neural Networks (CNNs) to fuse information from data of different sources. A residual structure is also applied to prevent overfitting issues in the training process. We compared our model with the most widely used AR models on USA and Japan datasets. Our approach provides consistently better results than these baseline methods.
更多查看译文
关键词
deep learning,epidemiology prediction,time series
AI 理解论文
溯源树
样例
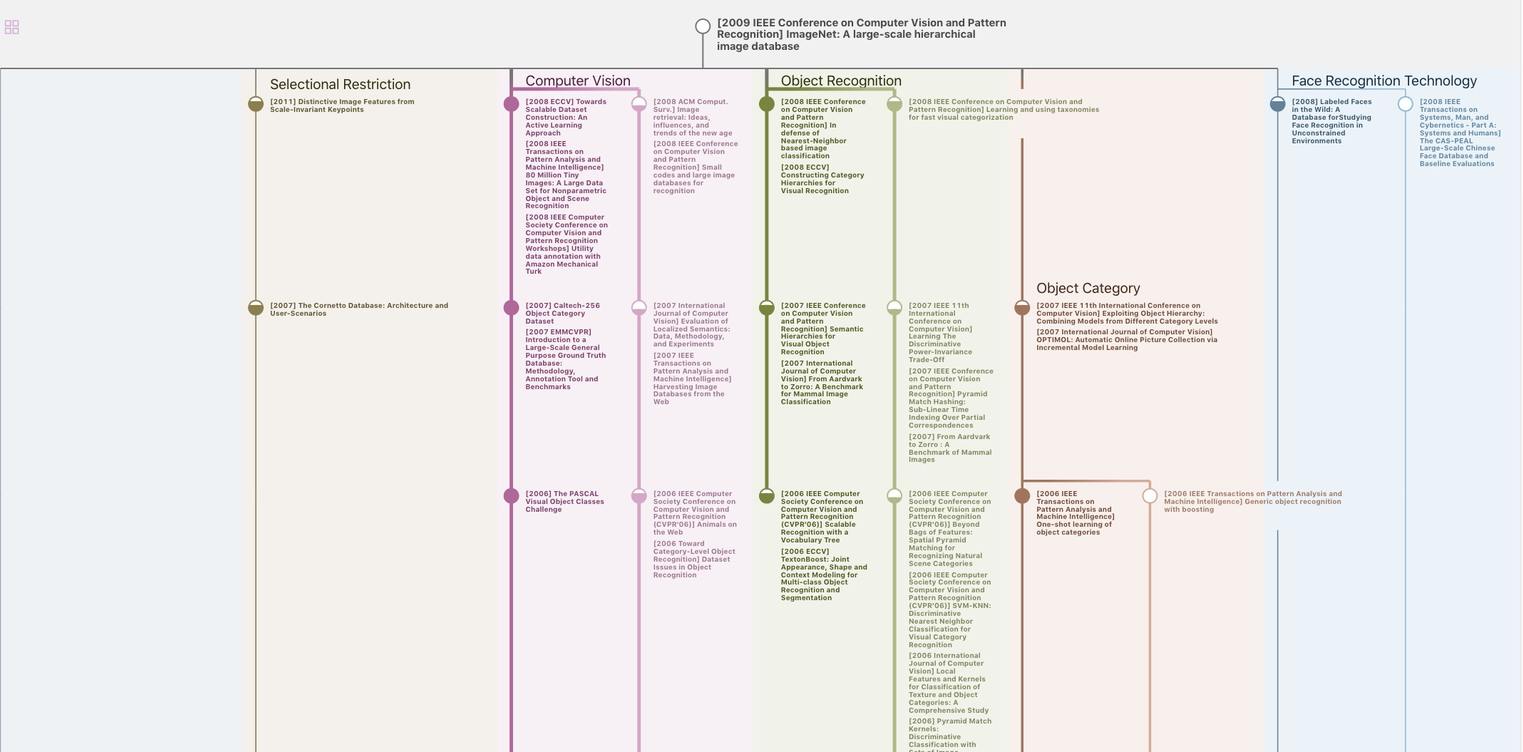
生成溯源树,研究论文发展脉络
Chat Paper
正在生成论文摘要