Better Textbooks with Human Language Technology.
SIGIR(2018)
摘要
Almost every part of the world relies on textbooks as the primary medium of imparting education. The quality of education, thus, is correlated with the quality of textbooks. In general, the quality of content in the textbooks used in the less-developed countries of the world is not up to the mark [1]. In addition to their intended purpose of delivering information, textbooks also promote behaviours that adults wish to pass on to the next generation [7]. It is, thus, important to ensure that textbooks are helpful in effective learning and do not condone undesirable social mores. The task of evaluating textbooks against these parameters is not trivial: experts must go through the entire content manually. This exercise being not only laborious, but also expensive [3]. This thesis attempts to propose a feasible computational alternative to this process
The dataset that we are working with is comprised of school textbooks collected from different boards of education of two south-east Asian countries that are widely regarded as 'developing countries'[4]. In general, a digitized textbook throws a large number of computational problems that require ideas from a number of disciplines such as Natural Language Processing, Information Retrieval, Human Computer Interaction and Cognitive Science. In this thesis we focus on the following research questions.
Research Question 1 How can we automatically identify the text fragments that reflect gender bias from textbooks?
The prevalence of gender bias has been reported in textbooks from many parts of the world [5,6]. Computational efforts to contain the effects of such biases have been proposed [2], but the detection of presence of gender bias, and identifying text passages that exhibit such biases is an unexplored problem. We model the task as a binary classification problem, with one class being biased text, and the other being unbiased text. For classifying text fragments, we propose a boosted memory-based model to learn the hidden patterns of biases from a small amount of labelled data. We are currently working on the sub-problem of identifying the gender of named human entities in textbooks in the absence of clear linguistic markers of gender such as 'sister', 'himself', etc. Our methodology is based on leveraging contextual data in the form of phrase vectors, along with sentence structure.
Research Question 2 How can we enhance the learning experience of a student through an optimal ordering of concepts, and ensure the coverage of all necessary topics?
We propose to represent textbook sections in the form of concept graphs [8], and utilize the linked structure of Wikipedia to determine necessary and sufficient concepts whose inclusion ensures completeness of the graph, i.e., prerequisite concepts [3] are not left out, while minimizing redundancy. For example, on the event of the presence of outgoing edges from an excluded concept C 1 to a concept C 2 included in the textbook, we suggest that C 1 be also included to ensure a comprehensive coverage of the subject matter. We also propose assigning weights to these edges, denoting the amount of dependence a concept has on another, to facilitate our aim of finding an optimal ordering of the concepts.
Research Question 3 How can we automatically identify and accumulate resources of the same difficulty level as the original text from external repositories, to facilitate betterunderstanding of the content among students?
Students often refer to online resources to better understand textbook content. A major hurdle associated with this practice is the wide mismatch in difficulty levels of the textbook, and the hits returned by a search engine [1]. Our aim is to learn to distinguish between texts of different levels of difficulty by using textbooks as the training data. We propose to apply a transfer learning-based approach to use the parameters learned during training to identify suitable web content. At present, we are planning to work with school-level basic science textbooks.
更多查看译文
关键词
Textbooks,Human Language Technology,Web resource mining
AI 理解论文
溯源树
样例
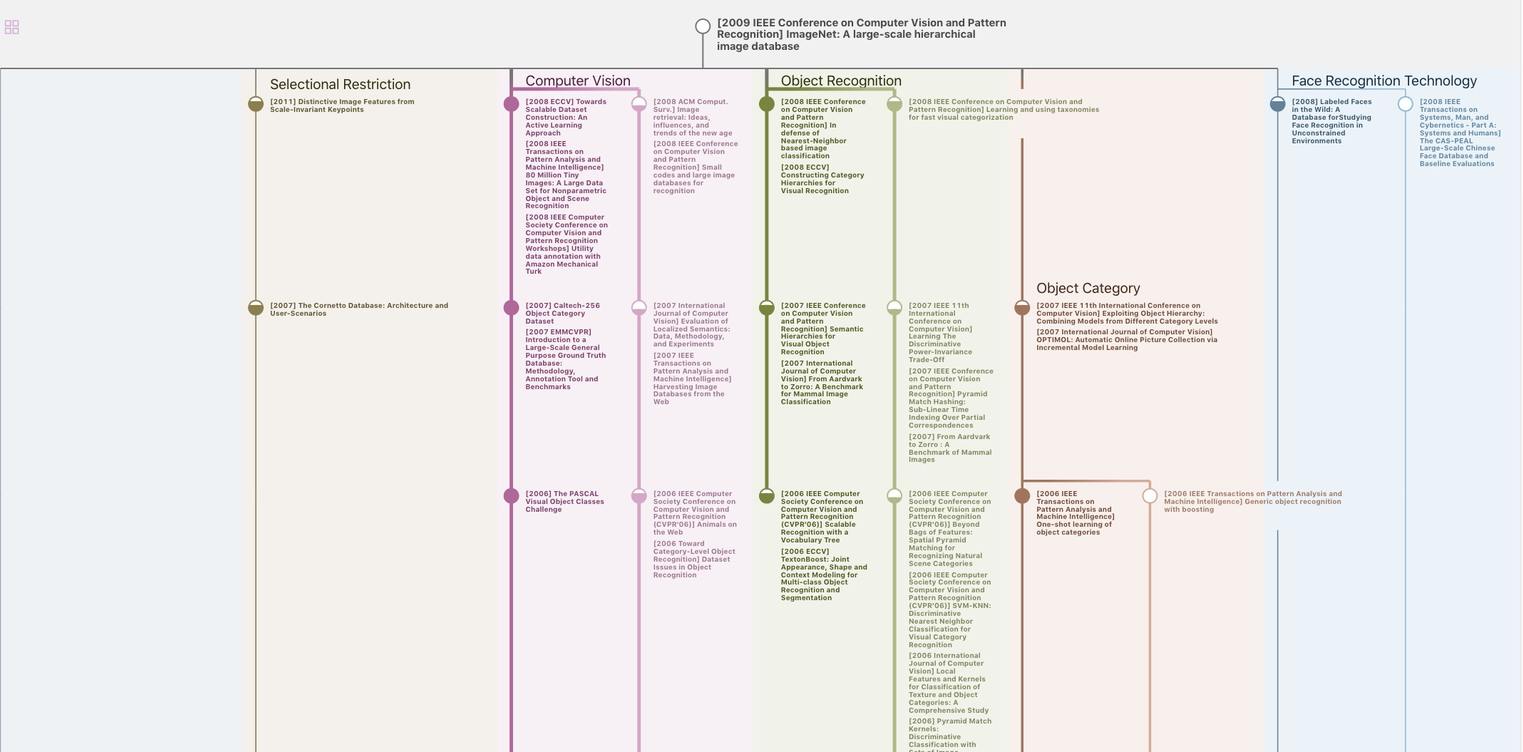
生成溯源树,研究论文发展脉络
Chat Paper
正在生成论文摘要