The Evolution of Content Analysis for Personalized Recommendations at Twitter.
SIGIR(2018)
摘要
We present a broad overview of personalized content recommendations at Twitter, discussing how our approach has evolved over the years, represented by several generations of systems. Historically, content analysis of Tweets has not been a priority, and instead engineering efforts have focused on graph-based recommendation techniques that exploit structural properties of the follow graph and engagement signals from users. These represent "low hanging fruits" that have enabled high-quality recommendations using simple algorithms. As deployed systems have grown in maturity and our understanding of the problem space has become more refined, we have begun to look for other opportunities to further improve recommendation quality. We overview recent investments in content analysis, particularly named-entity recognition techniques built around recurrent neural networks, and discuss how they integrate with existing graph-based capabilities to open up the design space of content recommendation algorithms.
更多查看译文
AI 理解论文
溯源树
样例
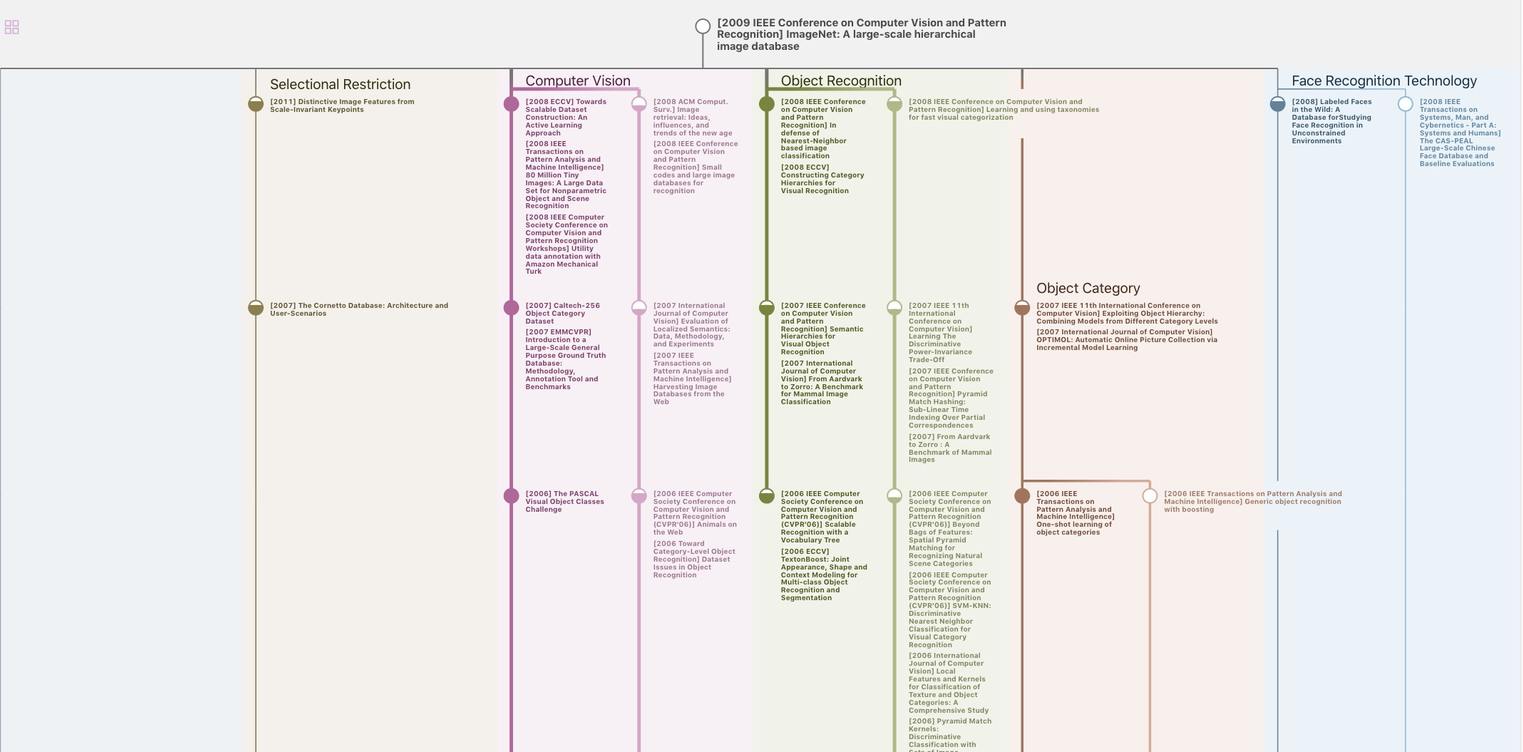
生成溯源树,研究论文发展脉络
Chat Paper
正在生成论文摘要