Comparing Visual, Textual, and Multimodal Features for Detecting Sign Language in Video Sharing Sites
2018 IEEE Conference on Multimedia Information Processing and Retrieval (MIPR)(2018)
摘要
Easy recording and sharing of video content has led to the creation and distribution of increasing quantities of sign language (SL) content. Current capabilities make locating SL videos on a desired topic dependent on the existence and correctness of metadata indicating both the language and topic of the video. Automated techniques to detect sign language content can aid this problem. This paper compares metadata-based classifiers and multimodal classifiers, using both early and late fusion techniques, with video content-based classifiers in the literature. Comparisons of applying TF-IDF, LDA, and NMF in the generation of metadata features indicates that NMF performs best, either when used independently or when combined with video features. Multimodal classifiers perform better than unimodal SL video classifiers. Experiments show multimodal features obtained results of up to 86% precision, 81% recall, and 84% F1 score. This represents an improvement on F1 score of roughly 9% in comparison with the video-based approach presented in the literature and an improvement of 6% over text-based features extracted using NMF.
更多查看译文
关键词
Sign language detection,multimodal classification,machine learning
AI 理解论文
溯源树
样例
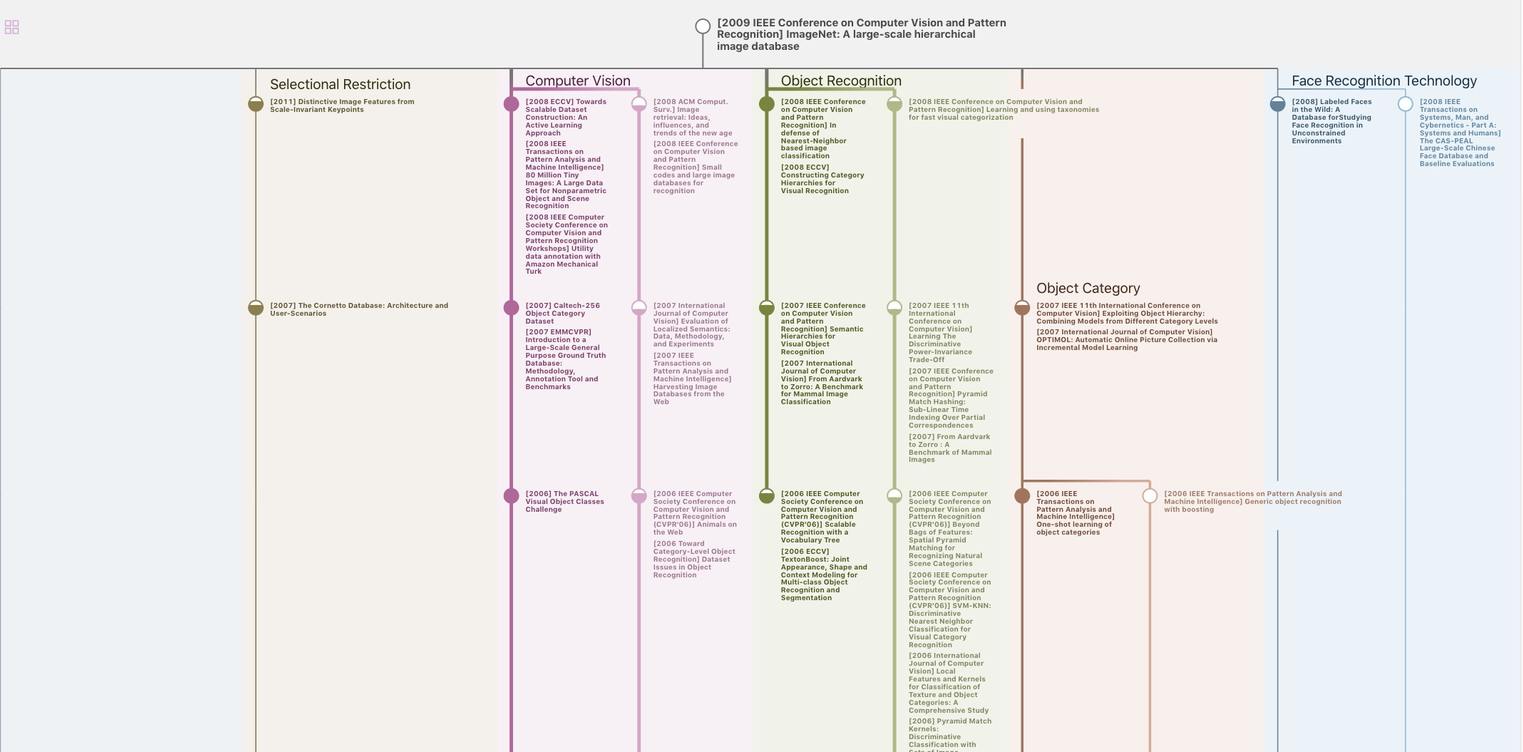
生成溯源树,研究论文发展脉络
Chat Paper
正在生成论文摘要